Glioblastomas (GBMs) are the most aggressive type of brain tumors, with a dismal prognosis. While certain genetic alterations have been identified as prognostic biomarkers, the role of the tumor’s cellular heterogeneity in predicting patient outcomes has remained elusive. In a groundbreaking study, researchers have now uncovered a novel approach to leveraging the diverse cell types within GBMs to improve prognosis prediction.
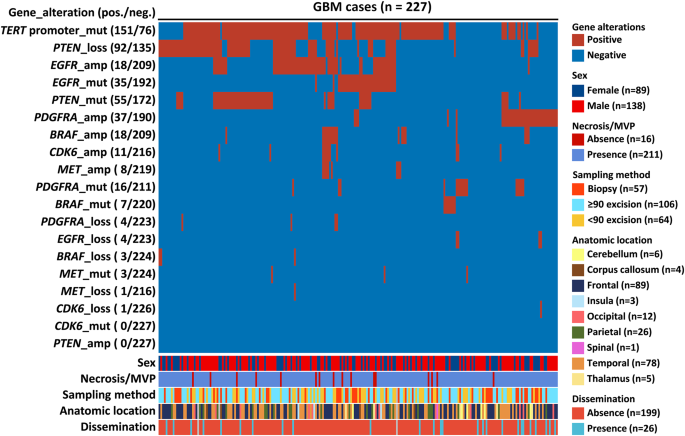
Unraveling the Complexity of Glioblastomas
Glioblastomas are malignant brain tumors that are notoriously difficult to treat, with a median survival of just 1.5 years after diagnosis. Despite intensive therapies, including surgery, radiation, and chemotherapy, the prognosis for patients with GBMs remains dismal. However, recent advances in genomic profiling have shed new light on the underlying drivers of these tumors, opening up new avenues for improving patient outcomes.
Cellular Diversity Holds the Key
One of the hallmarks of GBMs is their remarkable cellular heterogeneity, with a diverse array of cell types present within the same tumor. These include astrocytic, pleomorphic, gemistocytic, and rhabdoid cells, among others. Researchers have long suspected that this cellular diversity might hold the key to better understanding GBM prognosis, but until now, the relationship between cellular composition and patient outcomes has remained unclear.
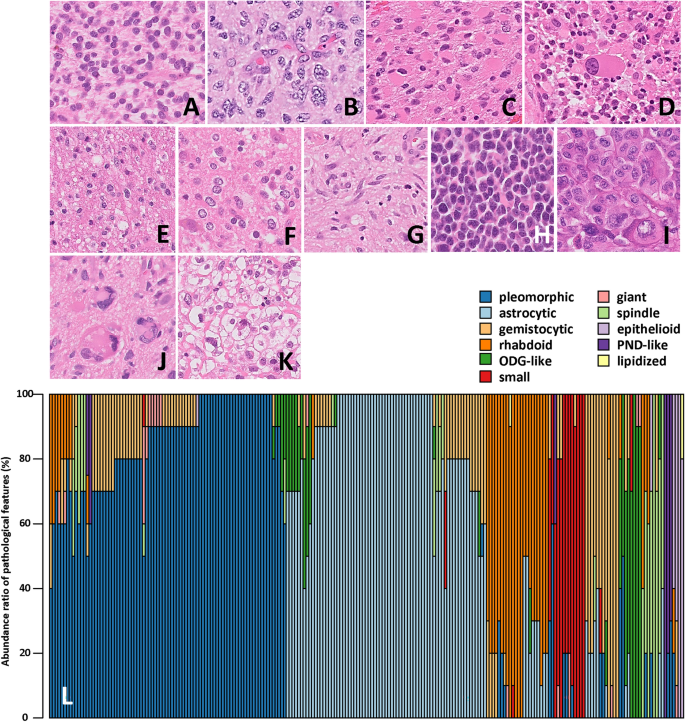
Fig. 2
A Novel Approach to Prognosis Prediction
In this groundbreaking study, a team of researchers analyzed 227 GBM cases, carefully evaluating the abundance of different cellular components within each tumor. Using advanced statistical techniques, such as t-distributed stochastic neighbor embedding (t-SNE), the researchers were able to identify two distinct groups of GBMs with significantly different prognoses.
