Discover how machine learning is paving the way for more efficient and sustainable Maglev transportation systems, potentially ushering in a new era of eco-friendly travel.
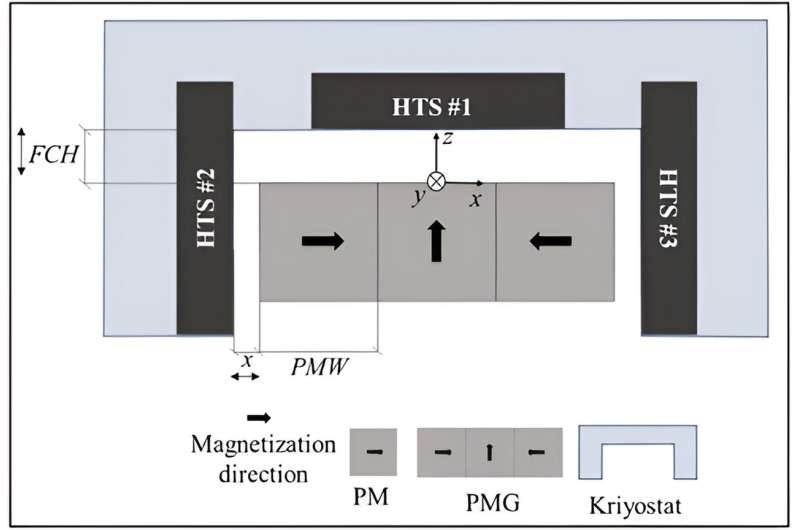
Harnessing the Power of AI
The transport sector has been one of the most significant contributors to global carbon emissions for centuries, but this is about to change. For this reason, researchers are working in an active way to look for how transport systems and technologies play a role in the sustainability and environmentally friendly field.
One of the most promising technologies that I want to highlight in this answer is magnetic levitation (Maglev) which uses strong magnets to quickly push a train forward while it floats just above the rail. It is this lack of friction from the track that allows Maglev trains to accelerate to such astounding speeds with very little energy used.
Unfortunately though, measuring the forces generated by maglev systems has proven to be tricky at best, turning into a major bottleneck in the viability of these concepts for practical use. Like many things in finance, this problem is the ideal use case for artificial intelligence (AI) and machine learning. Thanks to the efforts of a team headed by Erkan Caner Ozkat at Recep Tayyip Erdogan University in Türkiye, who have shown how an AI can be used to refine these force measurements through an innovative new technique following their latest round of research, Maglev systems could soon be one step closer to becoming commonplace.
Absorbing Maglev complexities
Whereas the Maglev uses high-temperature superconductors in which magnets can carry a current with no electrical resistance at ambient conditions you would encounter outdoors. Two major forces could substantially dictate the performance of the trains: levitation forces, which raise the train off their tracks and reduce friction with the track below, and lateral forces, applied at right angles to tracks that sway the train back and forth.
To increase the levitation and lateral guidance force of Maglev systems, which have a direct impact on load capacity and operational safety, respectively. Their earlier work on the speeds along multi-surface interactions of superconductors with the permanent magnetic track needed a mechanical set-up for measurement [3].
That is where the innovation of the team appears. Using a machine learning model, they successfully predicted the levitation and lateral forces which was impossible to show directly from traditional experimental approaches. The discovery has wonderful implications for the efficiency and operating reliability of future Maglev systems around the world, essentially reducing the carbon footprint in global transport.
Conclusion
Ozkat’s team is leading to upgrade AI to completely change traditional Maglev information, as shown in its research. With this new technology enabling more precise force measurement and system parameter optimizations, we may soon see an influx of Maglev systems that would ultimately allow us to move one step closer to a greener future in transportation. For a globe at the mercy of catastrophic climate change, transformations such as this offer hope for a cleaner, better, and more ubiquitous transport future for all.