Researchers have developed an innovative approach to optimize the electrochemical reduction of carbon dioxide (CO2) using machine learning (ML) models. This breakthrough could pave the way for more efficient and sustainable carbon capture and utilization technologies.
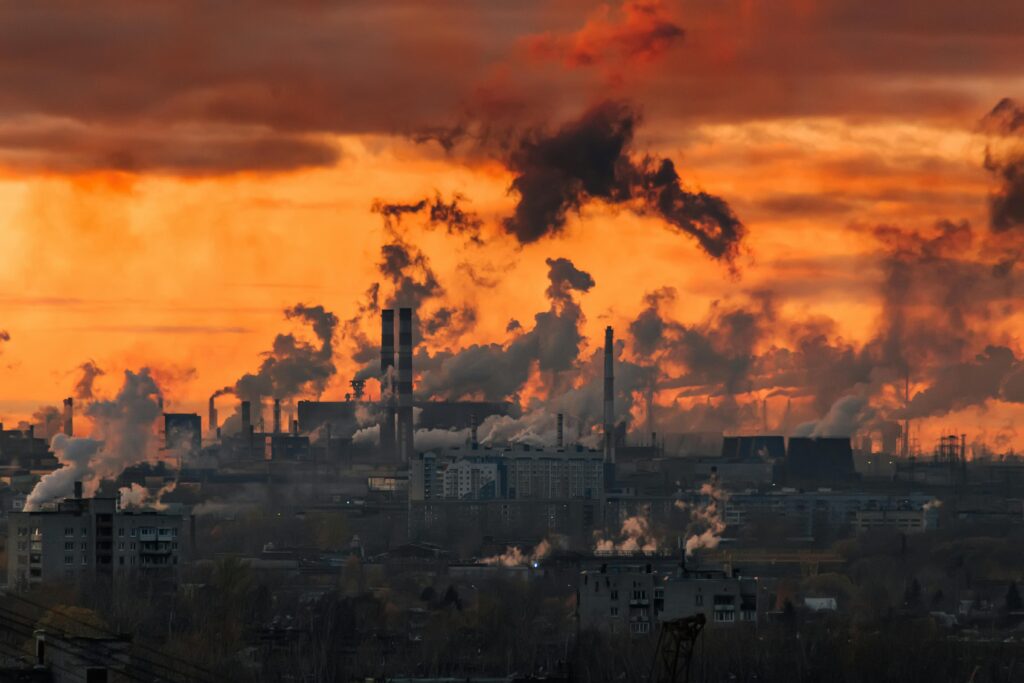
The study, led by a team from the University of Malaya, University of Riyadh, and RMIT University, focused on utilizing deep eutectic solvents (DES) as non-aqueous electrolytes to enhance the CO2 reduction reaction (CO2RR) process. By leveraging ML algorithms, the researchers were able to accurately predict the asymptotic current density, a crucial parameter for determining the maximum turnover frequency (TOF) of the CO2RR. This groundbreaking work not only advances our understanding of the complex CO2RR mechanisms but also provides a roadmap for optimizing the performance of future electrochemical systems. Carbon capture and utilization, Electrochemical CO2 reduction, and Deep eutectic solvents are key concepts highlighted in this research.
Harnessing the Power of Deep Eutectic Solvents for CO2 Reduction
The electrochemical reduction of CO2 has emerged as a promising approach for sustainable carbon capture and utilization. However, the process is often hindered by low current densities and the interference of undesired side reactions, such as the hydrogen evolution reaction (HER). To overcome these challenges, the research team explored the use of non-aqueous deep eutectic electrolytes, which have demonstrated enhanced CO2 solubility and the ability to suppress HER.
Deep eutectic solvents (DES) are a class of novel, environmentally friendly solvents composed of two or more components that form a liquid at room temperature due to hydrogen bonding interactions. These DES possess unique properties, including adjustable buffer capacities, that make them well-suited for electrochemical applications, particularly for the CO2RR process.
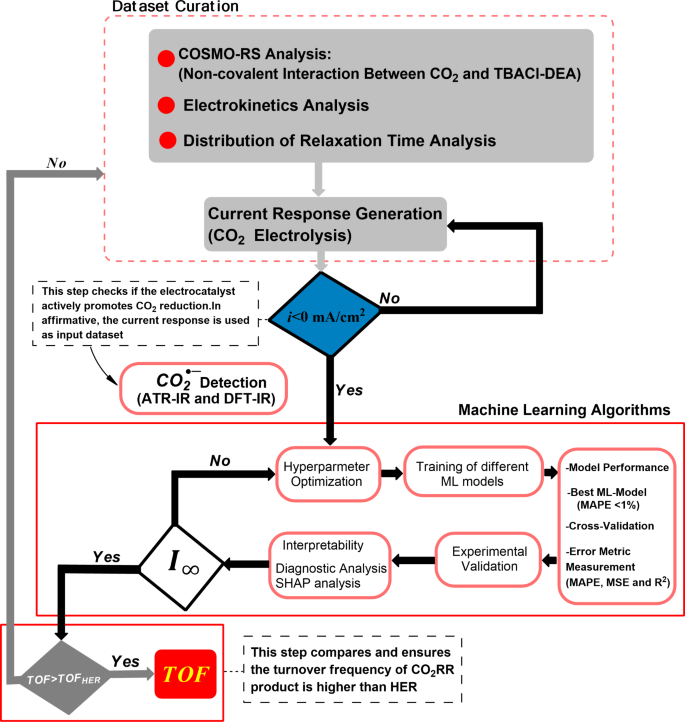
Leveraging Machine Learning for Optimal Performance
The key innovation of this study lies in the researchers’ use of machine learning (ML) techniques to predict the asymptotic current density of the CO2RR process in DES. By analyzing a comprehensive dataset of over 10,000 current density profiles, the team trained and compared four different ML models: Bagging, Gradient Boosting, Random Forest, and K-nearest Neighbors.