Interestingly, the researchers found that the presence of a high proportion of gemistocytic cells was associated with a more favorable prognosis, while the abundance of epithelioid cells was linked to a poorer outcome. These findings held true even after accounting for other well-established prognostic factors, such as the methylation status of the MGMT gene promoter.
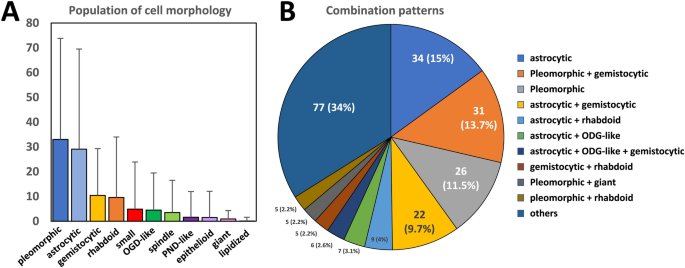
Fig. 3
Unlocking the Prognostic Potential of Cellular Heterogeneity
The researchers developed a simple, linear model that combines the abundance of gemistocytic and epithelioid cells to create a “morphological index” that can effectively predict patient prognosis. Importantly, this index was found to be independent of MGMT promoter methylation status, suggesting that it provides additional, complementary information for assessing a patient’s likely outcome.
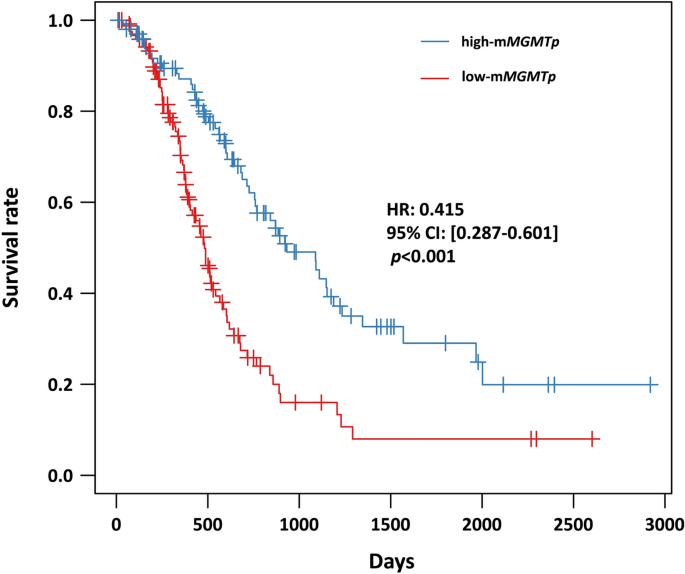
Fig. 4
Implications and Future Directions
This study represents a significant advance in our understanding of GBM biology and highlights the importance of considering the tumor’s cellular heterogeneity when making prognostic assessments. By integrating histological analysis of cellular composition with genomic data, the researchers have demonstrated a practical and effective approach to refining therapeutic strategies and optimizing patient management.
Looking ahead, the researchers suggest that further exploration of the underlying mechanisms driving the differential prognostic impact of specific cell types, such as gemistocytes and epithelioid cells, could lead to the identification of new therapeutic targets and the development of more personalized treatment approaches for patients with GBMs.
Author credit: This article is based on research by Mari Kirishima, Seiya Yokoyama, Toshiaki Akahane, Nayuta Higa, Hiroyuki Uchida, Hajime Yonezawa, Kei Matsuo, Junkoh Yamamoto, Koji Yoshimoto, Ryosuke Hanaya, Akihide Tanimoto.
For More Related Articles Click Here