Researchers have developed an innovative approach that combines deep reinforcement learning and fuzzy logic to identify the most suitable nanomaterials for harvesting energy from road traffic. This groundbreaking technique can optimize energy output while considering crucial factors like durability, cost, and environmental impact. By integrating advanced machine learning algorithms and expert knowledge, the researchers have paved the way for more efficient and sustainable energy solutions. Nanomaterials hold immense promise in revolutionizing renewable energy technologies, and this research represents a significant step forward in harnessing their full potential.
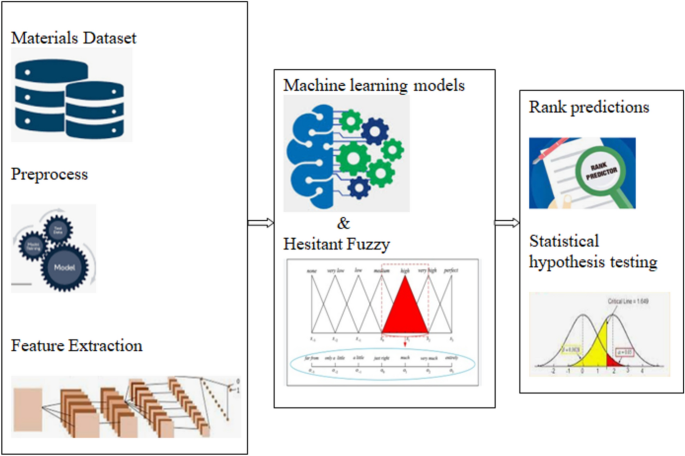
The Quest for Sustainable Energy Solutions
As the world grapples with the pressing need for renewable and eco-friendly energy sources, researchers have turned their attention to the remarkable capabilities of nanomaterials. These materials, which operate at the nanoscale (1-100 nanometers), possess unique properties that can greatly enhance the efficiency of energy harvesting systems. From piezoelectricity and thermoelectricity to ferroelectricity and photovoltaics, nanomaterials have the potential to revolutionize the way we capture and harness energy from the environment.
The Challenges of Nanomaterial Selection
However, the selection of the optimal nanomaterial for a specific energy harvesting application is a complex and multifaceted challenge. Factors such as energy output, durability, cost, and environmental impact must all be carefully considered to ensure the long-term sustainability and viability of the energy harvesting system. Traditional decision-making methods often fall short in effectively balancing these competing priorities, leading to suboptimal choices.
A Breakthrough in Nanomaterial Selection
To address this challenge, researchers have developed a groundbreaking approach that combines the power of deep reinforcement learning and fuzzy logic. By integrating the Fuzzy PROMETHEE decision-making method, the researchers have created a highly versatile and adaptable system for selecting the best nanomaterials for energy harvesting.
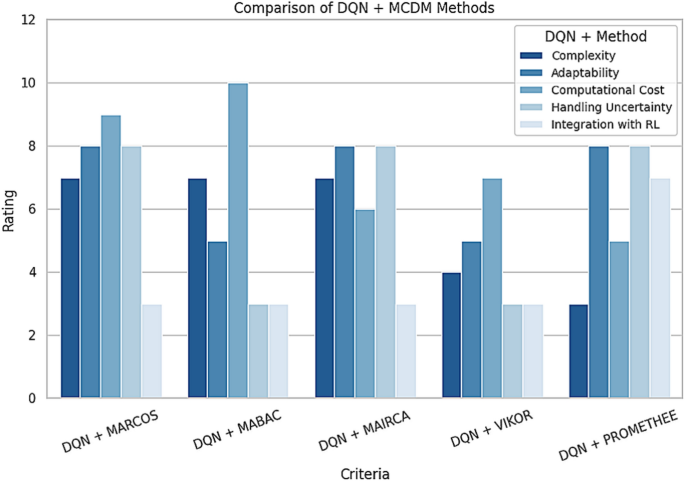
The Power of the Hybrid Approach
The DQN component of the model is trained to navigate the complex and dynamic environment of road traffic, learning from experience to select the nanomaterials that deliver the highest energy output. Meanwhile, the Fuzzy PROMETHEE component incorporates the expert knowledge and qualitative preferences of human decision-makers, ensuring that factors beyond just the quantitative data are taken into account.
This hybrid approach allows the model to adapt to changing conditions, such as fluctuations in traffic patterns or environmental factors, while also ensuring that the selected nanomaterials are not only highly efficient but also durable, cost-effective, and environmentally friendly.
Proven Results and Future Potential
The researchers’ findings demonstrate the remarkable success of this integrated approach, with the proposed model achieving an accuracy of 95% and a computational efficiency of 300 seconds. Practical testing on the selected nanomaterials has further validated the high energy-harvesting efficiency of the chosen materials under real-world, fluctuating traffic conditions.
As the global demand for sustainable energy solutions continues to grow, this breakthrough in nanomaterial selection represents a significant step forward. By harnessing the power of machine learning and expert knowledge, the researchers have paved the way for a more comprehensive and adaptable approach to energy harvesting that can positively impact the future of renewable energy technologies.
Author credit: This article is based on research by Sekar Kidambi Raju, Ganesh Karthikeyan Varadarajan, Amal H. Alharbi, Subhash Kannan, Doaa Sami Khafaga, Raj Anand Sundaramoorthy, Marwa M. Eid, S. K. Towfek.
For More Related Articles Click Here