Researchers have developed a novel solution to enhance the capabilities of light-sheet fluorescence microscopy, using a deep learning-powered phase mask to create unexpected beam shapes that significantly improve image quality.
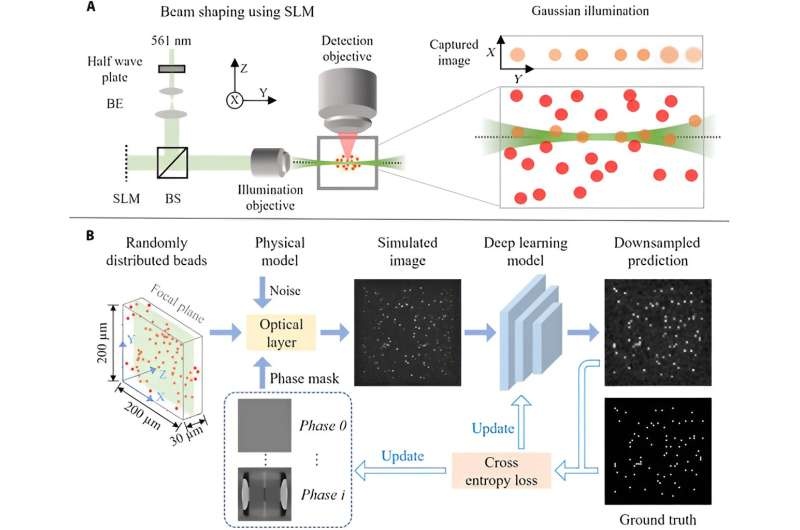
An Introduction to the Mathematics of Deep Learning
Conventional tools can be complicated, requiring a high level of expertise and can take long time. Yet, the researchers have come with an innovative technique using deep learning to automatize this step.
However, the team combined optics propagation modeling with a deep neural network for simultaneous optimization of the parameters of the deep learning network and an illumination beam. This enables direct contrast and image quality optimization, without the need for complex optical design software.
The real trick is that the deep learning model creates odd beams, like the butterfly beam shown above, it really innovates on what shapes a be should take to get better results. We train the model to sweep a wide range of parameters and determine the combination that best optimizes over hundreds of thousands of variables in the phase mask, appropriately including for example thermal constraints.
Changing The Face Of Light-Sheet Microscopy
This method is now considered to be one of the most promising tools for imaging large, tissue-cleared samples in 3D in recent years due to properties such as optical sectioning, low photodamage and fast acquisition. Nevertheless, the image is highly related to its illumination beam.
While recent achievements like spinning Bessel, reoriented Airy, or sheet-like lattice light-sheets have rendered impressive performances in uniform and high-contrast imaging. However, the researchers say that there may still be some room for development and their new method is the one which will lead to enhanced performance.
By enabling the generation of atypical, unconventional beam shapes — essentially allowing any kind of structured illumination that you want to reproduce at a sample plane where it is traditionally not considered possible — the deep learning-powered phase mask has a clear capability to lead to major improvements in image quality in biological samples and should take care of one essential gap in light-sheet based fluorescence microscopy right now. In this way, researchers are not just analyzing images; they control the illumination beam for better performance — a quantum leap in perspective.
Conclusion
This phase mask for light-sheet fluorescence microscopy is an important contribution of deep learning automated approaches. Using deep learning to devise unconventional beam shapes, the researchers showed an avenue to greatly enhance image quality, streamline design and enable new capabilities in biological imaging. The technique could one day transform how light-sheet microscopy is performed and thus, potentially uncover new science in numerous areas.