Researchers have developed an innovative approach to classify air pollution levels with unprecedented accuracy. By integrating support vector machines (SVMs) optimized through differential gravitational fireworks algorithms, this new method outperforms traditional techniques and offers a promising solution to the ongoing challenge of air quality assessment. The study’s findings hold significant implications for environmental conservation and urban planning, paving the way for more effective monitoring and control of air pollution. Support vector machines and optimization algorithms are at the heart of this groundbreaking research.
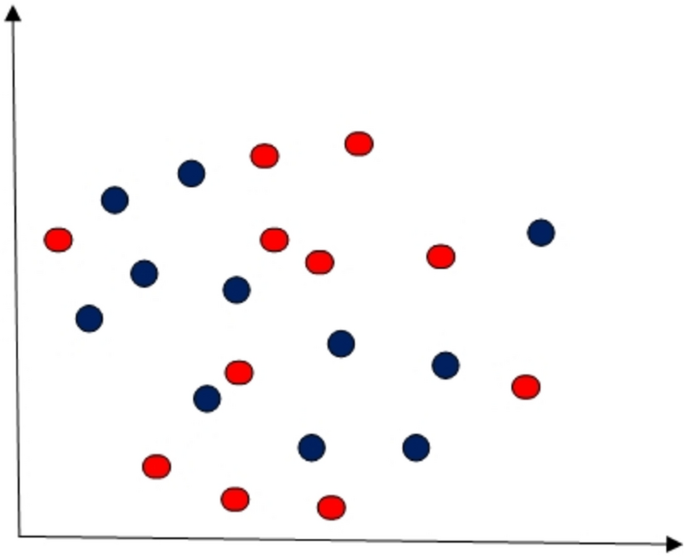
Tackling the Air Pollution Crisis with Cutting-Edge Technology
As the world grapples with escalating environmental concerns, the need for accurate and efficient air quality assessment has never been more pressing. Researchers have now unveiled a game-changing approach that combines advanced machine learning algorithms and optimization techniques to revolutionize the way we monitor and classify air pollution.
At the core of this innovative solution is the integration of support vector machines (SVMs) with a novel differential gravitational fireworks optimization (DGFO) algorithm. SVMs are a powerful class of machine learning models known for their ability to handle complex, nonlinear data patterns, making them well-suited for the intricate task of air quality analysis.
Unleashing the Power of Sequential Data Fusion
One of the key innovations of this research is the introduction of a sequential fusion classification technique. This method recognizes the inherent continuity and interdependencies within air pollution data, enabling the model to capture the nuanced relationships between consecutive data points. By incorporating this sequential approach, the researchers were able to achieve significantly higher classification accuracy compared to traditional non-sequential methods.
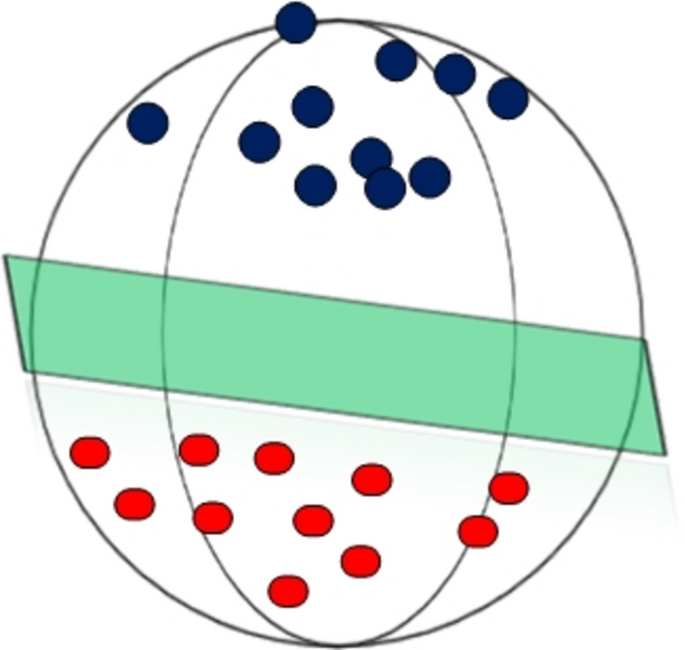
Optimizing SVM Performance with Differential Gravitational Fireworks
To further enhance the performance of the SVM model, the researchers integrated the differential gravitational fireworks optimization (DGFO) algorithm. This advanced optimization technique combines the strengths of differential evolution and gravitational search algorithms, providing precise tuning of the SVM’s crucial parameters, such as the penalty factor and kernel function. By optimizing these parameters, the researchers were able to significantly improve the model’s classification accuracy, mitigate the risks of overfitting and underfitting, and enhance its overall reliability.
Outperforming Conventional Approaches
Through rigorous testing and evaluation, the researchers demonstrated the superiority of their DGFO-SVM method over traditional SVM techniques, as well as other popular machine learning models like XGBoost and deep neural networks. The DGFO-SVM approach achieved an impressive classification accuracy of up to 91%, outperforming its counterparts by at least 3 percentage points.
Advancing Environmental Conservation and Urban Planning
The implications of this research extend far beyond the technical realm. By providing a more accurate and reliable method for air quality assessment, the DGFO-SVM framework has the potential to significantly impact environmental conservation and urban planning efforts. Accurate air pollution monitoring can inform policymakers, urban planners, and environmental agencies, enabling them to make more informed decisions and implement targeted strategies to improve air quality and safeguard public health.
As the world continues to grapple with the challenges of air pollution, this groundbreaking research offers a glimmer of hope. By leveraging the power of advanced machine learning and optimization techniques, researchers have developed a transformative solution that holds the promise of a cleaner, healthier future for all.
Author credit: This article is based on research by Bian Chao, Huang Guangqiu.
For More Related Articles Click Here