Researchers have made a groundbreaking discovery that could revolutionize our understanding of how the visual system processes information. By incorporating the complex, input-dependent nature of dendritic nonlinearities into their model, they have been able to explain a long-standing puzzle in spatial vision known as “plaid masking” – a phenomenon that the standard model of visual processing has failed to account for. This research not only sheds light on the intricate workings of the early visual system but also opens up new avenues for developing more accurate and comprehensive models of spatial vision. Visual system, Neuron, Dendrite
Unraveling the Mysteries of Plaid Masking
The human visual system is a remarkable feat of biological engineering, capable of processing and interpreting the complex patterns of light that reach our eyes. For decades, researchers have worked to develop models that can accurately describe and predict the behavior of this intricate system. One of the most successful of these models is the “standard model” (SM) of spatial vision, which has been able to account for a wide range of experimental data.
However, the SM has also faced significant challenges, particularly when it comes to explaining a phenomenon known as “plaid masking.” In this phenomenon, the detectability of a vertical sinusoidal grating signal is dramatically reduced when it is presented alongside a “plaid” mask – a pattern composed of two oblique gratings. Remarkably, the masking effect of the plaid is much stronger than the combined masking effects of the individual oblique gratings.
This “super-additive” masking effect has long been a thorn in the side of the standard model, which has been unable to account for it. The reason for this failure lies in the fundamental assumptions of the SM, which is based on a linear-nonlinear (LN) cascade model of visual processing.
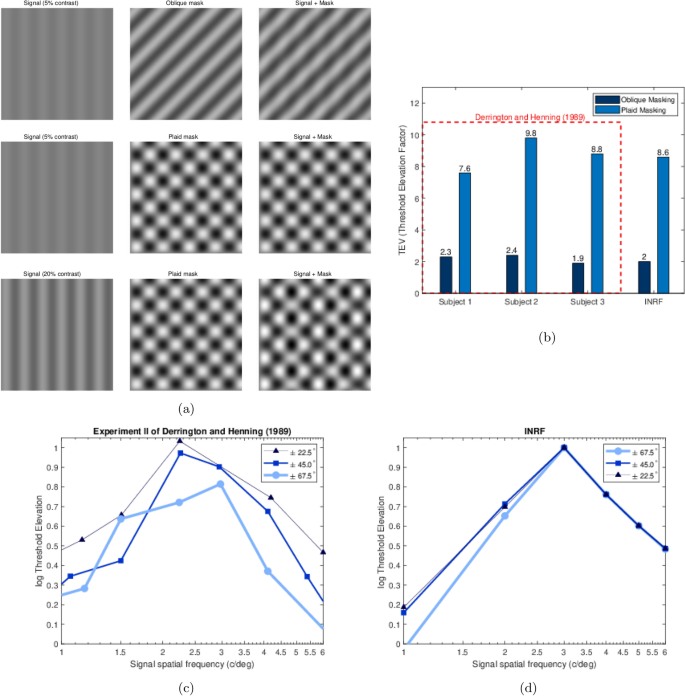
Rethinking the Standard Model: The Role of Dendritic Nonlinearities
The researchers behind the current study hypothesized that the key to understanding plaid masking lies in the complex, input-dependent nature of dendritic nonlinearities – the nonlinear computations that occur within the dendrites of individual neurons. These nonlinearities, which were largely ignored in the original formulation of the SM, play a crucial role in shaping the responses of neurons to visual stimuli.
To test this hypothesis, the researchers turned to the “Intrinsically Nonlinear Receptive Field” (INRF) model, which explicitly incorporates the input-dependent nature of dendritic nonlinearities. By applying this model to the plaid masking data, the researchers were able to reproduce the observed “super-additive” masking effect, something that the standard model had failed to do.
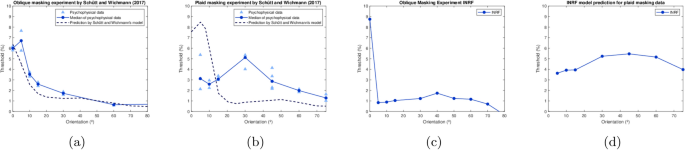
Fig. 2
Unraveling the Mysteries of Plaid Masking with the INRF Model
The INRF model works by considering the complex interplay between the input signal and the nonlinear computations occurring within the dendrites of individual neurons. Unlike the static nonlinearities assumed in the standard model, the INRF model uses an input-dependent nonlinearity that can adapt to the specific characteristics of the visual stimulus.
This flexibility allows the INRF model to capture the subtle, yet critical, differences in the way the visual system processes oblique and plaid masking stimuli. When the INRF model is applied to these different stimuli, the resulting receptive fields (RFs) of the simulated neurons change dramatically, reflecting the input-dependent nature of the dendritic nonlinearities.
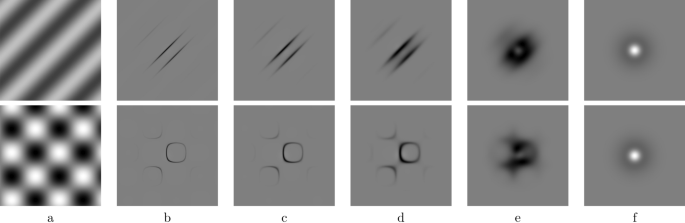
Fig. 3
Implications and Future Directions
The success of the INRF model in explaining plaid masking data has far-reaching implications for our understanding of the early visual system. It suggests that the key to unlocking the mysteries of spatial vision may lie not in the linear processing assumed by the standard model, but in the complex, nonlinear computations that occur within the dendrites of individual neurons.
Moreover, the researchers speculate that the relevant nonlinearities responsible for plaid masking may originate as early as in the retinal ganglion cells, challenging the traditional view that the critical computations happen at higher levels of the visual hierarchy.
Looking ahead, the researchers envision a future in which the INRF model is integrated into a more comprehensive, two-stage model of spatial vision. By combining the INRF’s ability to capture the input-dependent nature of dendritic nonlinearities with the strengths of the standard model, such a hybrid approach could provide a more accurate and complete understanding of how the visual system processes the rich tapestry of information that we encounter in the world around us.
Author credit: This article is based on research by Marcelo Bertalmío, Alexia Durán Vizcaíno, Jesús Malo, Felix A. Wichmann.
For More Related Articles Click Here