In the complex world of supply chain management, predicting and optimizing distribution costs is crucial for businesses striving to improve efficiency and profitability. Researchers have found that Convolutional Neural Networks (CNNs), a powerful deep learning technique, outperform conventional machine learning models in accurately forecasting supply chain management distribution costs (SCMDC). This breakthrough has the potential to transform how organizations manage their supply chains, ultimately leading to enhanced customer satisfaction and increased competitiveness.
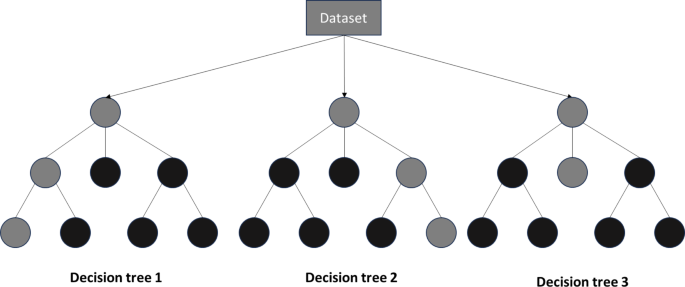
The Challenge of Supply Chain Optimization
Effective supply chain management is essential for organizations seeking to achieve their strategic goals and optimize performance. One critical aspect of this is accurately predicting the distribution costs within the supply chain, known as SCMDC. However, this has traditionally been a complex and challenging task, as supply chain networks are influenced by a myriad of factors, including fluctuating demand, transportation inefficiencies, and dynamic market conditions.
Leveraging the Power of Deep Learning
In recent years, the integration of advanced machine learning and deep learning models has revolutionized the field of supply chain management. Researchers have found that Convolutional Neural Networks (CNNs), a powerful deep learning technique, can outperform conventional machine learning algorithms in accurately predicting SCMDC.
CNNs are particularly well-suited for this task due to their ability to automatically learn hierarchical features, capture spatial and temporal patterns, and maintain computational efficiency through weight sharing. These attributes enable CNNs to handle complex, large-scale datasets with minimal preprocessing requirements, making them a preferred choice for precise and reliable SCMDC predictions.
Comparing Deep Learning and Conventional Algorithms
The study examined the performance of various machine learning algorithms, including Random Forest (RF), Support Vector Machine (SVM), Multilayer Perceptron (MLP), and Decision Tree (DT), alongside the deep learning-based CNN model. Using a comprehensive dataset of 180,519 open-source data points, the researchers evaluated the models’ predictive accuracy based on Root Mean Square Error (RMSE) and Correlation Coefficient (R2).
The results were quite remarkable: the CNN model demonstrated exceptional accuracy, achieving an RMSE of 0.528 and an R2 value of 0.953 on the test dataset. This significantly outperformed the other machine learning algorithms, which had higher RMSE values and lower R2 scores.
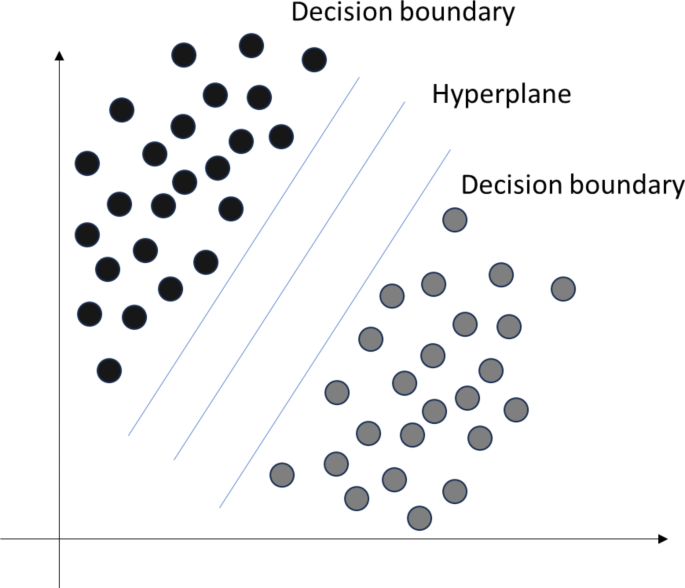
Fig. 2
Unlocking New Possibilities in Supply Chain Management
The superior performance of CNNs in SCMDC prediction has far-reaching implications for the future of supply chain management. By leveraging the power of deep learning, organizations can make more informed and timely decisions, leading to improved cost management, better resource allocation, and enhanced customer satisfaction.
Moreover, the real-time predictive capabilities of CNNs enable businesses to quickly respond to fluctuations in demand, transportation costs, and other dynamic variables, reducing the risk of bottlenecks and inefficiencies. This agility is particularly crucial in today’s rapidly evolving business landscape.
The Road Ahead
As the integration of artificial intelligence and deep learning continues to reshape various industries, the future of supply chain management looks brighter than ever. Researchers are now exploring ways to further enhance the capabilities of CNNs by integrating them with emerging technologies, such as real-time data analytics and advanced optimization techniques.
By harnessing the power of these cutting-edge tools, businesses can expect to see even greater improvements in their supply chain performance, ultimately leading to increased profitability, enhanced competitiveness, and better customer experiences.
Author credit: This article is based on research by Xiaomo Yu, Ling Tang, Long Long, Mohammad Sina.
For More Related Articles Click Here