Researchers have developed a groundbreaking approach using a deep learning model called GSCANet to diagnose Alzheimer’s disease (AD) early on. By combining magnetic resonance imaging (MRI) data, functional connectivity features, and neuropsychological assessments, this innovative method can accurately identify different stages of AD, including early mild cognitive impairment (EMCI) and late mild cognitive impairment (LMCI). This breakthrough could pave the way for earlier intervention and improved patient outcomes. The study highlights the power of artificial intelligence in revolutionizing the field of neurodegenerative disease detection.
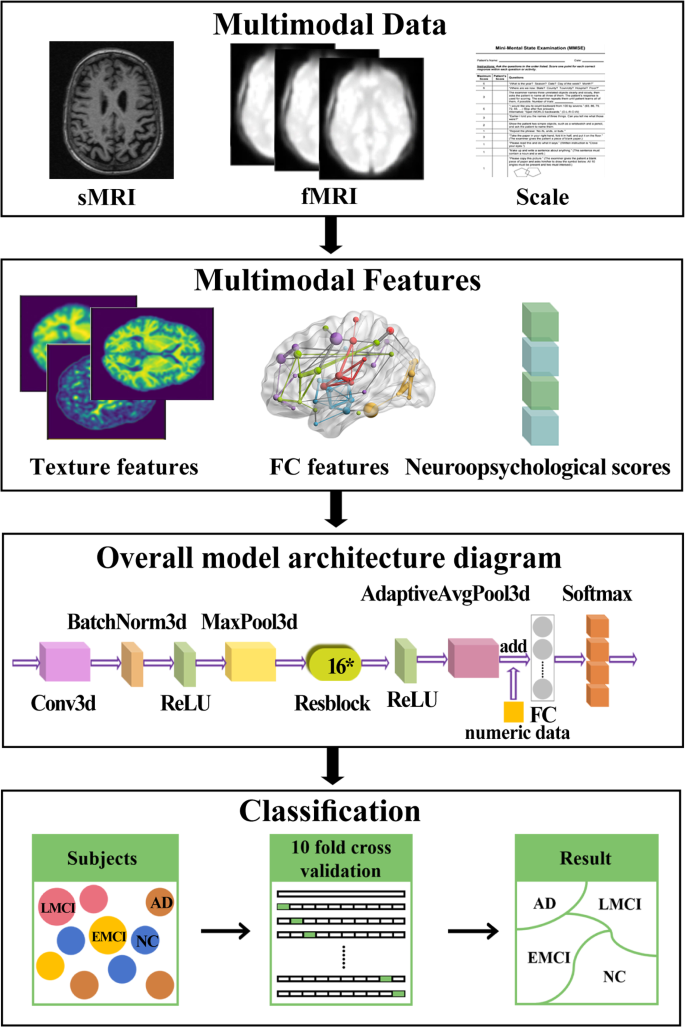
Unraveling the Complexity of Alzheimer’s Disease
Alzheimer’s disease (AD) is a devastating neurodegenerative condition that affects millions of people worldwide. As the global population ages, the burden of AD is only expected to grow, making early diagnosis and intervention crucial. Unfortunately, current diagnostic approaches often fall short, as they typically focus on a single aspect of the disease, such as brain structure or function, and fail to capture the full complexity of AD.
Harnessing the Power of Multimodal Data
To address this challenge, a team of researchers has developed an innovative deep learning model called the Group Self-Calibrated Coordinate Attention Network (GSCANet). This model integrates multiple sources of data, including magnetic resonance imaging (MRI), functional connectivity (FC) features, and neuropsychological assessments, to provide a comprehensive view of the brain’s structure, function, and cognitive changes associated with AD.
Expanding the Receptive Field for Improved Feature Extraction
One of the key innovations of the GSCANet model is its ability to expand the receptive field, which refers to the region of the input image that a neuron in a deep learning network can “see.” By incorporating group self-calibrated convolution and coordinated attention modules, the model can capture a broader and more detailed representation of AD-related brain changes, leading to enhanced feature extraction and improved classification performance.
Unlocking the Secrets of Early-Stage AD
The researchers tested the GSCANet model on data from the Alzheimer’s Disease Neuroimaging Initiative (ADNI) and a hospital-based dataset, and the results were impressive. The model was able to accurately differentiate between AD, early mild cognitive impairment (EMCI), late mild cognitive impairment (LMCI), and healthy controls, with an accuracy of up to 84.67% in the EMCI vs. LMCI classification.
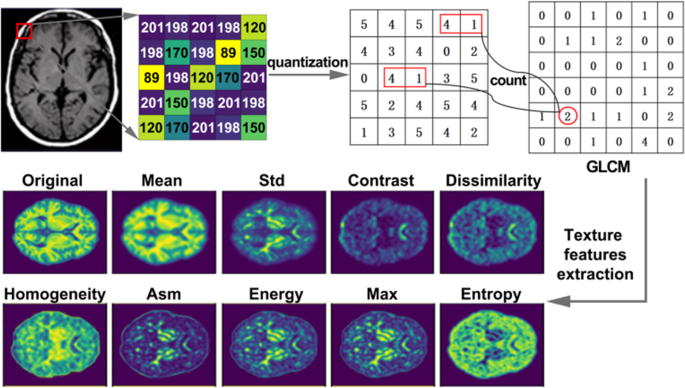
Paving the Way for Early Intervention
This breakthrough is particularly significant, as the ability to detect AD in its early stages is crucial for providing timely intervention and potentially slowing the disease’s progression. By accurately identifying individuals with EMCI, the GSCANet model could enable healthcare providers to implement targeted therapies and lifestyle modifications that could make a meaningful difference in the lives of those affected by this devastating disease.
Advancing the Field of Neurodegenerative Disease Detection
The success of the GSCANet model highlights the immense potential of deep learning in revolutionizing the field of neurodegenerative disease detection. By leveraging the power of multimodal data and innovative feature extraction techniques, this research paves the way for more accurate and comprehensive diagnoses, ultimately leading to better patient outcomes and a deeper understanding of the complex mechanisms underlying Alzheimer’s disease.
Author credit: This article is based on research by Xiaojie Yu, Jingyuan Liu, Yinping Lu, Shintaro Funahashi, Toshiya Murai, Jinglong Wu, Qi Li, Zhilin Zhang.
For More Related Articles Click Here