Bladder lesions are a common issue in patients with benign prostatic hyperplasia (BPH), a condition that affects many older men. Early detection and treatment of these lesions are crucial to prevent complications like acute urinary retention or kidney failure. However, current diagnostic methods can be inconvenient or invasive. In this study, researchers developed a machine learning-based model that can diagnose bladder lesions using clinical risk factors that can be easily obtained through non-invasive tests. The model identified four key factors – bladder compliance, maximum flow rate, prostate-specific antigen (PSA), and post-void residual urine – as the most important contributors to the diagnosis. This innovative approach could serve as a convenient and effective screening tool to help doctors identify bladder lesions early and provide timely treatment, potentially improving the quality of life for BPH patients. Benign prostatic hyperplasia, Urinary bladder
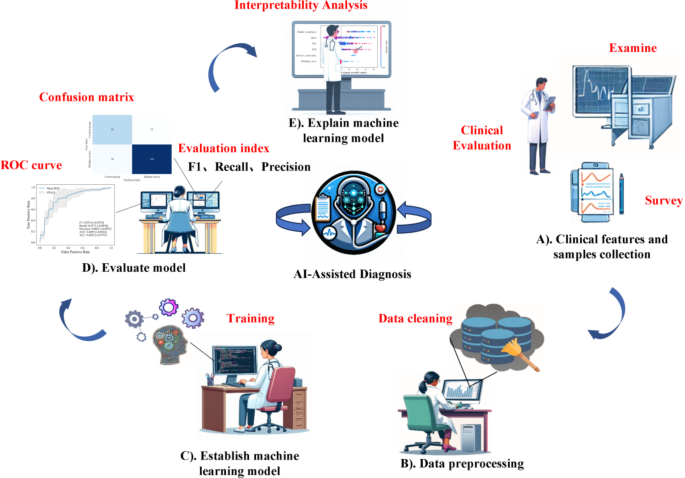
Bladder lesions are a common complication in patients with benign prostatic hyperplasia (BPH), a condition that leads to an enlarged prostate and lower urinary tract symptoms. These lesions, such as bladder diverticulum and bladder trabeculation, can develop due to the long-term effects of a blocked urine flow. If left untreated, bladder lesions can progress and lead to serious complications, including acute urinary retention and even kidney failure.
Limitations of Current Diagnostic Methods
Traditionally, doctors have relied on several methods to diagnose bladder lesions, such as cystoscopy, computed tomography (CT), magnetic resonance imaging (MRI), and ultrasound. However, these approaches have their limitations. Cystoscopy, the gold standard, is an invasive procedure that can cause discomfort for patients. Imaging techniques like CT and MRI can be costly or require contrast agents that may have adverse effects. Ultrasound, while non-invasive, often has low resolution and relies heavily on the operator’s experience.
A Machine Learning-Based Approach
To address these challenges, researchers from China set out to develop a more convenient and effective way to diagnose bladder lesions. They used machine learning, a powerful tool for uncovering complex relationships between clinical factors and disease outcomes.
The researchers analyzed data from 253 BPH patients, some of whom had bladder lesions and others who did not. They collected various clinical risk factors, including urodynamic characteristics, prostate-specific antigen (PSA) levels, bladder condition, and lower urinary tract symptoms.
Identifying the Key Factors
Using machine learning algorithms, the researchers were able to identify the four most important clinical risk factors for diagnosing bladder lesions:
1. Bladder compliance: Patients with decreased bladder compliance, indicating a stiff bladder wall, were more likely to have bladder lesions.
2. Maximum flow rate (Qmax): A lower Qmax, which reflects the obstruction of urine flow, was associated with a higher likelihood of bladder lesions.
3. Prostate-specific antigen (PSA): Higher PSA levels, often indicating prostate inflammation or enlargement, were linked to a greater risk of bladder lesions.
4. Post-void residual (PVR) urine: Patients with higher PVR, meaning more urine remained in the bladder after urination, were more prone to developing bladder lesions.
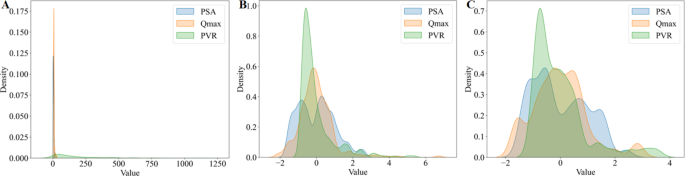
Potential Clinical Applications
The proposed machine learning-based model achieved high accuracy in diagnosing bladder lesions using these four key clinical risk factors. Unlike the current invasive or costly diagnostic methods, this approach relies on non-invasive, easily obtained measurements, making it a promising tool for routine screening of bladder lesions in BPH patients.
By identifying the most important factors contributing to the diagnosis, the researchers have provided valuable insights for clinicians. This information can help doctors better understand the relationship between these clinical factors and bladder lesions, enabling them to make more informed decisions and provide timely interventions to prevent the progression of these lesions and improve the quality of life for BPH patients.
Overall, this study demonstrates the potential of machine learning to revolutionize the diagnosis and management of bladder lesions, a common yet serious complication of BPH. By leveraging easily accessible clinical data, this non-invasive approach could pave the way for more effective and convenient bladder lesion screening, leading to earlier detection and improved patient outcomes.
Author credit: This article is based on research by Yunxin Wang, Jiachuang Li, Yunfeng Song, Hongguo Wei, Zejun Yan, Shuo Chen, Zhe Zhang.
For More Related Articles Click Here