Researchers have developed advanced machine learning models to predict the success rate of clinical pregnancy in assisted reproductive techniques (ART) like in-vitro fertilization (IVF) and intrauterine insemination (IUI). By analyzing sperm quality parameters such as morphology, motility, and count, these models can provide valuable insights to help improve fertility outcomes for couples struggling with infertility. The study highlights the potential of integrating cutting-edge AI techniques with traditional sperm assessments to elevate the precision and personalization of clinical decision-making in the field of ART. Assisted reproductive technology and infertility are complex issues that affect many couples worldwide.
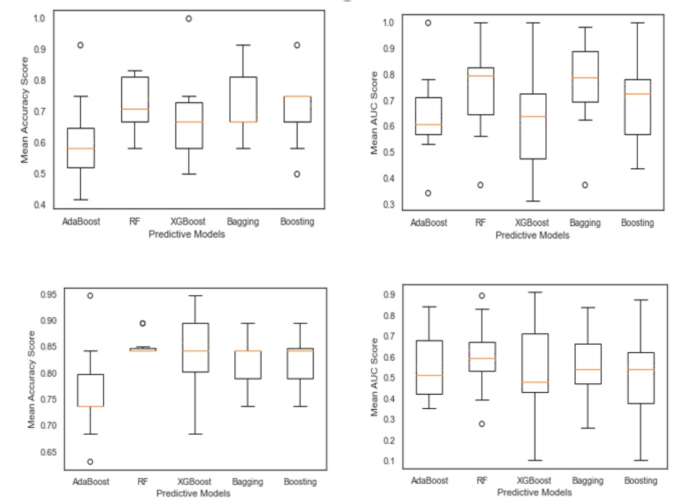
Ensemble Models Outperform Traditional Approaches
The researchers utilized various ensemble machine learning models, including Bagging, Boosting, and Random Forest, to predict the success rate of clinical pregnancy based on sperm quality parameters. Among the models evaluated, the Random Forest algorithm achieved the highest accuracy (72%) and area under the receiver operating characteristic curve (80%) in predicting pregnancy outcomes for both IVF/ICSI and IUI procedures.
Sperm Quality Parameters Impact Pregnancy Outcomes Differently
The study found that the influence of sperm quality parameters on pregnancy success varied between the IVF/ICSI and IUI procedures. For IUI cycles, all three sperm parameters (morphology, motility, and count) had significant negative impacts on the prediction of clinical pregnancy success. In contrast, for IVF/ICSI cycles, sperm motility had a positive effect, while sperm morphology and count were negative factors.
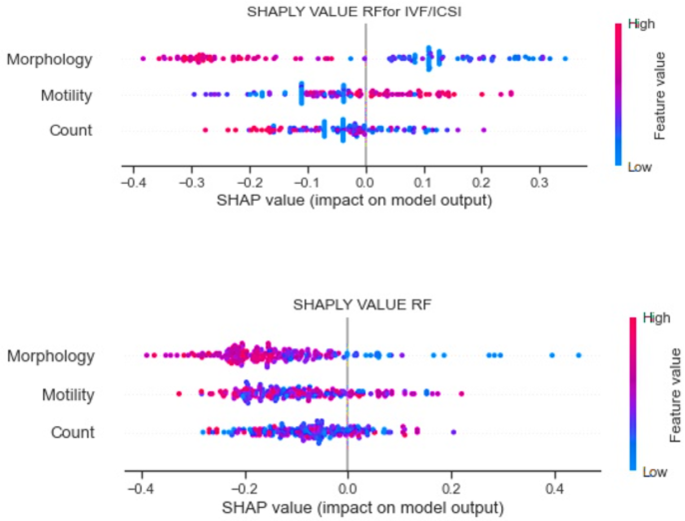
Identifying Critical Thresholds for Sperm Parameters
The researchers also determined optimal cut-off values for sperm parameters that could serve as evidence-based decision rules for clinicians. For example, the study identified a sperm count threshold of 54 million per ml for IVF/ICSI and 35 million per ml for IUI procedures. Additionally, a significant cut-off point of 30 million per ml was found for the sperm morphology parameter across all ART procedures.
Personalized Approach to Improving Fertility Outcomes
This study highlights the potential of leveraging advanced machine learning models in combination with traditional sperm quality assessments to enhance the precision and personalization of clinical decision-making in the field of assisted reproductive technologies. By integrating these innovative approaches, clinicians can better understand the complex interplay between sperm parameters and pregnancy success, ultimately offering more hope and better outcomes for couples struggling with infertility.
Author credit: This article is based on research by Ameneh Mehrjerd, Toktam Dehghani, Mahdie Jajroudi, Saeid Eslami, Hassan Rezaei, Nayyereh Khadem Ghaebi.
For More Related Articles Click Here