Bearing failures are a leading cause of electrical machine breakdowns, making reliable condition monitoring essential for industrial operations. Researchers have developed a novel hybrid model that combines the strengths of Long Short-Term Memory (LSTM) networks and Random Forest (RF) classifiers, further enhanced by the Grey Wolf Optimization (GWO) algorithm. This innovative approach significantly improves the accuracy and reliability of bearing fault detection, outperforming standalone models and offering a valuable tool for predictive maintenance. By integrating advanced machine learning techniques, this research paves the way for more robust and adaptive solutions in the realm of industrial diagnostics. Machine learning and artificial intelligence are revolutionizing the way we approach critical infrastructure monitoring and maintenance.
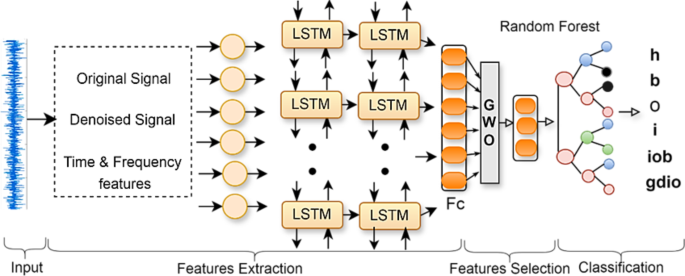
Tackling the Challenges of Bearing Fault Diagnosis
Rolling bearings are essential components in a wide range of industrial machinery, from manufacturing plants to heavy-duty equipment. These bearings facilitate the smooth rotation of shafts and other moving parts, minimizing friction and wear. However, the continuous use of bearings under demanding conditions can lead to gradual deterioration and eventual failure, which can have catastrophic consequences for the entire system.
Accurate and timely fault detection is crucial to prevent unexpected breakdowns, ensure safety, and maintain operational efficiency. Traditional fault diagnosis methods often rely on manual inspection or standalone machine learning models, which can be limited in their ability to capture the complex, dynamic nature of bearing faults.
Integrating the Power of LSTM, Random Forest, and Grey Wolf Optimization
To address these challenges, a team of researchers has developed a novel hybrid model that combines the strengths of LSTM networks, Random Forest classifiers, and the Grey Wolf Optimization (GWO) algorithm. This innovative approach leverages the sequential data processing capabilities of LSTM, the robust classification abilities of Random Forest, and the feature optimization prowess of GWO to enhance the accuracy and reliability of bearing fault detection.
The three-stage hybrid model works as follows:
1. Feature extraction: Time and frequency domain features are manually extracted from vibration signals, providing a comprehensive representation of the bearing’s operational state.
2. LSTM processing: The extracted features are fed into a dual-layer LSTM network, which is designed to capture complex temporal relationships within the data.
3. GWO-optimized Random Forest classification: The GWO algorithm is employed to select the most relevant features from the LSTM outputs, which are then used to train the Random Forest classifier for fault categorization.
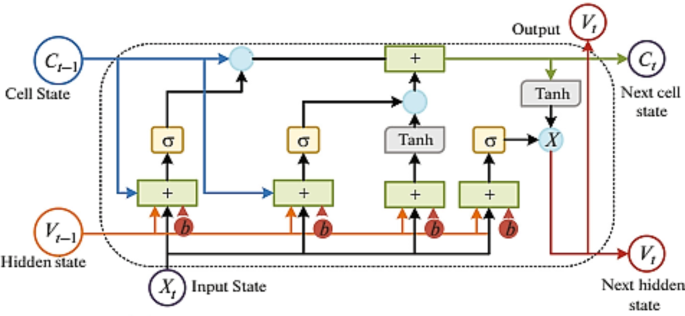
Unlocking Improved Diagnostic Accuracy
The researchers rigorously evaluated the performance of the hybrid LSTM-RF-GWO model using a comprehensive dataset covering six distinct bearing health conditions: healthy, outer race fault, ball fault, inner race fault, compounded fault, and generalized degradation.
The results were remarkable, with the hybrid model achieving a classification accuracy of 98.97% – a significant improvement over standalone LSTM (93.56%) and Random Forest (98.44%) models. Furthermore, the inclusion of the GWO algorithm led to an additional 0.39% increase in accuracy compared to the hybrid LSTM-RF model without optimization.
Other key performance metrics, such as precision, kappa coefficient, false negative rate (FNR), and false positive rate (FPR), were also significantly enhanced. The precision reached 99.28%, the kappa coefficient achieved 99.13%, and the FNR and FPR were reduced to 0.0071 and 0.0015, respectively.
Towards Robust and Adaptive Predictive Maintenance
The superior performance of the hybrid LSTM-RF-GWO model highlights its potential as a valuable tool for predictive maintenance in industrial applications. By combining advanced machine learning techniques, the framework not only improves fault detection accuracy but also provides a robust solution for distinguishing between closely related fault conditions.
This research represents a significant step forward in the field of industrial diagnostics, paving the way for more reliable and adaptable systems that can ensure the longevity of critical machinery, enhance safety, and optimize operational efficiency. As the demand for smart, data-driven maintenance strategies continues to grow, innovative hybrid approaches like this could become indispensable in the quest for industrial resilience and sustainability.
Author credit: This article is based on research by Said Djaballah, Lotfi Saidi, Kamel Meftah, Abdelmoumene Hechifa, Mohit Bajaj, Ievgen Zaitsev.
For More Related Articles Click Here