Cardiac arrhythmias are a leading cause of death globally, and early detection is crucial for effective treatment. Researchers have developed a cutting-edge deep learning model that can automatically diagnose cardiac arrhythmia from 12-lead electrocardiogram (ECG) data with unprecedented accuracy. This breakthrough technology combines the power of convolutional neural networks and recurrent neural networks with a multi-head attention mechanism to extract both spatial and temporal features from ECG signals. By accurately classifying ECG data into five different types of cardiac arrhythmia, this model has the potential to revolutionize how healthcare professionals diagnose and manage cardiovascular diseases.
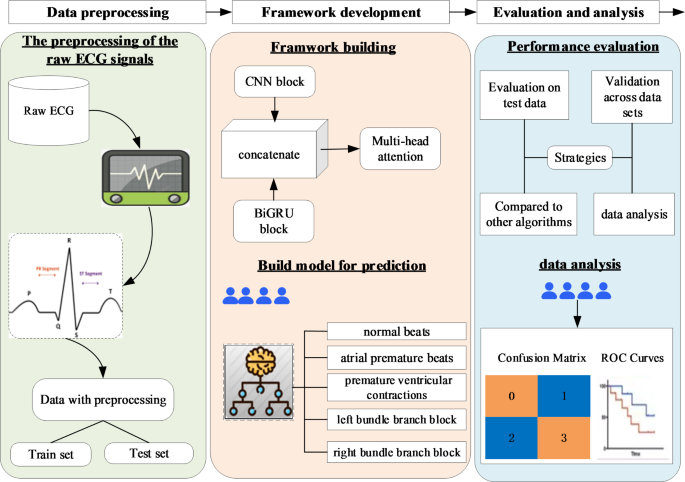
Unlocking the Secrets of Cardiac Arrhythmia
Cardiovascular diseases are a global health crisis, with cardiac arrhythmias being one of the leading causes of death worldwide. Early and accurate diagnosis of these abnormal heart rhythms is crucial for effective treatment and preventing adverse outcomes. Traditionally, healthcare professionals have relied on the electrocardiogram (ECG) to detect and classify cardiac arrhythmias, but this process can be time-consuming and requires extensive clinical expertise.
The Power of Deep Learning in ECG Analysis
To address these challenges, researchers have turned to advanced artificial intelligence (AI) techniques, such as deep learning, to automate the analysis of ECG data. Deep learning models, like convolutional neural networks (CNNs) and recurrent neural networks (RNNs), have demonstrated exceptional performance in extracting meaningful features from complex, time-series data like ECG signals. By combining these powerful techniques with a multi-head attention mechanism, the researchers have developed a highly accurate and robust model for diagnosing cardiac arrhythmias.
A Hybrid Deep Learning Approach
The key innovation of this research is the development of a hybrid deep learning model that integrates the strengths of CNNs and RNNs to comprehensively capture both the spatial and temporal features of ECG signals. The CNN component is responsible for extracting the spatial patterns, while the RNN (specifically, intelligenceinhealthcare’>AI in healthcare continues to advance, we can expect to see more transformative technologies like this deep learning model for ECG analysis. By leveraging the power of AI, researchers and clinicians can work together to unlock new insights, improve patient care, and ultimately save more lives.
Author credit: This article is based on research by Xiangyun Bai, Xinglong Dong, Yabing Li, Ruixia Liu, Henggui Zhang.
For More Related Articles Click Here