Researchers have developed an innovative hexagonal deployment model for wireless sensor networks (WSNs) that can transform precision agriculture. This model integrates advanced features like adaptive frequency-hopping spread spectrum and decentralized real-time adaptation to optimize data transmission in dynamic agricultural environments. The study showcases the model’s exceptional performance, achieving low latency, high reliability, and efficient obstacle management – positioning it as a promising solution for enhancing environmental monitoring in precision farming.
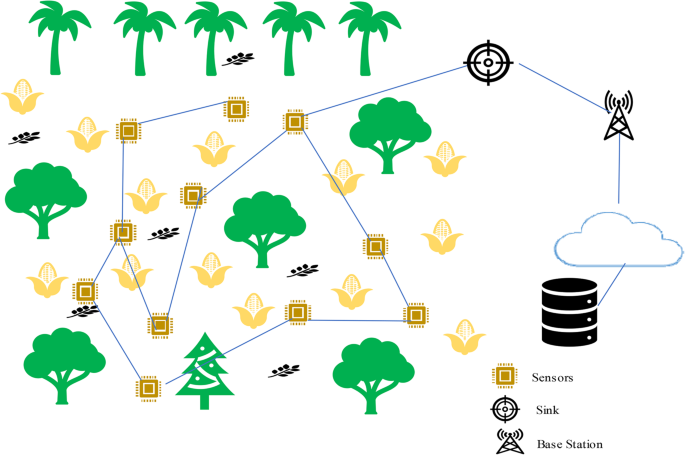
Navigating the Challenges of Wireless Sensor Networks in Agriculture
Wireless sensor networks (WSNs) have emerged as a crucial tool for precision agriculture, enabling real-time monitoring of essential environmental parameters like soil moisture, temperature, and crop health. However, deploying WSNs in agricultural settings poses unique challenges. The dynamic, volatile, and unpredictable nature of these environments can compromise uniform coverage and effective communication, while the constrained resources of individual sensor nodes add to the complexity.
Hexagonal Deployment: A Nature-Inspired Solution
Drawing inspiration from the natural world, researchers developed an innovative hexagonal deployment model to address the limitations of traditional WSN deployment strategies. This model strategically positions sensor nodes in a hexagonal grid pattern, ensuring uniform coverage of the agricultural field. The hexagonal layout offers several advantages:
– Efficient Resource Utilization: The hexagonal shape allows for more uniform distribution of radiation, leading to efficient use of resources.
– Improved Connectivity: The hexagonal arrangement ensures that each sensor node has up to six neighboring nodes, enhancing the network’s connectivity and reliability.
– Scalability: The modular nature of the hexagonal grid makes it easy to expand the network as the monitoring area grows, without compromising performance.
Adaptive Features for Dynamic Environments
To further enhance the resilience of the WSN, the researchers incorporated two key features:
1. Adaptive Frequency-Hopping Spread Spectrum (AFHSS): This mechanism dynamically adjusts frequency channels, mitigating interference and fortifying the network’s robustness against external disruptions.
2. Decentralized Real-Time Adaptation: This empowers individual sensor nodes to autonomously respond to ever-changing environmental conditions, optimizing data transmission efficiency.
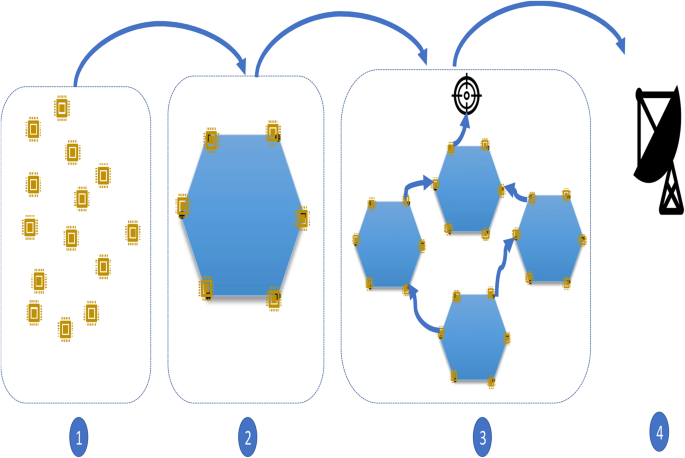
Exceptional Performance in Precision Agriculture
The researchers conducted extensive simulations to evaluate the performance of the hexagonal deployment model in diverse terrains and realistic sensor node distributions. The results were impressive:
– Latency: The protocol achieves an average latency of just 50 milliseconds, ensuring timely data delivery.
– Packet Loss Rate: The network maintains a packet loss rate below 2%, demonstrating exceptional reliability.
– Success Rate: The protocol exceeds a 95% success rate in data transmission.
– Obstacle Management: Less than 5% of the nodes require adjustment to accommodate obstacles, showcasing the model’s adaptability.
These compelling outcomes underscore the protocol’s exceptional ability to deliver responsive and reliable data transmission, positioning it as a promising solution for enhancing environmental monitoring in precision agriculture.
Paving the Way for the Future of Precision Farming
The findings of this study provide valuable insights and a robust foundation for researchers and practitioners engaged in designing and implementing resilient WSNs for precision agriculture applications. By integrating advanced features like AFHSS and decentralized real-time adaptation, the proposed hexagonal deployment model demonstrates its potential to revolutionize the way we monitor and manage agricultural environments, ultimately leading to more sustainable and efficient farming practices.
Author credit: This article is based on research by Zhang Yinjun.
For More Related Articles Click Here