Researchers have developed a powerful new deep learning model, called SFSCF-Net, that can accurately distinguish between closely related subcategories of objects, like different species of birds or models of cars. By using innovative techniques like “salient feature suppression” and “cross-feature fusion,” SFSCF-Net outperforms existing methods on several challenging fine-grained visual classification tasks. This breakthrough could lead to exciting applications in areas like smart retail, security, and wildlife conservation. Machine learning and computer vision continue to push the boundaries of what’s possible in computer-based recognition and classification.
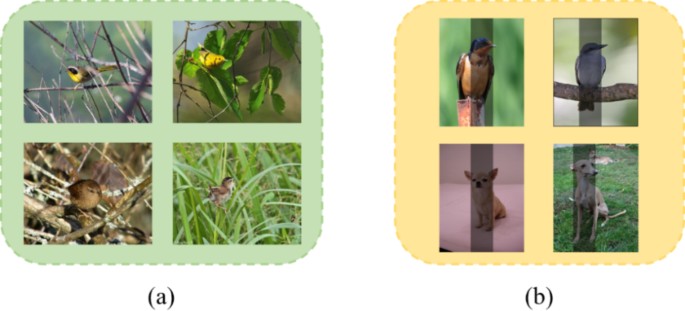
Tackling the Challenges of Fine-Grained Visual Classification
Accurately distinguishing between closely related subcategories of objects, like different bird species or car models, is a challenging task for computer vision systems. This “fine-grained visual classification” (FGVC) problem is important for a wide range of applications, from smart retail to security and wildlife conservation. However, the small differences between subcategories, combined with complex backgrounds and varying object poses, make FGVC a tough nut to crack.
A Novel Approach: SFSCF-Net
To address these challenges, a team of researchers developed a new deep learning model called SFSCF-Net (Significant Feature Suppression and Cross-Feature Fusion Network). SFSCF-Net uses three key innovations to improve fine-grained classification:
1. Object-Level Image Generation (OIG): This step isolates the target object from the background, helping the network focus on the most relevant features.
2. Salient Feature Suppression Module (SFSM): SFSM accurately identifies and suppresses the most distinctive, “salient” features, forcing the network to also consider less prominent but still important features.
3. Cross-Feature Fusion Method (CFM): CFM integrates features from different layers of the network, allowing the model to capture a richer, more diverse set of visual cues.
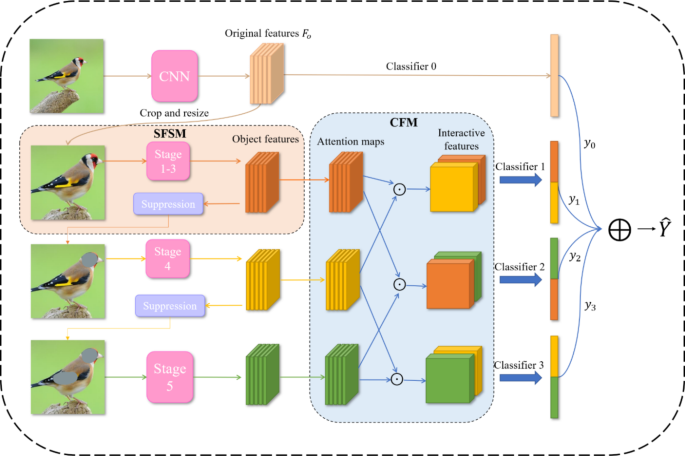
Fig. 2
Impressive Results Across Challenging Datasets
The researchers tested SFSCF-Net on four well-known FGVC benchmark datasets, covering birds, aircraft, cars, and dogs. Remarkably, the model achieved state-of-the-art or highly competitive results across all four datasets, outperforming a wide range of existing methods.
For example, on the challenging CUB-200-2011 bird dataset, SFSCF-Net improved classification accuracy by up to 7.7% compared to previous approaches. And on the FGVC-Aircraft dataset, it beat out other top-performing models by 0.7-1.2%.
Unlocking New Possibilities
The success of SFSCF-Net demonstrates the power of innovative deep learning techniques for tackling complex visual classification problems. By intelligently suppressing and fusing visual features, the model is able to extract the most relevant and discriminative information, even in the face of subtle subcategory differences and challenging backgrounds.
This breakthrough could pave the way for exciting new applications in areas like smart retail (e.g., automatic inventory tracking), security (e.g., fine-grained object recognition), and wildlife conservation (e.g., automated species identification). As computer vision and deep learning technologies continue to advance, the possibilities for fine-grained visual classification are truly endless.
Author credit: This article is based on research by Shengying Yang, Xinqi Yang, Jianfeng Wu, Boyang Feng.
For More Related Articles Click Here