Researchers at Caltech have developed a groundbreaking algorithm inspired by the human brain’s remarkable ability to learn and adapt. This new approach, called the Functionally Invariant Path (FIP) algorithm, enables neural networks to continuously update with new data without losing previously encoded information. This breakthrough has wide-ranging applications, from improving online recommendations to fine-tuning self-driving cars. The algorithm’s development was influenced by neuroscience research on how animals and humans can rewire their brains to relearn skills after injury. This discovery could pave the way for more flexible and resilient artificial intelligence systems. Artificial neural networks, machine learning
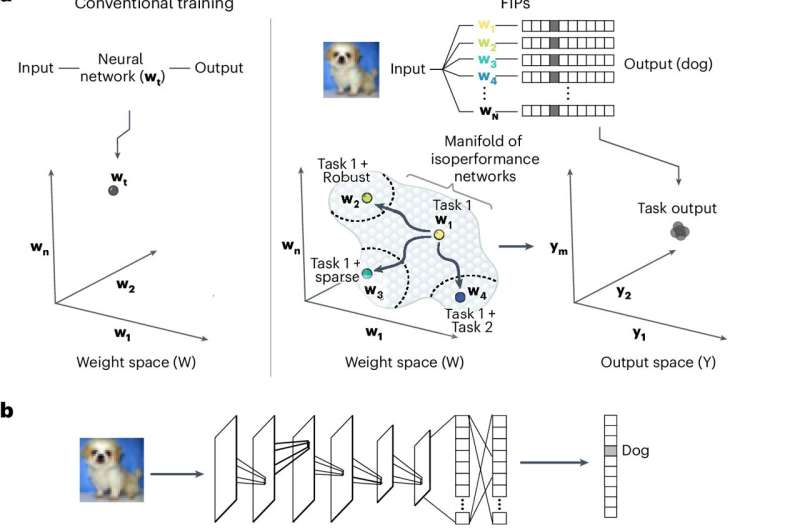
Crossing the Frontier in Neural Networks
Neural networks have proven very good at learning tasks like how to identify handwritten digits. Yet, these models have long struggled with an issue called catastrophic forgetting. Even if trained on other tasks, they can successfully learn the new assignments but will continue to “forget” how to perform the originals. For example, intelligent systems inspired by human brain (called artificial neural network) such as the one a self-driving car uses has a serious limitation: once it is programmed to do something, e.g. recognize an apple on the road and take specific action to avoid it, in order to teach their AI new tricks tens of thousands of times more effort than training casual human responds would require complete reprogramming.
But living brains — like the ones that human and animals have — are so much more flexible No one is born walking, talking or playing games, all these are acquired skills and an individual can soon acquire how to play a new game without having relearn how to step again. Taking a cue from this innate plasticity, researchers at Caltech have developed an algorithm that can help neural networks continue to learn by updating with new datasets, eliminating the need for training them from scratch.
The FIP Algorithm: The Brain’s Hidden Power
The researchers continued to design a new algorithm for this purpose, called Functionally Invariant Path (FIP) algorithm, in the lab of Matt Thomson, assistant professor of computational biology and Heritage Medical Research Institute (HMRI) Investigator. Inspired by neuroscience work being done at Caltech, and in particular research on birds that can rewire their brains to learn how to sing again after suffering a brain injury (like the work of Carlos Lois, a Research Professor of Biology at Caltech)
The 6-compartment FIP model was derived according to the principles of differential geometry. This framework rewrites the weights of a neural network without forgetting their previous encoded information, which in turn, solves catastrophic forgetting. All of this, says Thomson, was a years long project that began with an inquiry into the basic science behind how brains learn flexibly. I need to give that to an artificial neural network.
The Real World — The Visions for Flexible AI
The brains behind the FIP algorithm, Guru Raghavan and Matt Thomson, are working with Julie Schoenfeld at Caltech to develop a business venture around it; through Julie’s prodding, Yurts has officially launched. FIP algorithm can be used across an array of different use cases, from making better recommendations on online stores all the way to tuning self-driving cars.
This breakthrough has consequences beyond the applications. The FIP algorithm presents an important advancement towards the creation of more flexible, adaptable artificial intelligence designs, based on the unusual flexibility in the way the human brain functions. The emergence of the FIP algorithm is a wonderful example of how, as AI advances, groundwork is laid for evolutionary machine learning; a future in which artificial intelligence learns and adapts throughout its lifetime – as did the spectacular biological brains that gave it rise.