Researchers at the Chinese Academy of Sciences have developed neural network models that can quickly and accurately predict ion temperature and rotation velocity in fusion experiments. This breakthrough could significantly advance fusion technology by providing critical data for optimizing plasma performance and stability.
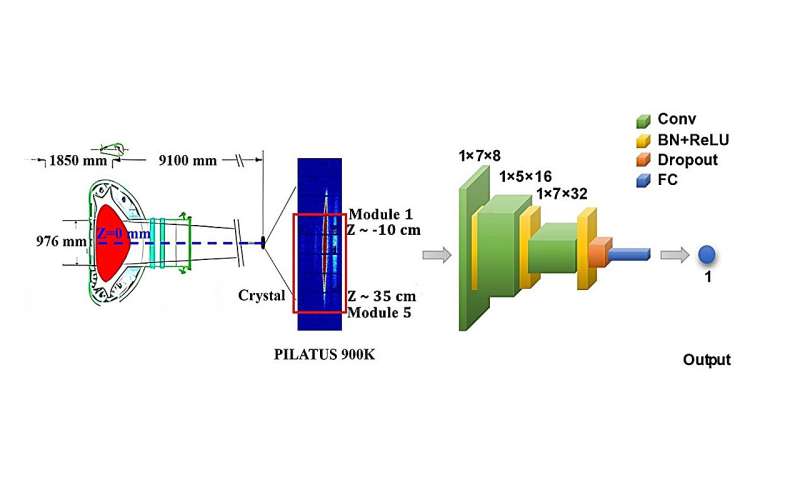
Harnessing the Power of Neural Networks for Fusion Research
In the world of fusion energy research, understanding the behavior of plasma – the superheated, ionized gas that fuels fusion reactions – is absolutely crucial. Two key parameters that play a critical role in plasma stability and performance are ion temperature and rotation velocity. However, accurately measuring these values has long been a significant technical challenge for scientists operating fusion reactors.
That is, until now. A team of researchers at the Hefei Institutes of Physical Science of the Chinese Academy of Sciences, led by Prof. Lyu Bo, have developed a groundbreaking solution: the application of artificial neural networks to X-ray Crystal Spectroscopy (XCS) data. Their findings, published in the prestigious journal Nuclear Fusion, demonstrate the power of this approach to rapidly and reliably predict ion temperature and rotation velocity profiles in the Experimental Advanced Superconducting Tokamak (EAST) fusion device.
Unleashing the Speed and Accuracy of Neural Networks
The researchers created two distinct models – Deep Neural Networks (DNN) and Convolutional Neural Networks (CNN) – both of which are capable of performing real-time calculations. Validation tests confirmed that these neural network-based predictions closely matched the actual data collected from the EAST fusion device.
One of the most remarkable outcomes of this study was the significant speed improvement offered by the DNN model. It was found to be more than 10 times faster than traditional methods, providing quick results without compromising accuracy. This breakthrough paves the way for more intelligent and automated diagnostic systems in the future, as the models can also assess the range and errors of input data.
The CNN models, on the other hand, successfully predicted line-integrated rotation velocity profiles and localized radial ion temperature profiles, further demonstrating their reliability and versatility. This adaptability means that the neural network models developed in this study can be applied to a wide range of diagnostic systems beyond the current research, making them highly valuable for the broader fusion community.
Advancing Fusion Technology through Intelligent Diagnostics
The implications of this research are profound for the future of fusion energy. By enhancing the accuracy and speed of predicting ion temperature and rotation velocity profiles, the team has provided a critical tool for optimizing plasma performance and stability in fusion reactors.
Accurate and timely data on these parameters is essential for researchers and engineers to make informed decisions and adjustments to the fusion process, ultimately accelerating the development of viable fusion technology. Furthermore, the adaptable and automated nature of the neural network models opens the door for more intelligent and adaptive diagnostic systems, which could revolutionize the way fusion experiments are conducted and analyzed.
As the global push for clean, sustainable energy sources continues, the advancements made by this research team at the Chinese Academy of Sciences stand as a shining example of how the latest in artificial intelligence and machine learning can be leveraged to overcome longstanding challenges in the field of fusion energy. With the promise of rapid, reliable predictions of key plasma parameters, the future of fusion research has never looked brighter.