Wheat is a crucial crop that feeds over 30% of the world’s population. Accurately detecting and counting wheat spikes is vital for estimating yield and improving breeding efforts. Researchers have developed a novel deep learning model, RIA-SpikeNet, that can detect and count wheat spikes with unprecedented accuracy, even in challenging field conditions. This breakthrough could revolutionize agricultural practices and help meet the growing global demand for wheat. Wheat is a staple food for billions, and improving its yield is crucial for food security and sustainability.
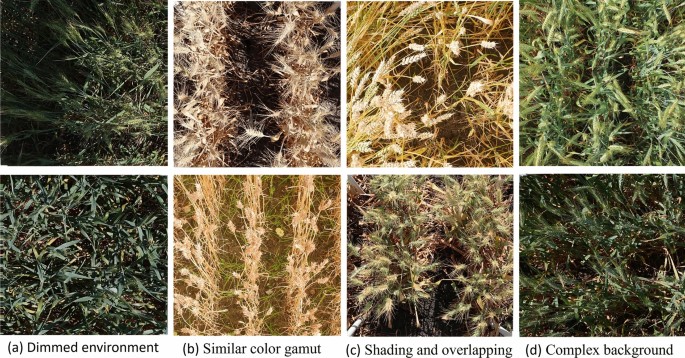
Overcoming Challenges in Wheat Spike Detection
Wheat spike detection and counting has traditionally been a labor-intensive and time-consuming task, relying on manual observation. The rise of image-based detection methods using deep learning has shown promise, but still face significant challenges in complex field environments. These include:
- Lighting variations: Changing weather and shooting angles can lead to unclear and difficult-to-detect wheat spikes, especially under dim conditions.
- Similar colors: The color of wheat spikes can closely resemble the background, making them hard to distinguish.
- Overlapping and shading: Wheat spikes can be obscured by leaves or overlap with each other, hindering accurate detection.
- Complex backgrounds: Cluttered field environments with diverse textures and features can confuse detection algorithms.
RIA-SpikeNet: A Breakthrough in Wheat Spike Detection
To address these challenges, a team of researchers developed RIA-SpikeNet, a deep learning model that can accurately detect and count wheat spikes even in complex field scenarios. The key innovations of RIA-SpikeNet include:
Implicit Decoupling Detection Head: RIA-SpikeNet incorporates an implicit model into the detection head, enabling the algorithm to leverage more contextual information and better distinguish visually similar wheat spikes.
Asymmetric Loss Function: The model uses an asymmetric loss function that assigns different weights to positive and negative samples, helping it focus on correctly identifying difficult or rare wheat spikes.
Optimized Backbone Network: RIA-SpikeNet employs the RepLKNet backbone, which has a larger effective receptive field and enhanced shape information extraction. This allows the model to better capture global features and identify wheat spikes in complex, textured environments.
Impressive Results and Real-World Impact
The researchers tested RIA-SpikeNet on the Global Wheat Head Detection (GWHD) Dataset 2021, a comprehensive dataset covering diverse wheat varieties and field conditions. The model achieved an impressive mean average precision (mAP) of 81.54% and a correlation coefficient (R^2) of 90.29% for wheat spike counting, outperforming state-of-the-art detection models.
These results demonstrate the exceptional performance of RIA-SpikeNet in challenging field scenarios, making it a valuable tool for accurate wheat yield estimation and supporting breeding efforts to enhance wheat production. By providing a reliable, automated solution for wheat spike detection and counting, this research could have a significant impact on global food security and sustainable agriculture.
Author credit: This article is based on research by Changji Wen, Zhenyu Ma, Junfeng Ren, Tian Zhang, Long Zhang, Hongrui Chen, Hengqiang Su, Ce Yang, Hongbing Chen, Wei Guo.
For More Related Articles Click Here