Discover how Oak Ridge National Laboratory scientists have harnessed the power of deep learning to revolutionize the analysis of plasma plumes, paving the way for advanced materials and cutting-edge technologies.
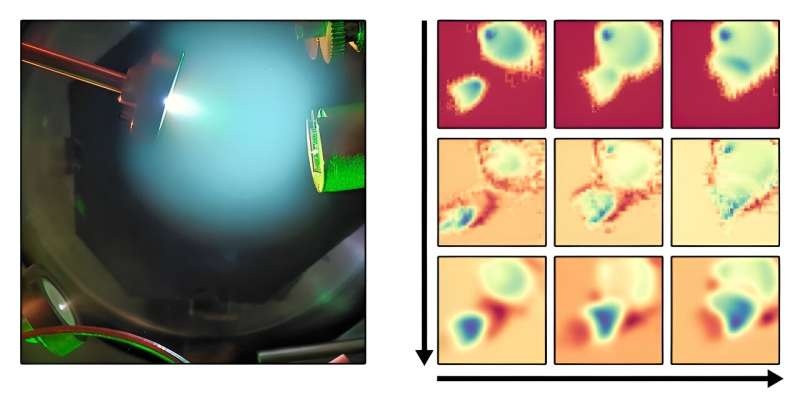
Automating Quality Control
Pulsed laser deposition (PLD) is a key way to develop new materials for electronics and energy technologies in the field of materials science. In this technique, a target material is vaporized using intense laser pulses to produce a plasma plume i.e. an atom and particle stream resembling cloud.
In the past, expert scientists have used their judgment to judge color, shape, size and brightness of this plasma-related plume for the thin films produced. Unfortunately, this manual process can be quite time-intensive and is subject to a great deal of human error.
The groundbreaking work of Oak Ridge National Laboratory scientists. In their project, they created a deep learning model to accomplish this mapping autonomously. This AI-enabled system can now analyze high-speed videos of plasma plumes in a fraction of the time it takes expert scientists to make the same assessment — and more accurately.
Their ability to train the AI on what constitutes an ideal sample has not only shortened the quality control process, but it has also now given us fresh new insights into how these particles are behaving during film formation on a microscopic scale. This advance has the potential to revolutionize monitoring of materials synthesis and speed up development, and it significantly differs from the traditional approaches using mass spectrometry.
Speeding Up the Tools of Science
This system, which analyzes plasma plumes using AI, develops on an autonomous PLD system that ORNL previously pioneered. These systems have already proven that they can be ten times faster in finding new materials — a major undertaking with the potential to change the way materials science is done.
The alignment of PL imagery with AI for plume feature identification provides a powerful platform to automate the identification and analysis of these films in a speedy manner, thus to greatly facilitate further materials research. Such a synergetic manner not only improves the control of quality, but also offers a new perspective for understanding the complicated dynamics of plasma plume in film deposition.
Picture a day when materials scientists could quickly iterate on new material compositions, with the AI system watching over the process every step of the way, offering real-time suggestions. Such a high level of automation and optimization can reduce the time and resources needed to create these next-generation materials that will be used in electronics, energy technologies, etc.
This work has implications that go way beyond the lab. With such a growing need for the next big ideas, let us hope that designing and synthesising materials is quicker. With AI, we are entering a new space in materials science where the only limits will be set by our imagination.
Conclusion
Deep learning with plasma plume analysis would be quite a significant advancement for material scientists. This new development, which automates quality control and provides insight into the behavior of minuscule particles, would revolutionize the manufacturing process of advanced materials. Providing the potential to speed up materials discovery by a factor of 10, this technology could create a future in which innovative new materials are being rapidly and efficiently developed to fuel the next wave of technological breakthroughs.