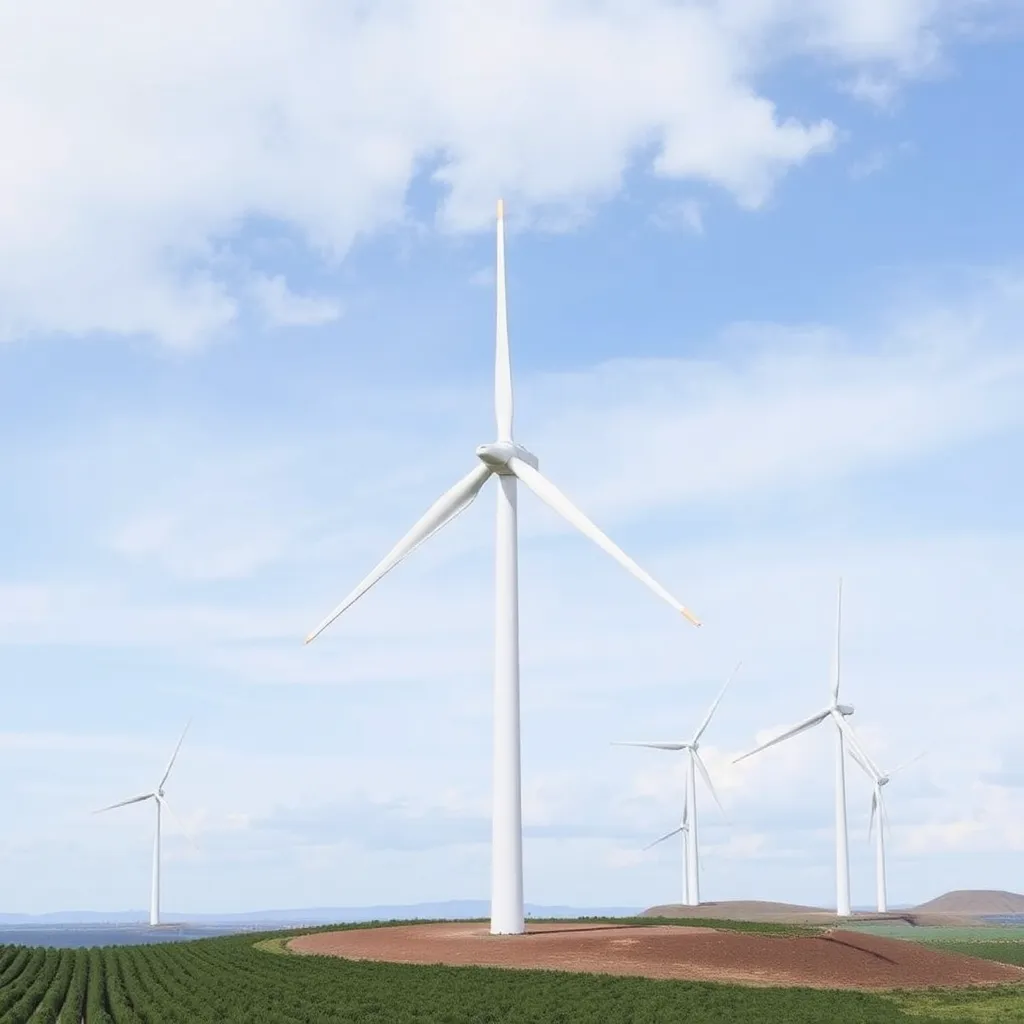
Wind power is a rapidly growing renewable energy source, but maintaining the intricate machinery of wind turbines can be a major challenge. Researchers from Beihua University have developed a cutting-edge deep learning algorithm that can detect surface defects in wind turbines with unprecedented speed and accuracy. This breakthrough has the potential to revolutionize wind turbine maintenance, improving efficiency and reducing operational costs. By utilizing advanced techniques like partial convolution and efficient multiscale attention, the researchers have created a lightweight, real-time object detection model that can accurately identify a variety of defects, from cracks and erosion to dirt and paint flaws. This innovative approach could have far-reaching implications for the renewable energy industry, helping to unlock the full potential of wind power and drive us closer to a sustainable energy future.
Optimizing Wind Turbine Maintenance with Deep Learning
Wind power is a rapidly growing source of renewable energy, with wind turbines now dotting landscapes across the globe. However, maintaining these complex machines can be a significant challenge. Wind turbines operate under demanding conditions, subjected to a variety of stresses and environmental factors that can lead to a range of surface defects, from cracks and erosion to dirt buildup and paint damage. Identifying and addressing these issues early on is crucial for maximizing the efficiency and lifespan of wind turbines, but traditional inspection methods can be time-consuming, costly, and often ineffective.
Unlocking the Power of Deep Learning
To tackle this challenge, researchers from Beihua University have developed a revolutionary deep learning algorithm capable of detecting surface defects in wind turbines with unprecedented speed and accuracy. By leveraging advanced techniques like partial convolution and efficient multiscale attention, the team has created a lightweight, real-time object detection model that can identify a wide variety of defects, from tiny cracks to large-scale erosion.
A Lightweight, High-Performance Solution
The key innovation behind this breakthrough is the researchers’ design of the PC-EMA block, which enhances the model’s ability to extract and process multiscale feature information efficiently. This module, which is incorporated into the backbone of the deep learning network, allows the algorithm to better capture the diverse characteristics of defective targets, even in complex environments.
To further optimize the model for real-world deployment, the researchers also introduced a number of other improvements, including the use of GSConv and VoVGSCSP modules in the neck network to reduce computational complexity, and a novel PConv Head design to streamline the detection process. Additionally, the team leveraged the WIoUv3 loss function, which helps the model focus on common quality samples and enhances its accuracy and localization capabilities.
Transforming Wind Turbine Maintenance
The result is a highly efficient, lightweight deep learning model that can be easily deployed on edge devices, such as the Click Here