Explore how a groundbreaking AI tool, PertKGE, is transforming the world of drug discovery by efficiently deconvoluting the complex interactions between compounds and proteins, paving the way for accelerated drug development.
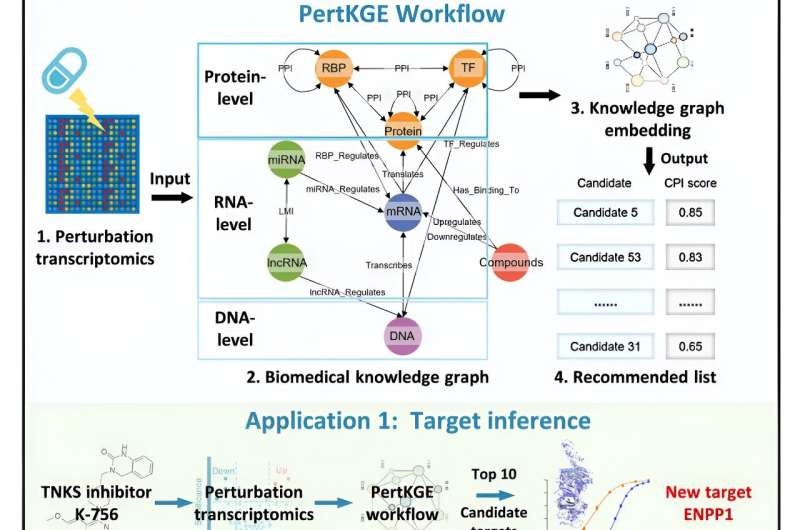
Cracking the Code of Compound-Protein Binding
In the pharmacological world identifying and understand how compounds interact with proteins is very crucial. Compounds and proteins are the basic components but what really matters is how these compounds and proteins interact, this is where ultimate answers lie for creating new effective targeted treatments.
Historically, predicting and evaluating compound-protein interactions (CPI) has been an area of great concern and despite many breakthroughs over the past decades there is still no silver bullet approach to this computational task. Nevertheless, recent breakthroughs in high-throughput transcriptomic analysis enable new possibilities for drug detection.
Perturbation transcriptomics, that captures the cellular transcriptomic responses to multiple compounds, offer a renewed perspective for CPI decoupling. As the compound-associated features contrast groups with single cells, cell lines or even patients, by linking these features to omics data a direct link is provided between responses of various key entities (compounds) and their subjects.
PertKGE: AI for CPI Deconvolution
Good thing, too, because it has a majorly cool name: PertKGE—a tool created by researchers Zheng Mingyue of the Shanghai Institute of Materia Medica (SIMM) of the Chinese Academy of Sciences and his colleagues Zhang Sulin and Li Xutong. This novel instrument uses knowledge graph embedding to disentangle CPI from perturbation transcriptomics.
A the crux of pertKGE is this way to build an interpretable knowledge graph. Contrary to standard techniques, PertKGE decomposes genes into: DNA, messenger RNA (mRNA), long noncoding RNA (lncRNA), microRNA (miRNA) and the two main protein regulators of gene transcription and mRNA processing: transcription factors (TFs) and RNA-binding proteins (RBPs). This strategy enables the tool to model the complex network of low-level cooperativity among these different families of gene-related entities, effectively mimicking post-transcriptional and post-translational regulatory events typical in natural systems.
Utilizing the DistMult interpretation of knowledge graph embedding for all these entities, PertKGE will map them into rich semantic hidden space which allows us to identify CPIs efficiently from vast perturbation transcriptomic data.
Conclusion
PertKGE demonstrated its high throughput capacity to predict binding targets for new compounds and perform virtual ligand screening against new targets, offering a promising solution in drug discovery. The tool has led to the discovery of new biological insights (e.g., Ectonucleotide Pyrophosphatase/Phosphodiesterase 1 as a target mediating the selective anti-tumor immunotherapy response of a tankyrase inhibitor, and five novel inhibitors targeting the emerging cancer therapeutic target ALDH1B1), highlighting its potential for pharmacological studies. With pertKGE getting more regulatory events integrated and becoming amenable for other perturbation omics data, the future of drug discovery seems to be promising as this could potentially transform the way we approach developing life-saving therapies.