Researchers have leveraged material informatics to develop high-performance solid electrolytes for rechargeable batteries, paving the way for safer, more energy-dense, and longer-lasting power sources. This breakthrough could revolutionize the electric vehicle industry and promote the widespread adoption of renewable energy. Rechargeable batteries and solid-state batteries are key technologies for a sustainable future.
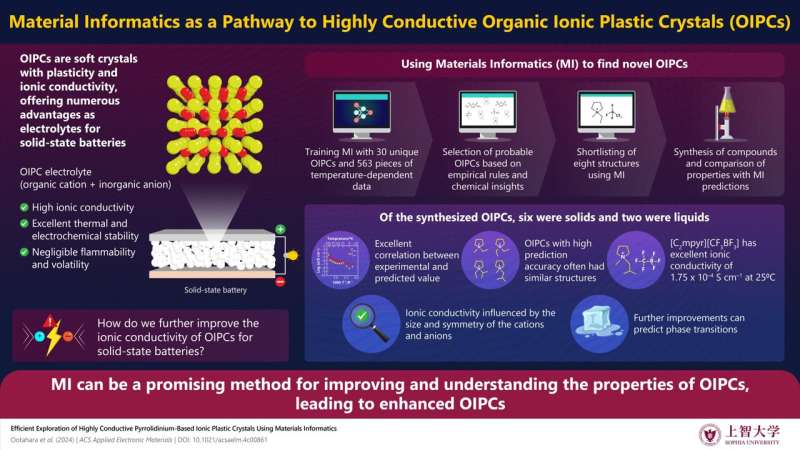
High-throughput Data-Driven Discovery and Development
The large amount of renewable energy and the progress of electric vehicles currently on the market has also caused high-performance all solid-state batteries to be in demand. In comparison to traditional liquid electrolyte-based batteries, solid-state storage comes with many benefits such as greater energy density, higher safety, longer cycle life and no need for a dedicated temperature control system.
Several challenges including the low ionic conductivity, high interfacial resistance and particle-particle interfaces existing in the electrolyte have impeded their comprehensive utilizationization. Two broad categories of solid electrolytes — inorganic and organic — have been looked at extensively by researchers, each with its own advantages and downsides. To solve these problems, the researchers focused on a novel class of materials called organic ionic plastic crystals (OIPCs).
Material Informatics to Discover Highly Conductive OIPCs
Solid electrolytes made of both lithium salts and OIPCs (organic cation-based ionic compounds, for example an organic amine and a particular inorganic anion) have numerous advantages. The materials demonstrate high ionic conductivity, outstanding stability, and the lowest flammability possible for rechargeable organic lithium batteries. OIPCs are characterized by their ability to undergo a phase change between a solid crystalline state and a liquid, or molten, state — called the plastic crystal state.
For the improvement of ionic conductivity in OIPCs, we utilize material informatics (MI), a function of informational science such as statistical science and machine learning that leads to accelerated development of materials. After constructing a training set with the relevant OIPC literature data for selected chemical structures and corresponding conductivity values, researchers validated performance of MI model based prediction using two additional test compounds. ResultsThe results indicate that the prediction accuracy is able to improve with same chemical structures in the training examples.
Click here to Unlock the Power of Pyrrolidinium OIPCs!
With the Thesis Space ML models also presenting with issues in selecting candidate substances, due to them generally just identifying CHOS cations [26], the researchers chose pyrrolidinium cations (which were well-represented in the training data) as candidates based on these insights. In addition to this, they employed MI so as to shortlist other candidate materials as per the empirical observations from earlier studies that were directed at bettering ionic conductivity in pyrrolidinium cation OIPCs.
Thus, eight new compounds were synthesized: six OIPC and two ionic liquids. One of these compounds showed a maximum ionic conductivity of 1.75 × 10-4 S cm-1 at 25°C which is just after the highest values obtained in such materials. Moreover, the MI results also provided a guideline for developing high performance OIPCs to improve ionic radius and ionic conductivity of materials, in which the optimal value was presented instead of the conventional empirical rules.