Researchers have found a novel way to evaluate the speech information available to cochlear implant users – by using advanced deep learning speech recognition models. This approach offers significant time and cost savings compared to traditional human subject studies, while providing a comprehensive understanding of how different cochlear implant settings affect speech intelligibility. The findings have important implications for optimizing cochlear implant technology and improving outcomes for people with hearing loss. Cochlear implants are devices that directly stimulate the auditory nerve, helping to restore hearing for individuals with profound deafness.
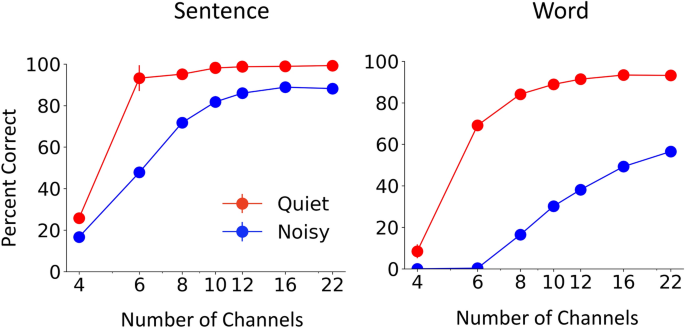
Revolutionizing Cochlear Implant Research with Deep Learning
Traditionally, the intelligibility of cochlear implant simulations has been assessed through speech recognition experiments with normally-hearing human subjects – a time-consuming and expensive process. However, a team of researchers from New York University has found a game-changing alternative: employing advanced deep learning speech recognition models.
The study, led by Rahul Sinha and Mahan Azadpour, utilized the Whisper model, an open-source deep learning speech recognition system developed by OpenAI. The researchers evaluated the Whisper model’s performance on vocoder-processed words and sentences, where the vocoder simulates the sound processing in cochlear implants.
Uncovering the Secrets of Cochlear Implant Intelligibility
The researchers manipulated various vocoder parameters, such as the number of spectral channels, envelope frequency range, and envelope dynamic range, to mimic the signal processing and perceptual limitations experienced by cochlear implant users. Interestingly, the Whisper model exhibited a human-like response to these alterations, closely resembling the results of previous studies conducted with human participants.
“Despite not being originally designed to mimic human speech processing, the Whisper model was able to provide a comprehensive picture of how different cochlear implant settings affect speech intelligibility,” explained Mahan Azadpour, the corresponding author of the study.
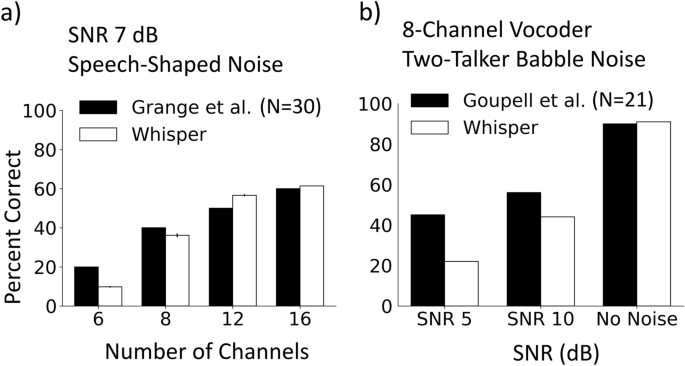
Fig. 2
Unlocking the Potential of Deep Learning in Auditory Research
The findings of this study demonstrate the potential of employing speech recognition models as a computational tool in auditory research. These models can provide valuable insights into the factors that contribute to poor speech perception outcomes in cochlear implant users, such as the limited number of spectral channels or the elimination of temporal fine structure.
“The ability to assess large-scale sets of speech processing parameters without the need for human subject testing is a game-changer,” said Rahul Sinha, the first author of the study. “This approach can significantly accelerate research and clinical practice in the field of cochlear implants.”
Optimizing Cochlear Implants for Better Outcomes
The researchers believe that the insights gained from this study can help guide the optimization of cochlear implant signal processing and sound coding strategies. By understanding the specific cues that contribute to speech intelligibility, researchers and clinicians can work towards enhancing the performance of these devices and improving the quality of life for individuals with hearing loss.
“This study is a testament to the power of leveraging advanced artificial intelligence techniques to solve complex problems in the field of hearing science,” Azadpour added. “As we continue to push the boundaries of technology, we can unlock new possibilities for improving the lives of those with hearing impairments.”
Author credit: This article is based on research by Rahul Sinha, Mahan Azadpour.
For More Related Articles Click Here