Researchers have developed a revolutionary hybrid machine learning approach to accurately predict the drilling rate index (DRI) of rocks, a crucial factor in optimizing mining and construction projects. By integrating powerful algorithms like grey wolf optimization, support vector machines, random forests, and extreme gradient boosting, this innovative technique outperforms traditional methods, offering significant advancements for the industry. The study’s findings pave the way for more efficient and cost-effective rock excavation practices, benefiting industries ranging from mining to infrastructure development. Machine learning and optimization techniques are transforming the way we understand and utilize rock properties for various applications.
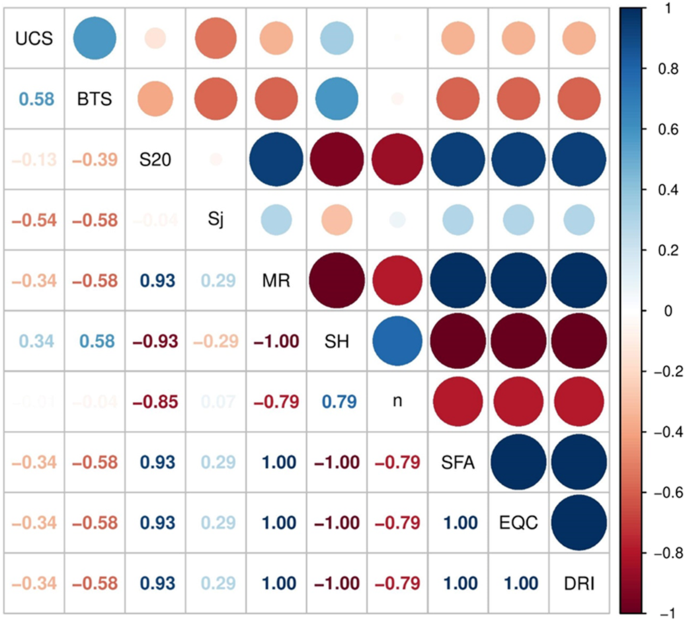
Unlocking the Secrets of Rock Drillability
The drilling rate index (DRI) is a critical parameter that informs the choice of appropriate drilling methods and equipment, ultimately improving the efficiency of rock excavation projects. However, accurately predicting DRI has long been a challenge for the industry. Traditional approaches often fall short, leading to suboptimal decision-making and reduced productivity.
A Hybrid Approach to Accurate DRI Prediction
In a groundbreaking study, researchers from the China University of Mining and Technology have developed a hybrid machine learning approach that revolutionizes DRI prediction. By integrating grey wolf optimization with support vector machines, random forests, and extreme gradient boosting, the team has achieved unprecedented accuracy in forecasting the DRI of various rock types, including sedimentary, metamorphic, and igneous varieties.
Outperforming Traditional Methods
The hybrid models demonstrated outstanding performance, with the GWO-XGBoost model emerging as the clear standout. This model achieved a remarkable coefficient of determination (R²) of 0.999, meaning it captured 99.9% of the variance in the target variable. Additionally, the model exhibited exceptionally low mean absolute error (MAE) and root mean square error (RMSE) values, further confirming its superior predictive capabilities.
Revolutionizing Rock Excavation Practices
The implications of this research are far-reaching. By providing a highly accurate and reliable tool for DRI prediction, the study aims to transform rock excavation practices across various industries, from mining to construction. Armed with this powerful hybrid approach, professionals can now make more informed decisions, optimize drilling operations, and enhance the overall efficiency of their projects.
Paving the Way for the Future
The success of this hybrid machine learning approach highlights the immense potential of integrating advanced algorithms and optimization techniques in the field of geotechnical engineering. As the industry continues to evolve, similar innovative solutions are expected to emerge, further streamlining rock excavation processes and driving progress in the exploration and utilization of Earth’s resources.
Author credit: This article is based on research by Niaz Muhammad Shahani, Xigui Zheng, Xin Wei, Jiang Hongwei.
For More Related Articles Click Here