Researchers have developed a groundbreaking method called partCNV and partCNVH that can accurately identify cancer cells within a tumor sample using single-cell RNA sequencing (scRNA-seq) data. By incorporating information from routine clinical tests called cytogenetics, these new tools can pinpoint cells with specific chromosomal abnormalities that are hallmarks of cancer. This advancement could lead to earlier and more precise diagnoses, as well as better understanding of how cancer develops and progresses. The research has significant implications for improving cancer detection and treatment, particularly in blood cancers like myelodysplastic syndrome and acute myeloid leukemia.
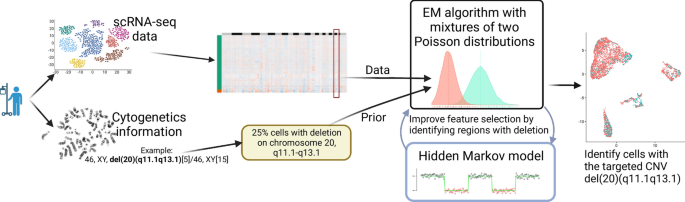
Unraveling Cancer’s Complexity with Single-Cell Technology
Single-cell RNA sequencing (scRNA-seq) has revolutionized our understanding of cancer by allowing researchers to analyze the genetic makeup and behavior of individual cells within a tumor. This powerful tool has revealed the remarkable diversity and complexity of cancer, as each patient’s tumor can harbor a unique collection of cell types, each with its own set of genetic mutations and gene expression patterns.
One of the key challenges in analyzing scRNA-seq data from cancer patients is accurately identifying the neoplastic cells – the abnormal, rapidly dividing cells that drive tumor growth and progression. These cancer cells can closely resemble normal cells, making them difficult to distinguish using standard techniques.
Incorporating Cytogenetic Information for Precise Cell Classification
To address this challenge, researchers have developed two new methods, called partCNV and partCNVH, that leverage additional information from a routine clinical test called cytogenetics. Cytogenetics analyzes the chromosomes of a patient’s cells, looking for specific structural changes or abnormalities that are often associated with cancer.
By incorporating the cytogenetic data into their statistical models, the researchers were able to more accurately identify the neoplastic cells within the scRNA-seq data. The partCNV method uses an expectation-maximization (EM) algorithm to classify cells as either normal or aneuploid (having an abnormal number of chromosomes), while partCNVH further refines the classification by using a hidden Markov model to select the genes most likely to be affected by chromosomal changes.
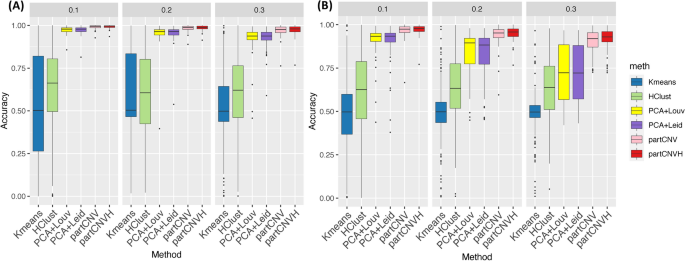
Validating the Accuracy and Robustness of the New Methods
The researchers thoroughly tested the performance of partCNV and partCNVH through extensive simulation studies and real-world data analysis. They found that the new methods significantly outperformed existing techniques, particularly when the chromosomal changes were relatively small or the signal was weak compared to the background noise.
Importantly, the researchers also demonstrated that the new methods were robust to variations in the prior information from the cytogenetic data, highlighting their flexibility and potential for broader application.
Unlocking Insights into Cancer Biology and Improving Patient Care
The success of partCNV and partCNVH in identifying neoplastic cells has important implications for cancer research and patient care. By accurately distinguishing cancer cells from normal cells, these methods can provide valuable insights into the biological processes and molecular pathways involved in cancer development and progression.
Furthermore, the ability to detect specific chromosomal abnormalities in individual cells could lead to earlier and more precise diagnoses, as well as better-tailored treatment strategies for cancer patients. This is particularly relevant for blood cancers like myelodysplastic syndrome and acute myeloid leukemia, where chromosomal changes play a crucial role in disease progression and prognosis.
As single-cell technologies continue to advance, the integration of cytogenetic data through methods like partCNV and partCNVH will be an essential tool for unraveling the complexities of cancer and improving patient outcomes.
Author credit: This article is based on research by Ziyi Li, Ruoxing Li, Irene Ganan-Gomez, Hussein A. Abbas, Guillermo Garcia-Manero, Wei Sun.
For More Related Articles Click Here