Researchers have developed a groundbreaking artificial intelligence (AI) system that can accurately predict the risk of a dangerous heart condition called Torsades de Pointes (TdP) caused by certain drugs. This advance could revolutionize the way we assess the safety of new medications, helping to ensure they don’t cause potentially life-threatening heart problems. The study utilized explainable AI techniques to identify the most influential factors, or “biomarkers,” that contribute to TdP risk, providing crucial insights into the complex mechanisms behind drug-induced cardiac toxicity.
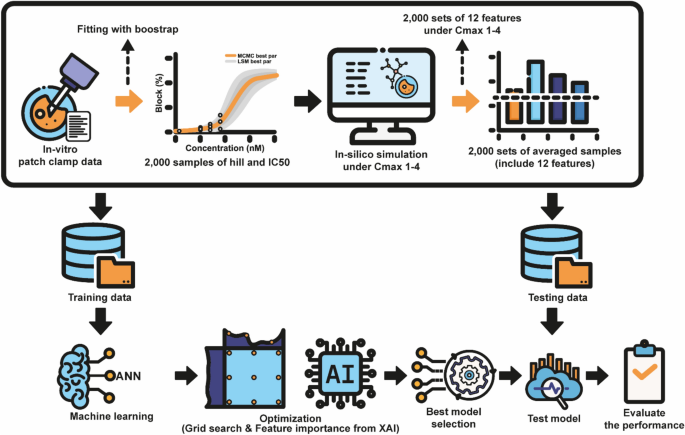
Unraveling the Complexity of Drug-Induced Heart Risks
Evaluating the potential for new drugs to cause dangerous heart rhythm disorders, such as TdP, is a critical step in the drug development process. TdP is a hazardous condition characterized by the twisting of the heart’s electrical signals, which can lead to sudden cardiac arrest. The Comprehensive In-vitro Proarrhythmia Assay (CiPA) initiative was established to address this issue, using a combination of laboratory experiments and computer simulations to assess a drug’s proarrhythmic potential.
Harnessing the Power of Explainable AI
In this study, the researchers leveraged the power of explainable artificial intelligence (XAI) to delve deeper into the factors that influence a drug’s ability to cause TdP. They used a technique called SHAP (SHapley Additive exPlanations) to quantify the contribution of 12 different “in-silico biomarkers” derived from computer simulations of cardiac cell behavior. These biomarkers, such as action potential duration, calcium dynamics, and ionic current characteristics, provide a comprehensive representation of the complex interplay between drugs and the heart’s electrical activity.
Identifying the Key Biomarkers for Accurate Risk Prediction
The researchers tested several machine learning models, including Artificial Neural Networks (ANN), Support Vector Machines (SVM), Random Forests (RF), and others, to classify drugs into high, intermediate, and low-risk categories for TdP. By examining the SHAP values for each biomarker, they were able to determine the most influential factors in the predictive process.
The ANN model, which demonstrated the highest overall accuracy, highlighted the critical importance of the qInward biomarker, which reflects the flow of charged particles into the cardiac cells. This underscores the fundamental role of ion balance in maintaining healthy heart rhythms and the disruption that can lead to dangerous arrhythmias.
Optimizing Feature Selection for Enhanced Predictions
The researchers also explored the impact of feature selection, or the choice of which biomarkers to include in the models. They found that reducing the number of input features could, in some cases, improve the models’ ability to accurately predict TdP risk, particularly at the high and low-risk levels. This suggests that carefully curating the most influential biomarkers is crucial for developing reliable and efficient risk assessment tools.
Advancing Cardiac Safety Evaluation
The findings of this study represent a significant step forward in the field of cardiac safety assessment, providing a systematic and data-driven approach to identifying the key factors that contribute to drug-induced arrhythmia risk. By leveraging the power of XAI, the researchers have not only enhanced the accuracy of TdP predictions but also shed light on the complex interplay between drugs and the heart’s electrical system.
This research has the potential to streamline the drug development process, helping pharmaceutical companies and regulatory agencies make more informed decisions about the safety of new medications. By focusing on the most critical biomarkers, drug developers can optimize their testing strategies and improve the overall safety of the drugs that reach the market, ultimately protecting the health and well-being of patients.
Author credit: This article is based on research by Muhammad Adnan Pramudito, Yunendah Nur Fuadah, Ali Ikhsanul Qauli, Aroli Marcellinus, Ki Moo Lim.
For More Related Articles Click Here