In a groundbreaking development, researchers at the University of Illinois Urbana-Champaign have discovered a way to simplify and democratize the process of agricultural quality assessment. By leveraging deep machine learning, they have found a method to reconstruct hyperspectral images from standard RGB images, paving the way for a more affordable and accessible approach to product analysis. This innovative technique has the potential to transform the agricultural industry, empowering producers and consumers alike with accurate and cost-effective quality assessment tools.
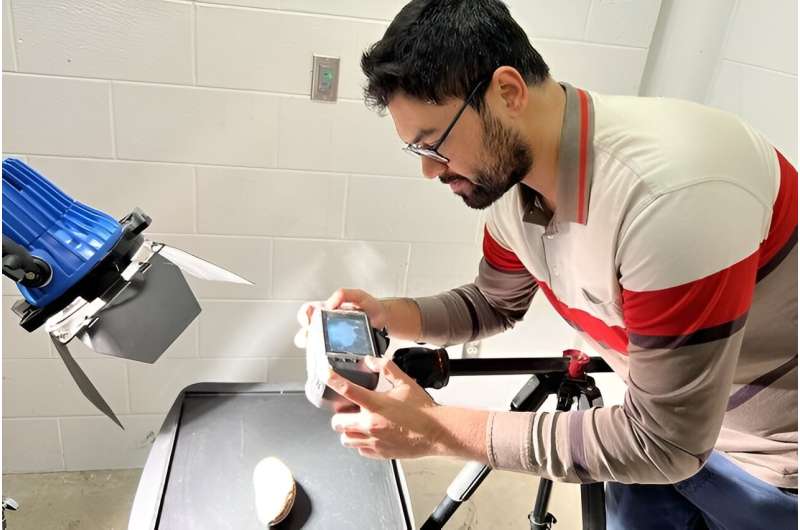
Unleashing the Power of Hyperspectral Imaging
The powerful application of hyperspectral imaging in food and agricultural products for chemical composition analysis has been well recognized for a long time. But widespread adoption has been inhibited due to the high upfront cost and complicated machines necessitated.
However, researchers at the University of Illinois Urbana-Champaign have solved this problem. Using deep learning algorithms, they came up with a technique to convert standard RGB images, which can be obtained from any ordinary camera or smartphone, into Trichromatic OECF hyperspectral images. Thanks to this, it is already possible to determinate some characteristic chemical properties that define the quality consumer attributes such soluble solid content and dry matter important in the evaluation of agricultural products related with taste and nutritional value.
Improving Quality Control Through the Entire Industry
The potential applications of this technology are truly extraordinary. This will allow low-cost, handheld devices to be used to assess the products making it feasible and easily accessible for quality control on a large scale within the agricultural sector.
After conducting initial research on sweet potatoes, the team is confident in their work thus far: their deep learning models have successfully predicted soluble solid content over 70% of the time and dry matter content over 88% of the time. The results are a vast improvement upon those reported in prior studies and are indicative of the promise with which this technology holds toward revolutionizing how agricultural products are assessed.
Farther beyond are other applications in the egg and hatchery industry, where hyperspectral images were reconstructed to predict chick embryo mortality using their deep learning methods. This versatility underscores how broadly applicable their method would be, truly revolutionizing the world of agricultural quality control.
A Greater, more Economical Potential
The world is catching news waves of recipes and as global population continues to increase the importance of sustainable, high-quality products becomes relevant more than ever. One of the key ways to address it is ensuring that we are able to locally assess product quality with high precision, cost-effectively and accessibly.
The efforts of the University of Illinois Urbana-Champaign researchers are an important step in that direction. Their method is game-changing right, as it has democratized the analysis by making it the easy yet cost-effective — something that may alter #agricultural quality control for good.
There are still problems to overcome in making this technology useful on an industrial scale, but the results from the team have opened a new chapter in their use. But as they refine and expand that work, the vision of a future in which quality assessment is both affordable and accurate looms within reach. It is a development with the incredibly useful potential to revolutionize agriculture for the benefit of both producers and consumers everywhere, as well as our environment.