Researchers have developed a novel approach using machine learning (ML) algorithms to evaluate the ecological status of surface waters in Poland that are not regularly monitored. This exciting breakthrough could significantly enhance water management and conservation efforts, helping to achieve the environmental goals set by the European Union’s Water Framework Directive (WFD). The study demonstrates the high efficiency of ML techniques, such as XGBoost and Random Forest, in accurately classifying the status of unmonitored water bodies, even when limited data is available. This innovative application of AI technology promises to revolutionize how we assess and protect our precious water resources. Machine learning, water quality, water management
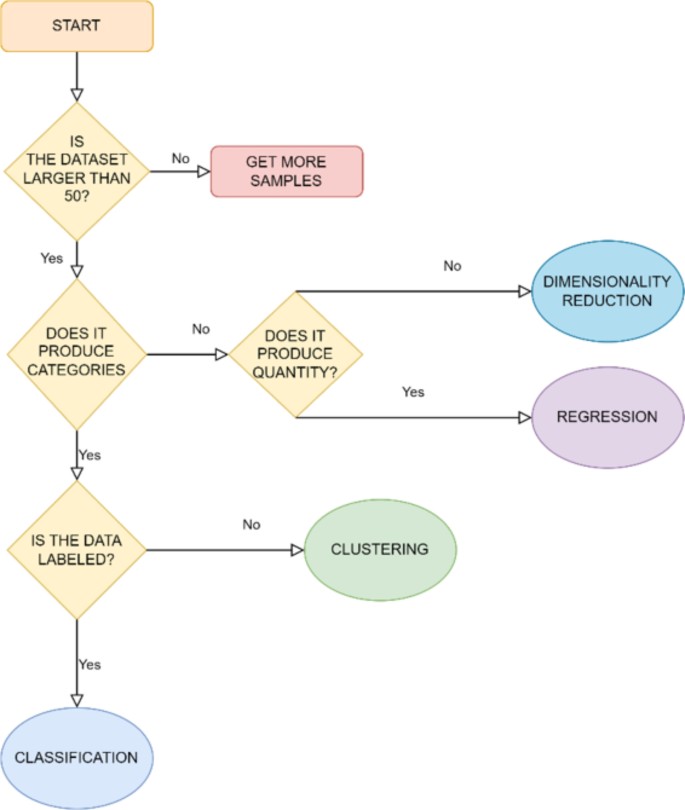
Unlocking the Power of Machine Learning for Water Quality Assessment
In a groundbreaking study, researchers from the Warsaw University of Technology and the Institute of Environmental Protection – National Research Institute have demonstrated the remarkable potential of machine learning (ML) algorithms in evaluating the ecological status of unmonitored water bodies in Poland. This innovative approach presents a significant advancement in the field of environmental protection, offering a cost-effective and efficient alternative to traditional water quality monitoring methods.
Addressing the Challenges of Comprehensive Water Monitoring
The European Union’s Water Framework Directive (WFD) mandates that all member states, including Poland, assess the ecological status of their water bodies and report the findings every six years. However, due to the resource-intensive nature of comprehensive water monitoring, it is often not feasible to assess the status of all designated water bodies solely based on actual measurement data.
This is where the power of machine learning comes into play. By utilizing an array of classification algorithms, including XGBoost and Random Forest, the researchers were able to accurately assess the ecological status of unmonitored water bodies based on available data on anthropogenic pressures within the catchment areas.
Achieving High Accuracy with Machine Learning Models
The study’s findings are particularly impressive, with the binary classification model (assessing water bodies as either “at least good” or “below good” status) achieving an accuracy of up to 93%. Furthermore, the researchers found that the XGBoost and Random Forest algorithms consistently outperformed other ML techniques, demonstrating their suitability for this specific application.
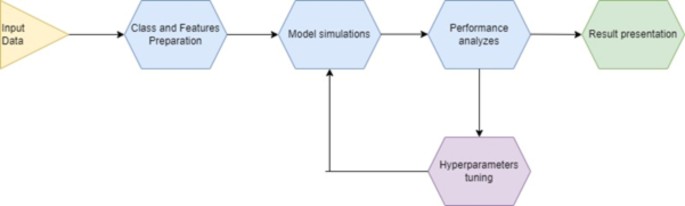
Ensuring Reliable Water Management Decisions
The high accuracy and low probability of misclassification achieved by the ML models provide water managers with a reliable tool for assessing the ecological status of unmonitored water bodies. This information is crucial for making informed decisions regarding water resource protection and sustainable management, as the WFD’s environmental objectives hinge on accurately identifying water bodies that fail to meet the “good status” threshold.
Paving the Way for a Sustainable Future
The successful application of machine learning in this context represents a significant step forward in the field of environmental protection. By leveraging the power of AI technology, researchers have developed a cost-effective and efficient method for evaluating the ecological status of water bodies that would otherwise go unmonitored. This breakthrough promises to enhance water management practices and contribute to the achievement of the WFD’s environmental goals, ultimately leading to a more sustainable future for Poland’s water resources.
Author credit: This article is based on research by Andrzej Martyszunis, Małgorzata Loga, Karol Przeździecki.
For More Related Articles Click Here