Scientists have developed a remarkable deep learning technique that can accurately detect and classify pests infesting crop leaves. This innovative approach, called regional convolutional neural network (R-CNN), combines advanced computer vision algorithms with a novel hierarchical masking method to identify pest-affected regions on leaves with unprecedented precision. By leveraging the power of deep learning, this technology promises to revolutionize the way farmers and agricultural experts monitor and manage crop pests, ultimately leading to increased productivity and reduced crop losses.
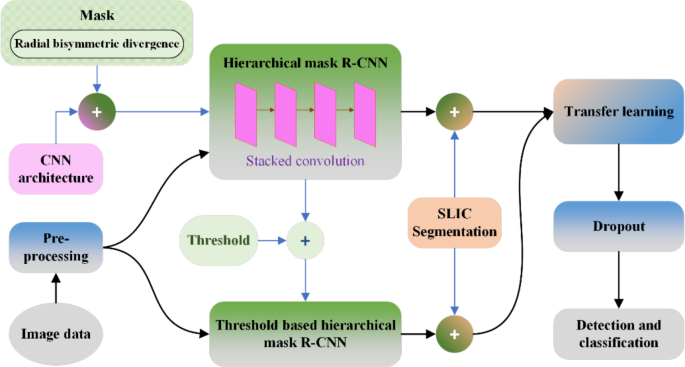
Empowering Precision Pest Control
Pests pose a significant threat to crop production, causing substantial economic losses and challenging the global food supply. Traditional pest management techniques, such as routine pesticide spraying, are often ineffective and can have detrimental environmental consequences. The need for a more sustainable and efficient solution has led researchers to explore the potential of advanced technologies, particularly in the field of computer vision and deep learning.
The study presents a novel image segmentation approach that utilizes the power of R-CNN architecture, combined with a innovative radial bisymmetric divergence (RBD) method for enhanced efficiency in pest detection. This method, dubbed the hierarchical mask R-CNN (HM-R-CNN), effectively segments the leaf images to identify regions where pests may be present.
Overcoming Challenges with Threshold-Based Improvements
One of the key challenges in pest detection is dealing with inaccurately labeled data, which can lead to flawed learning and diminish the efficacy of pest identification methods. To address this issue, the researchers introduced the threshold-based hierarchical mask R-CNN (TbHM-R-CNN) architecture. This innovative approach incorporates a fault-tolerant mechanism, allowing the model to adapt to various complex situations of pest variations and maintain a high level of accuracy.
Unparalleled Precision and Efficiency
The results of the study are truly impressive. The TbHM-R-CNN model achieved a classification accuracy of 96.2%, a recall of 97.5%, and an F1 score of 0.982, outperforming conventional techniques and other deep learning methods. This level of precision and efficiency is a game-changer in the field of crop protection, enabling farmers and agricultural experts to quickly and accurately identify pest infestations, allowing for targeted and timely interventions.
Revolutionizing Digital Agriculture
The development of this AI-powered pest detection system represents a significant step forward in the realm of Click Here