Through rigorous optimization, validation, and interpretability analyses, the researchers found that the Gradient Boosting model demonstrated the most stable and accurate performance in predicting the asymptotic current density. This allowed them to calculate the corresponding TOF, a crucial metric for evaluating the efficiency of the CO2RR process.
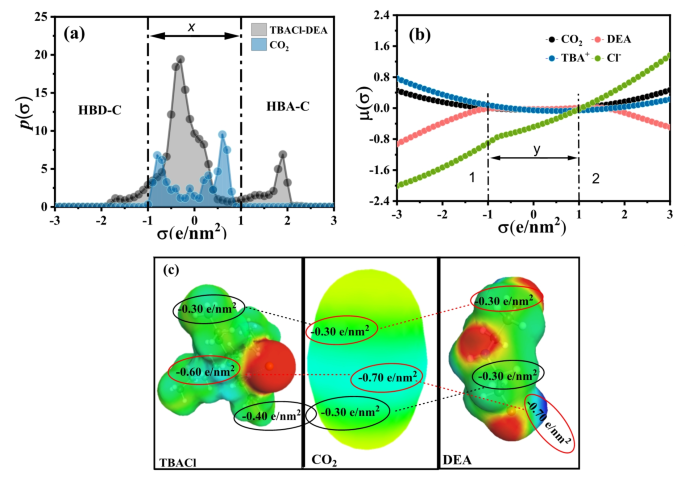
Fig. 2
Unraveling the Electrochemical Mechanisms
The researchers conducted a detailed investigation into the electrochemical mechanisms underlying the CO2RR in DES. They found that the process is non-adiabatic, meaning that the time required for nuclear motion of the reaction products (e.g., CO2•- and K2CO3) is shorter than the charge transfer relaxation time. This observation suggests that the rate-limiting step in the CO2RR is the association of CO2 with the DES, rather than the electron transfer step.
Further analysis using techniques like Tafel slope analysis and distribution of relaxation times (DRT) provided insights into the reaction regime and the influence of various factors, such as the electrode material, on the CO2RR performance. These findings highlight the importance of understanding the complex interplay between the electrolyte, electrode, and reaction intermediates in optimizing electrochemical systems.
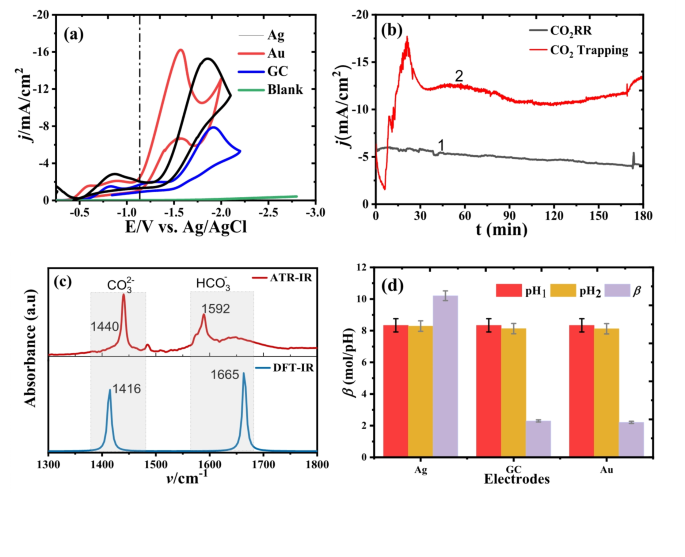
Fig. 3
Unlocking the Potential of CO2 Conversion
The results of this study demonstrate the effectiveness of using machine learning to predict and optimize the performance of electrochemical CO2 reduction processes. By accurately determining the asymptotic current density and TOF, the researchers have provided a roadmap for scaling up CO2RR technologies and achieving industrially relevant current densities.
Furthermore, the insights gained into the electrochemical mechanisms can inform the rational design of more efficient electrocatalysts and electrolyte systems. This could lead to significant advancements in the field of carbon capture and utilization, contributing to a more sustainable future.
Paving the Way for a Greener Future
This research represents a significant step forward in the quest to develop efficient and scalable CO2 conversion technologies. By combining the unique properties of deep eutectic solvents with the predictive power of machine learning, the researchers have opened up new avenues for optimizing electrochemical systems and advancing the practical application of CO2 reduction.
As the world grapples with the pressing challenge of climate change, innovations like this hold the promise of transforming carbon dioxide from a problematic greenhouse gas into a valuable resource for the production of fuels, chemicals, and other valuable products. The potential impact of this work extends beyond the scientific community, contributing to a more sustainable and environmentally conscious future for all.
This article is based on research by Ahmed Halilu, Mohamed Kamel Hadj-Kali, Hanee Farzana Hizaddin, Mohd Ali Hashim, Emad M. Ali, Suresh Bhargava.
For More Related Articles Click Here