Aging is a complex process that affects us all, but did you know that our DNA holds the key to understanding it? In a groundbreaking study, researchers have explored the correlation between DNA methylation and biological age using advanced machine learning techniques. DNA methylation is a crucial epigenetic process that can influence gene expression without altering the DNA sequence itself. By analyzing large datasets of human methylation data, the researchers have identified specific methylation sites that are strongly associated with biological age, providing valuable insights into the mechanisms of aging. This research could have significant implications for the prevention and treatment of age-related diseases, opening up new avenues for personalized healthcare and a better understanding of the aging process.
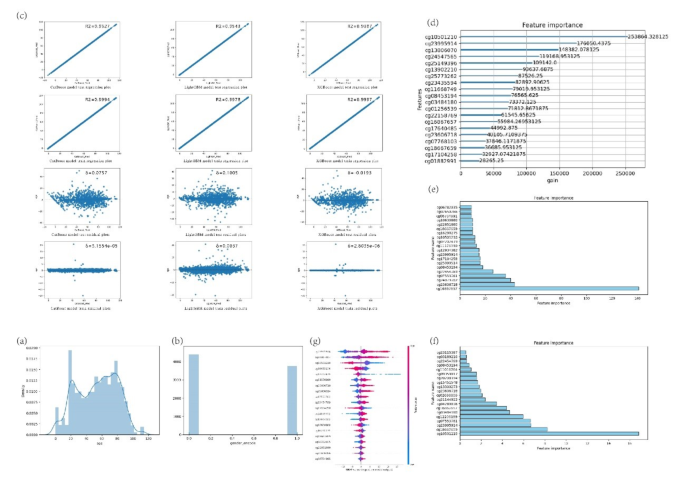
Uncovering the Link Between DNA Methylation and Biological Age
DNA methylation is a widespread epigenetic phenomenon that plays a crucial role in regulating gene expression. As we age, our DNA undergoes systematic changes in methylation patterns, which can be used as markers to predict an individual’s biological age. In this study, the researchers set out to explore this relationship using an interpretable machine learning framework.
The researchers began by analyzing a large dataset of human methylation data, which included over 10,000 samples. They preprocessed the data, performed feature selection, and then used various machine learning models, including XGBoost, LightGBM, and CatBoost, to construct a biological age prediction model.
Identifying Key Methylation Sites Associated with Aging
The feature selection process revealed 15 groups of methylation sites that were strongly associated with biological age. The researchers then used the Shapley Additive Explanations (SHAP) framework to calculate the contribution of each methylation site to the biological age prediction. They found that the cg23995914 locus was the most significant contributor, with the corresponding gene being ZNF518B.
Further analysis using Gene Ontology (GO) enrichment and Kyoto Encyclopedia of Genes and Genomes (KEGG) revealed that the genes associated with the 15 methylation sites were involved in important biological processes, such as fatty acid elongation, long-chain fatty acid biosynthesis, cell cycle regulation, and alpha-linolenic acid metabolism. These findings suggest that the identified methylation sites may play a crucial role in regulating cellular and tissue aging.
Implications for Age-Related Disease Prevention and Treatment
The researchers’ findings have important implications for the prevention and treatment of age-related diseases. By understanding the specific methylation sites and associated genes that contribute to biological aging, healthcare professionals can develop targeted strategies for early detection, personalized interventions, and potentially even slowing down the aging process.
Additionally, the use of interpretable machine learning frameworks, such as the one employed in this study, can help improve the transparency and explainability of age prediction models. This can lead to better-informed decision-making and a deeper understanding of the underlying biological mechanisms at play.
Paving the Way for a Healthier Future
This research represents a significant step forward in our understanding of the relationship between DNA methylation and biological age. By leveraging the power of machine learning and exploring the biological significance of key methylation sites, the researchers have opened up new avenues for the prevention and management of age-related diseases.
As we continue to unravel the complexities of the aging process, studies like this will play a crucial role in shaping the future of personalized healthcare and our collective pursuit of a healthier, longer-lasting life.
Author credit: This article is based on research by Sheng Zhou, Jing Chen, Shanshan Wei, Chengxing Zhou, Die Wang, Xiaofan Yan, Xun He, Pengcheng Yan.
For More Related Articles Click Here