Breast cancer remains a formidable challenge, with drug resistance posing a significant obstacle to successful treatment. However, a team of researchers has developed a groundbreaking deep learning model, called ResisenseNet, that could revolutionize the way we approach this battle. By integrating transcription factor expression, genomic markers, drug data, and molecular descriptors, ResisenseNet can accurately predict drug sensitivity and resistance, paving the way for more effective personalized therapies. This innovative approach holds the potential to transform the landscape of cancer treatment, offering new hope for patients fighting this devastating disease. Breast cancer and drug resistance are complex challenges that require innovative solutions.
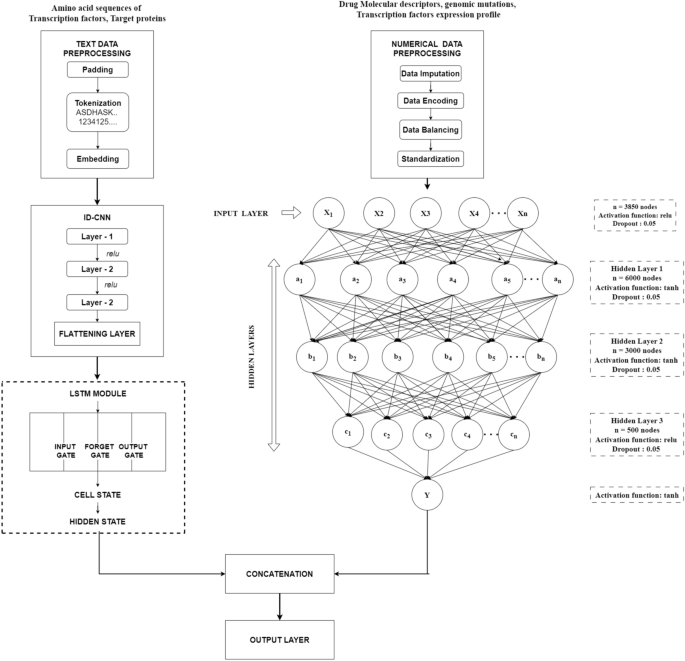
Unraveling the Complexities of Breast Cancer
Breast cancer is a leading cause of mortality among women worldwide, with drug resistance being a critical concern. Factors such as transcription factors and genomic mutations play a crucial role in determining treatment outcomes, highlighting the need for targeted intervention strategies. Transcription factors, like STAT3, can drive drug resistance and metastasis, while mutations in genes like BCR-ABL1 and p53 can also contribute to resistance. Exploring alternative drug molecules and repurposing existing ones have become essential to address this challenge.
ResisenseNet: A Game-Changer in Predictive Modeling
In response to this pressing need, researchers developed the ResisenseNet model, a hybrid neural network framework that integrates various data sources to predict drug sensitivity and resistance. ResisenseNet utilizes a unique combination of 1D-CNN (Convolutional Neural Network), LSTM (Long Short-Term Memory), and DNN (Deep Neural Network) architectures to effectively capture patterns from amino acid sequences, transcription factor expression, genomic markers, and drug molecules.
Validating the Predictive Power of ResisenseNet
The ResisenseNet model underwent rigorous validation, including ablation studies and comparisons with state-of-the-art models. The results were impressive, with the model achieving a validation accuracy of 0.9794 and a loss value of 0.042. This exceptional performance was further corroborated through extensive testing on diverse cancer datasets, demonstrating the model’s generalizability and robustness.
Unlocking the Potential of Drug Repurposing
One of the key features of the ResisenseNet model is its ability to identify existing drugs that could be repurposed for breast cancer treatment. By screening drugs from 14 different cancer types, the researchers found that certain drugs targeting Low-grade Glioma (LGG) and Lung Adenocarcinoma (LUAD) showed increased sensitivity in breast cancer. Remarkably, 14 of the identified sensitive drugs had no prior history of anticancer activity against breast cancer, presenting novel therapeutic opportunities.
Empowering Personalized Cancer Treatments
The ResisenseNet model’s ability to predict drug sensitivity and resistance not only enhances the drug discovery process but also paves the way for personalized cancer treatments. By understanding the complex interactions between transcription factors, genomic markers, and drug molecules, clinicians can tailor their approach to each patient’s unique cancer profile, leading to more effective and targeted therapies.
In conclusion, the development of the ResisenseNet model represents a significant step forward in the fight against breast cancer. By harnessing the power of artificial intelligence and deep learning, researchers have created a tool that can overcome the challenge of drug resistance, opening up new avenues for personalized cancer care and improved patient outcomes.
Author credit: This article is based on research by Anush Karampuri, Bharath Kumar Jakkula, Shyam Perugu.
For More Related Articles Click Here