Researchers have developed a groundbreaking artificial intelligence (AI) system that can detect early signs of retinal toxicity in patients undergoing treatment with chloroquine or hydroxychloroquine. These medications, commonly used for autoimmune diseases like systemic lupus erythematosus and rheumatoid arthritis, can unfortunately lead to vision-threatening retinal damage if not detected and treated early.

The new AI-powered approach analyzes multifocal electroretinogram (mfERG) data, capturing detailed electrical responses from different regions of the retina, to identify even the subtlest functional changes that could indicate the onset of this potentially devastating condition. By harnessing the power of machine learning, the researchers have developed models that outperform traditional analysis methods, paving the way for more accessible and effective screening for retinal toxicity. This breakthrough has significant implications for improving patient outcomes and ensuring safe, long-term use of these essential medications.
Protecting Vision: The Importance of Screening for Retinal Toxicity
Chloroquine and hydroxychloroquine are widely used drugs in the field of rheumatology, offering effective treatment for a range of autoimmune disorders. However, these medications can also pose a serious risk to the retina, the light-sensitive tissue at the back of the eye responsible for vision. Prolonged use of these drugs can lead to a condition known as toxic maculopathy, which can cause irreversible damage to the retina and result in severe visual impairment if not detected and addressed in a timely manner.
Unlocking the Power of Multifocal Electroretinography (mfERG)
To detect the early signs of retinal toxicity, clinicians often rely on a specialized diagnostic tool called electroretinography (ERG), which measures the electrical responses of the retina to light stimuli. The multifocal ERG (mfERG) technique, in particular, allows for the simultaneous recording of local ERG responses from multiple regions of the retina, providing a more detailed assessment of retinal function.
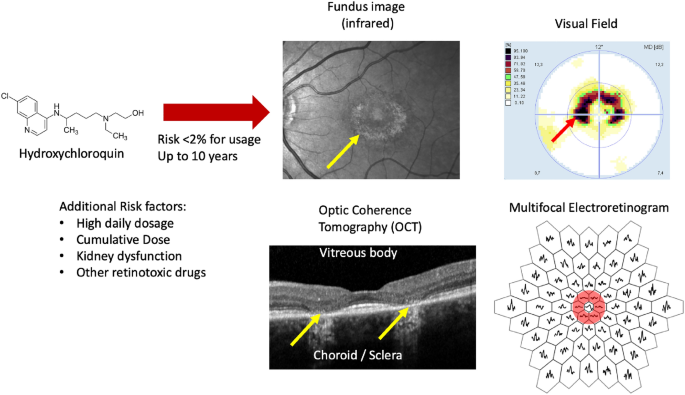
However, interpreting the complex mfERG waveforms requires specialized knowledge and can be a challenging task, even for experienced clinicians. This is where the power of artificial intelligence comes into play.
Harnessing the Power of AI for Improved Diagnosis
The research team, led by Mikhail Kulyabin, Jan Kremers, Vera Holbach, Andreas Maier, and Cord Huchzermeyer, set out to develop AI-powered models that could analyze the full mfERG traces, rather than relying solely on the standard amplitude and timing parameters. By utilizing advanced machine learning algorithms, such as Bidirectional Long Short-Term Memory (BiLSTM) and Time Series Transformer (TST), the researchers were able to uncover complex patterns and relationships within the mfERG data that were previously overlooked.
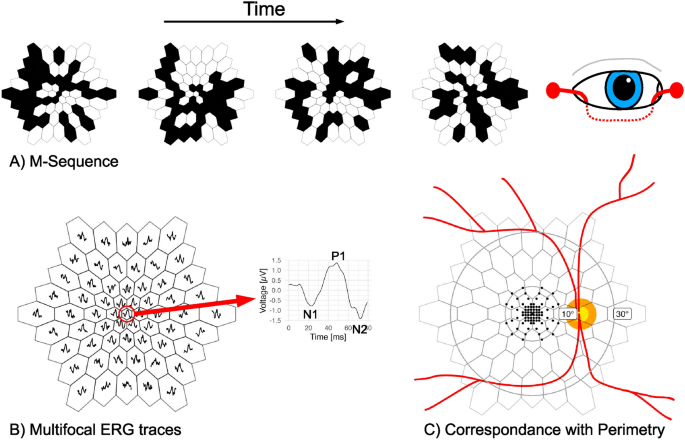
Table 1 Criteria for the OCT classification. Note that only the OCT information was used as a ground truth for the classification task.
The findings were remarkable. The AI-based models demonstrated superior predictive performance compared to traditional linear models, particularly in identifying the presence of toxic maculopathy. By leveraging the full mfERG traces, the AI algorithms were able to detect subtle functional changes that were not as evident when relying solely on the standard waveform parameters.
Predicting Visual Function with AI
In addition to the classification task of identifying toxic maculopathy, the researchers also explored the use of AI for predicting visual field sensitivities, a key indicator of retinal function. Here, the AI models again outperformed the traditional linear regression approaches, showcasing their ability to capture complex relationships between the mfERG data and the patient’s visual performance.
This finding suggests that the AI-powered analysis of mfERG data could not only enhance the detection of retinal toxicity but also provide valuable insights into the overall functional state of the retina, potentially aiding in the early identification of other retinal diseases.
Implications and Future Directions
The successful application of AI to mfERG analysis represents a significant step forward in the field of retinal health monitoring. By automating the interpretation of these complex electrophysiological signals, the researchers have paved the way for more widespread adoption of this screening tool, making it more accessible to clinicians and patients alike.
Moreover, the potential to identify early functional changes before the onset of irreversible structural damage could lead to more timely interventions and better patient outcomes. As the researchers continue to refine and expand their dataset, the AI models may even uncover previously unrecognized patterns in retinal function, opening up new avenues for understanding and managing a variety of retinal conditions.
In conclusion, this groundbreaking research demonstrates the transformative power of artificial intelligence in the realm of ophthalmology. By leveraging the full potential of mfERG data, the researchers have developed a game-changing approach to the detection and monitoring of retinal toxicity, ultimately empowering clinicians to provide more effective and personalized care for their patients.
Author credit: This article is based on research by Mikhail Kulyabin, Jan Kremers, Vera Holbach, Andreas Maier, Cord Huchzermeyer.
For More Related Articles Click Here