Researchers have developed a cutting-edge approach that combines reinforcement learning and fuzzy logic to identify the best nanomaterials for efficient energy harvesting, especially in dynamic road-based conditions. This innovative technique not only maximizes energy output but also takes into account crucial factors like durability, cost, and environmental impact. By leveraging the strengths of these advanced methods, the researchers have paved the way for a more sustainable and resilient future in renewable energy technologies.
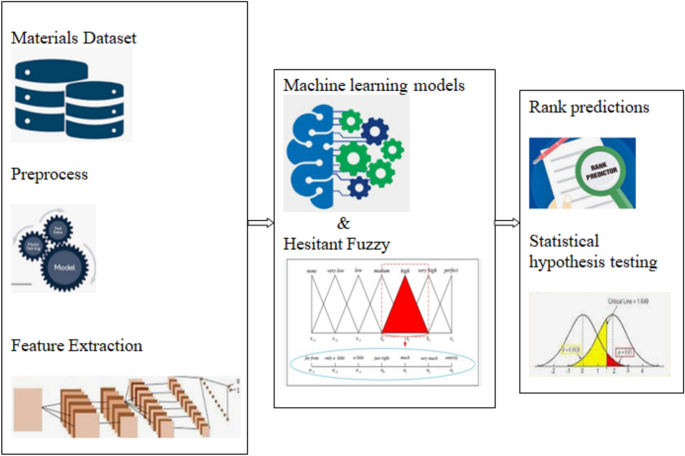
Navigating the Complexities of Nanomaterials for Energy Harvesting
Energy harvesting technologies based on nanomaterials have been gaining significant attention in recent years, as they hold the promise of improving the efficiency and sustainability of renewable energy systems. However, the selection of the right nanomaterial for a specific application can be a complex and challenging task, considering the various factors that need to be balanced.
Combining Reinforcement Learning and Fuzzy Logic for Optimal Material Selection
To address this challenge, researchers have developed a hybrid approach that integrates Reinforcement Deep Q-Network (DQN) and Fuzzy PROMETHEE, a multi-criteria decision-making method. The DQN algorithm enables the system to learn from its experiences in dynamic traffic environments, allowing it to select materials that deliver the highest energy output. Meanwhile, the Fuzzy PROMETHEE component incorporates the qualitative preferences and expertise of human experts, going beyond the purely quantitative data learned by the DQN.
Maximizing Energy Efficiency, Durability, and Sustainability
The key innovation of this approach is its ability to optimize for multiple, sometimes conflicting, criteria. By considering not only energy efficiency but also factors like durability, cost, and environmental impact, the researchers have developed a comprehensive framework for selecting the most suitable nanomaterials for energy harvesting applications. This holistic approach ensures that the chosen materials not only perform well technically but also align with the broader goals of sustainability and environmental stewardship.
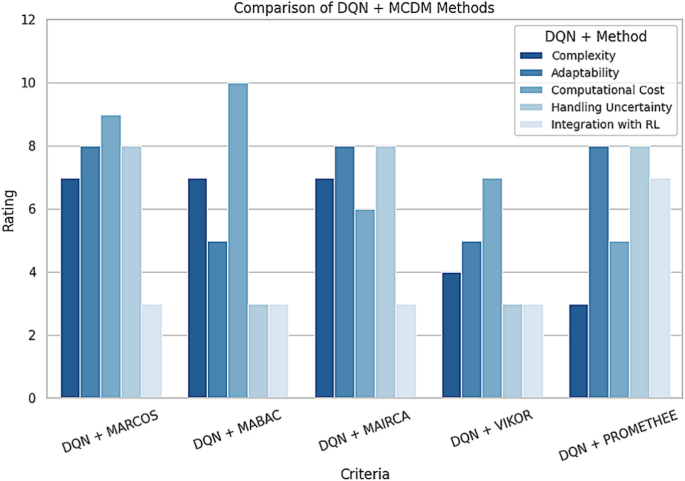
Navigating Real-World Challenges with Resilience
One of the standout features of this research is its focus on real-world scenarios, particularly the dynamic conditions found in road-based environments. By training the DQN model to adapt to changing traffic conditions, the researchers have created a system that can reliably operate in the face of fluctuating and unpredictable circumstances. This resilience is crucial for the successful deployment of energy harvesting technologies in practical applications.
Towards a Sustainable Energy Future
The findings of this study hold significant implications for the future of renewable energy technologies. By identifying the most suitable nanomaterials for energy harvesting, the researchers have paved the way for the development of more efficient and sustainable systems. This work not only contributes to the advancement of renewable energy but also highlights the potential of integrating cutting-edge machine learning and decision-making techniques to tackle complex environmental and technological challenges.
As the world continues to strive for a more sustainable future, the insights gained from this research offer a promising path forward, empowering researchers and policymakers to make informed decisions and drive the adoption of innovative energy solutions.
Author credit: This article is based on research by Sekar Kidambi Raju, Ganesh Karthikeyan Varadarajan, Amal H. Alharbi, Subhash Kannan, Doaa Sami Khafaga, Raj Anand Sundaramoorthy, Marwa M. Eid, S. K. Towfek.
For More Related Articles Click Here