Parkinson’s disease is a complex and debilitating neurological disorder that affects millions of people worldwide. Researchers from Korea University have developed a groundbreaking approach to managing Parkinson’s medication that takes into account the dynamic nature of the disease’s progression. By using a novel time-series clustering technique, the team identified four distinct patterns of symptom progression, ranging from a desirable “fair state” to a deteriorating “severe state.” Armed with this comprehensive understanding of the disease’s trajectory, the researchers then constructed a Markov Decision Process (MDP) model to provide personalized medication recommendations. This dynamic framework not only suggests the optimal treatment strategy to maintain a favorable health state but also warns against high-risk medication adjustments that could accelerate disease worsening. This innovative approach represents a significant advancement in the field of Parkinson’s disease management, offering clinicians a data-driven tool to optimize patient outcomes over time. Parkinson’s disease, Levodopa, Markov Decision Process, Medication Management
Unraveling the Complexities of Parkinson’s Disease
Parkinson’s disease is a chronic and progressive neurological disorder that affects millions of people worldwide. It is characterized by a range of motor and non-motor symptoms, including tremors, muscle stiffness, slow movement, and cognitive impairment. The exact cause of Parkinson’s disease is still not fully understood, but it is known to involve the degeneration of dopamine-producing neurons in the brain.
Parkinson’s disease is a challenging condition to manage, as its symptoms can fluctuate over time and the effectiveness of medications can diminish. While initial treatment selection is well-established, guidelines for long-term medication adjustment strategies to optimize symptom control and minimize adverse effects are limited. This is where the groundbreaking research from Korea University comes into play.
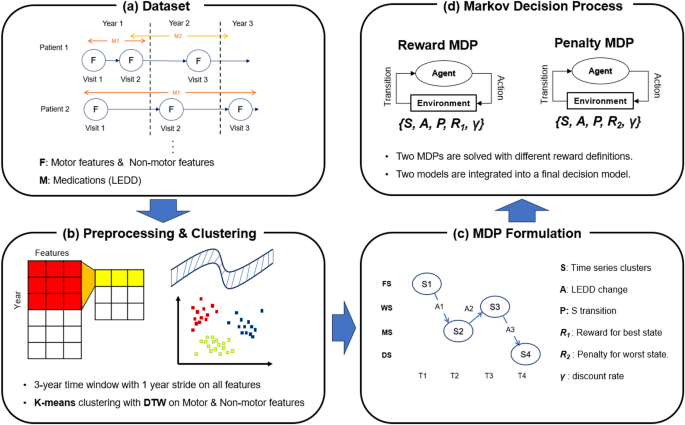
A Novel Approach to Parkinson’s Medication Management
The researchers from Korea University developed a comprehensive framework that combines time-series analysis and Markov Decision Process (MDP) modeling to provide personalized medication recommendations for Parkinson’s patients. The key to their approach lies in the way they capture the dynamic nature of the disease’s progression.
Using data from the Parkinson’s Progression Markers Initiative (PPMI), the researchers employed a multivariate time-series clustering technique to identify four distinct patterns of symptom progression:
1. Fair State (FS): Patients in this cluster exhibit improvement or maintain their status across various clinical assessments, including motor and non-motor symptoms.
2. Worsening State (WS): Patients in this cluster show mild deterioration in both motor and non-motor domains.
3. Motor-focused Worsening State (MS): Patients in this cluster experience significant worsening in motor-related symptoms.
4. Deteriorating State (DS): Patients in this cluster exhibit a general decline across all clinical features.
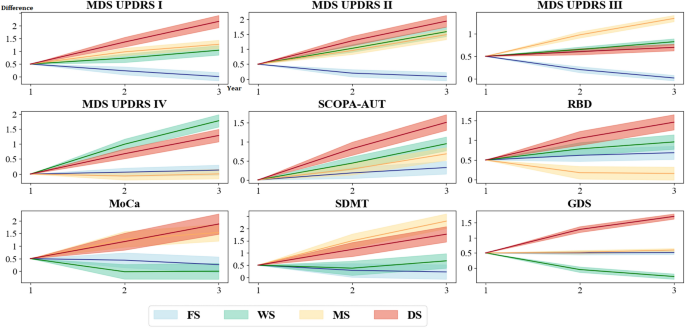
Table 1 Statistical data, including the mean, standard deviation (Std), first quartile (Q1), and third quartile (Q3), is provided for each clinical feature.
By defining these distinct patient states, the researchers were able to construct two separate MDP models: one aimed at maximizing the time spent in the desirable FS state, and the other focused on minimizing the time spent in the undesirable DS state. The key to their approach was the incorporation of Levodopa Equivalent Daily Dose (LEDD) as a representation of medication intensity, rather than just considering the types of medications.
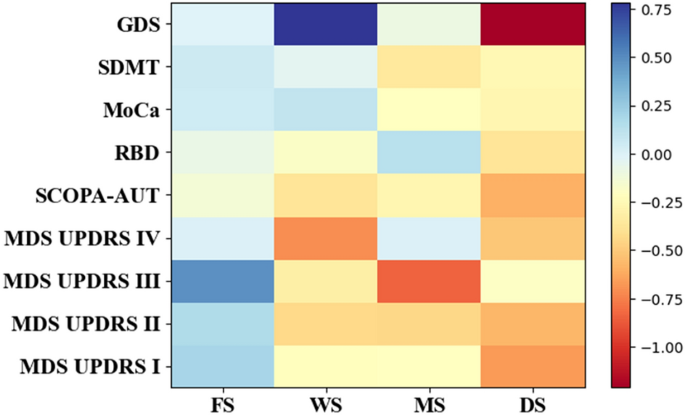
Figure 2
Optimizing Medication Strategies for Parkinson’s Patients
The MDP models developed by the researchers provide valuable insights into the optimal and high-risk medication adjustment strategies for Parkinson’s patients. The optimal policy, derived from the Reward MDP model, suggests that higher LEDD is more effective for maintaining a desirable health state, while the Penalty MDP model’s worst policy warns against increasing medication intensity for patients in the worsening or deteriorating states.
This comprehensive framework offers several advantages over previous approaches:
1. Dynamic Representation of Disease Progression: By using time-series clustering, the researchers were able to capture the complex and evolving nature of Parkinson’s symptoms, going beyond the traditional static assessment of disease stages.
2. Personalized Medication Recommendations: The MDP models provide tailored guidance on medication intensity adjustments, rather than prescribing specific medication types, making the approach more flexible and applicable in clinical practice.
3. Identification of High-Risk Actions: The integration of the Reward and Penalty MDP models allows clinicians to not only identify the optimal medication strategy but also be aware of the high-risk actions to avoid, further enhancing patient safety.
Implications and Future Directions
The research from Korea University represents a significant advancement in the field of Parkinson’s disease management. By leveraging the power of data-driven techniques, the researchers have developed a framework that can help clinicians optimize medication strategies and improve patient outcomes over the long term.
This innovative approach has the potential to be expanded and adapted to other chronic, progressive diseases, where personalized medication management is crucial. Furthermore, the incorporation of additional data sources, such as genetic information or adverse event data, could further enhance the precision and effectiveness of the medication recommendations.
As the scientific community continues to unravel the complexities of Parkinson’s disease, research like this offers hope for a future where patients can navigate the challenges of this condition with greater confidence and improved quality of life.
Author credit: This article is based on research by Minjae Oh, Hongbum Kim, Hyo Kyung Lee.
For More Related Articles Click Here