Researchers have developed a powerful new AI model called GSCANet that can accurately diagnose Alzheimer’s disease (AD) using a combination of brain imaging, functional connectivity, and neuropsychological data. This innovative approach outperforms traditional single-modality methods, providing a more comprehensive understanding of the complex changes occurring in the brains of AD patients. By leveraging the strengths of different data sources, GSCANet shows promise in enabling early detection and tracking the progression of this devastating neurodegenerative disorder. This breakthrough could pave the way for better treatment and management strategies for the millions affected by Alzheimer’s disease.
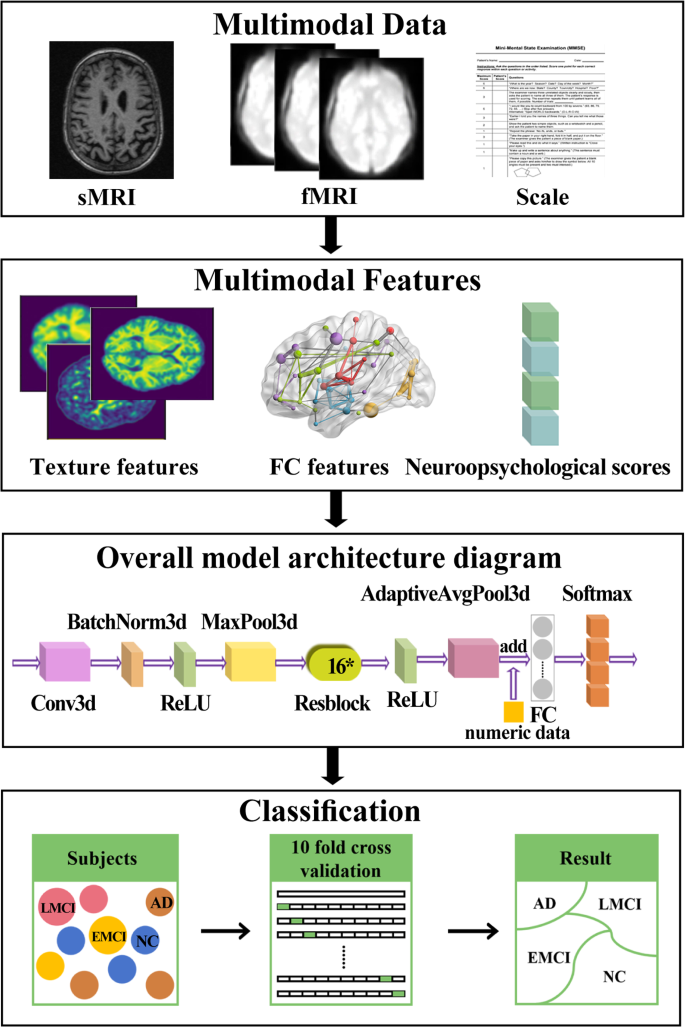
Unraveling the Complexity of Alzheimer’s Disease
Alzheimer’s disease is a debilitating neurodegenerative disorder that affects millions of people worldwide, predominantly the elderly. As the population ages, the prevalence of AD is rising rapidly, posing a significant public health challenge. While the disease progression is irreversible, early diagnosis and intervention can make a significant difference in slowing the onset and improving the quality of life for patients.
The complex changes in brain structure and function associated with AD, including cortical thinning, reduced gray matter volume, hippocampal atrophy, and weaker connectivity in the default mode network, make it a challenging condition to diagnose accurately. Traditional approaches that rely on a single data modality, such as structural MRI or functional MRI, only capture a partial picture of the underlying pathological changes.
Embracing Multimodal Data for Improved Diagnosis
To address these limitations, a team of researchers have developed a novel deep learning-based model called GSCANet (Group Self-Calibrated Coordinate Attention Network) that integrates multiple data sources, including:
1. magneticresonanceimaging’>Functional MRI – Functional connectivity measures that capture the altered patterns of neural activity and communication in the brain.
3. Neuropsychological assessments – Cognitive, emotional, and behavioral data from tests like the Mini-Mental State Examination (MMSE) and Clinical Dementia Rating (CDR).
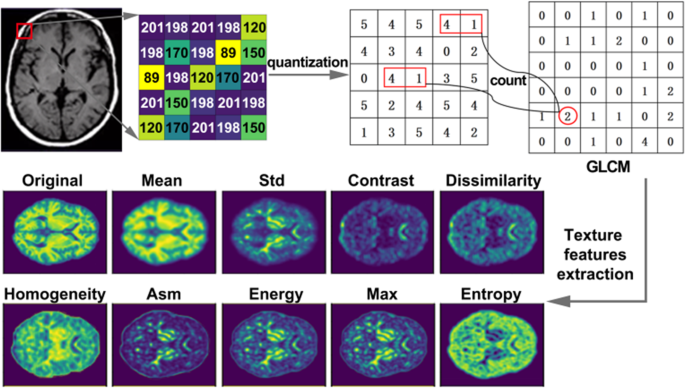
Enhancing Feature Extraction and Interactions
The key innovations of the GSCANet model lie in its ability to extract more comprehensive and relevant features from the multimodal data. By incorporating a group self-calibrated convolution module, the model can expand the receptive field and capture multi-scale features, enabling more accurate identification of subtle brain changes associated with AD.
Furthermore, the coordinate attention module embedded in the model facilitates the integration of spatial and channel-wise information, allowing for more effective interactions between different features. This targeted focus on critical information helps the model suppress less relevant details and capture a diverse set of features essential for accurate AD diagnosis.
Impressive Performance and Generalizability
The researchers evaluated the performance of GSCANet on both the widely used Alzheimer’s Disease Neuroimaging Initiative (ADNI) dataset and an independent in-house dataset. The results were impressive, with the model achieving:
– 78.70% accuracy in distinguishing between four groups: AD, late mild cognitive impairment (LMCI), early mild cognitive impairment (EMCI), and normal control (NC).
– 83.33% accuracy in the three-way classification of AD, MCI, and NC.
– 92.81% accuracy in the binary classification of AD and NC.
– 84.67% accuracy in differentiating between EMCI and LMCI.
Importantly, the model’s strong performance was maintained when tested on the independent in-house dataset, demonstrating its ability to generalize and perform well across different populations and data sources.
Paving the Way for Improved AD Management
The development of GSCANet represents a significant stride towards more accurate and earlier diagnosis of Alzheimer’s disease. By leveraging the complementary information from multimodal data sources, this innovative AI model can capture the complex pathological changes in the brain more comprehensively, leading to enhanced classification accuracy.
This advancement has the potential to revolutionize the way Alzheimer’s disease is detected and managed. Earlier identification of the disease can enable timely interventions, potentially slowing the progression and improving patient outcomes. Additionally, the model’s ability to differentiate between different stages of cognitive impairment (EMCI vs. LMCI) could aid in tracking disease progression and guiding treatment strategies.
As the field of Click Here