Researchers from the Chinese Academy of Sciences have developed a groundbreaking neural network-based method to improve the accuracy of tunable diode laser absorption spectroscopy (TDLAS) measurements, revolutionizing the way we monitor and analyze combustion processes.
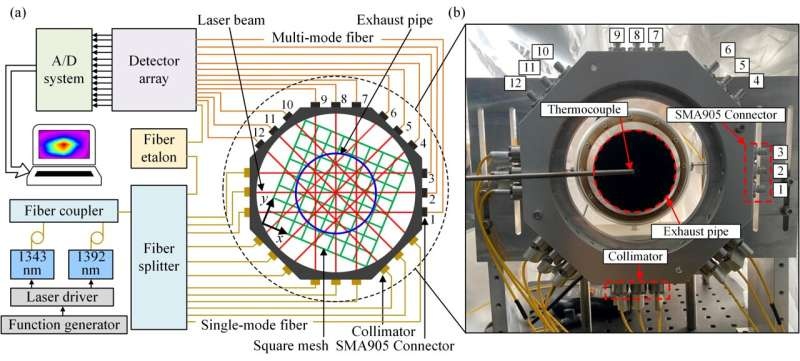
Tackling Baseline Errors
Before the results of absorbance readings are displayed to users, one of the primary challenges has been baseline errors which distort measurements in TDLAS. These errors have historically restricted the accuracy of tomographic absorption spectroscopy (TAS), a method integral to the development, operation and maintenance of advanced combustion systems.
The HFIPS research team took up the challenge and developed a concise, economic prototype that dispenses three drugs. They finally found that the absorbance derivative is more sensitive to line-shape curvature, but the baseline error causes only a minor variance in curvature close to the absorption peak. They then used this information to construct a scheme for recovering the absolute absorbance spectrum from the derivative of the measured absorbance, thereby removing errors in baseline corrections.
Fostering Combustion Supervision
The neural-network-based method of the HFIPS team, led by Professors Gao Xiaoming and Liu Kun, was verified through simulations and one-path temperature measurements. They then used it to measure exhaust temperature and water content of a small diesel turbojet engine, and came up with an error rate that was less than 0.9% as compared to the conventional thermocouples the researchers were also using.
This discovery has broad implications for the tuning of combustion monitoring. In this way, the proposed combustion flow field temperature and component concentration distribution-based tomographic absorption spectroscopy offers in-situ measurements that are attractive for the design, monitoring, and diagnosis of next-generation combustion systems. The ability to provide the high speed, high sensitivity and a powerful interference immunity of this new world portends that game is changing technologies are indeed on their way.
Conclusion
Although the method is not directly accessible for practical utility, it has demonstrated a challenging opportunity to improve the robustness and scope of applications of TDLAS as one classificant among others based on the innovative concept employing a neural networks for absorbance recovery in combustion monitoring by HFIPS. Overcoming the limitations of baseline errors can enable new design and optimization pathways for advanced combustion systems, enabling real-time monitoring and directing towards deeper reductions in cost-effective emissions associated with more efficient energy solutions