Researchers have developed a groundbreaking computational algorithm that can accurately classify individuals into distinct “lifemetabotypes” based on their health, lifestyle, and metabolic traits. This innovative approach, described in a study published in the journal Scientific Reports, holds immense potential for personalized chronic disease prevention and management. By integrating data on factors like diet, physical activity, sleep, and mental well-being, the algorithm enables the identification of unique health profiles within a population, paving the way for tailored interventions and targeted public health strategies.
Unraveling the Complexities of Health and Lifestyle
Our health and well-being are profoundly shaped by a complex interplay of factors, from our dietary choices and physical activity levels to our genetic predispositions and socioeconomic status. Understanding these intricate relationships is crucial for developing effective strategies to prevent and manage chronic diseases, which have become a growing global health concern.
The Power of Clustering and Machine Learning
The research team, led by scientists from the IMDEA Food Institute in Spain, utilized a combination of statistical methods and machine learning algorithms to analyze data from the NUTRiMDEA online cohort, which included over 17,000 participants. By applying exploratory factor analysis and hierarchical clustering techniques, the researchers were able to identify five distinct “lifemetabotypes” within the population, each characterized by unique patterns of metabolic, lifestyle, and personal traits.
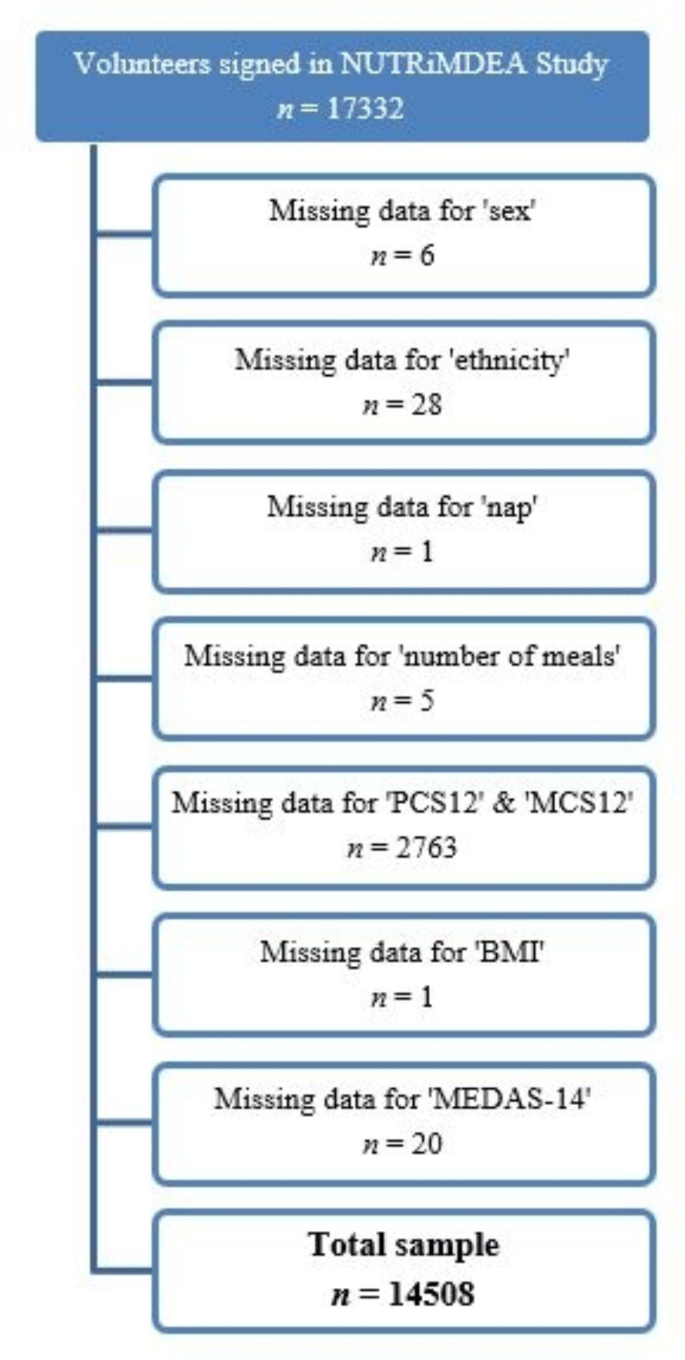
Unveiling the Lifemetabotypes
The five lifemetabotypes identified in the study include:
1. Westernized Millennial: Healthy young individuals with fair lifestyle habits.
2. Healthy: Healthy adults with generally positive health and lifestyle profiles.
3. Mediterranean Youth-Adult: Healthy young and middle-aged adults with a strong adherence to the Mediterranean diet.
4. Pre-morbid: Healthy adults with declining mental well-being.
5. Pro-morbid: Older individuals with poorer lifestyle habits, worse health, and lower health-related quality of life.
Developing a Computational Algorithm for Precision Health
The researchers then used a forward stepwise regression approach to identify the key variables that contributed the most to the classification of these lifemetabotypes. This information was then used to develop a computational algorithm that can quickly assign individuals to their respective lifemetabotype based on their responses to a set of 32 accessible questions.
Implications for Personalized Health and Public Health
The development of this computational algorithm represents a significant advancement in the field of precision health and personalized medicine. By accurately categorizing individuals based on their unique health and lifestyle profiles, healthcare providers and public health officials can now tailor interventions and preventive strategies to the specific needs of each lifemetabotype. This approach holds promise for enhancing chronic disease prevention, improving overall well-being, and supporting targeted public health initiatives.
Beyond the Horizon: Future Directions and Challenges
While this study showcases the immense potential of clustering and machine learning techniques in the realm of personalized health, the researchers acknowledge the need for further validation in diverse populations and the exploration of additional risk stratification methods. Additionally, the reliance on self-reported data, a common limitation of online surveys, highlights the importance of continued research to establish the reliability and validity of web-based data collection methods.
As the field of precision nutrition and personalized medicine continues to evolve, the insights gained from this study pave the way for a future where healthcare becomes increasingly tailored to the unique needs of each individual, ultimately leading to more effective prevention and management of chronic diseases.
Author credit: This article is based on research by Andrea Higuera-Gómez, Víctor de la O, Rodrigo San-Cristobal, Rosa Ribot-Rodríguez, Isabel Espinosa-Salinas, Alberto Dávalos, María P. Portillo, J. Alfredo Martínez.
For More Related Articles Click Here