Malaria, a deadly disease caused by Plasmodium parasites, has plagued humanity for centuries. Despite advancements in medicine, the emergence of drug-resistant strains has made it increasingly challenging to combat this global health threat. However, a new study by researchers Soham Choudhuri and Bhaswar Ghosh offers a promising solution – leveraging the power of single-cell transcriptomics and machine learning to identify crucial proteins as drug targets and generate potential new drug molecules. This innovative approach holds the key to tackling the ever-evolving malaria parasite and paving the way for more effective and long-lasting treatment options.
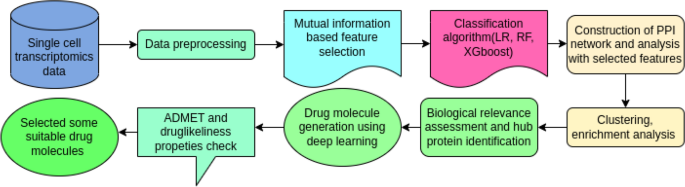
Unraveling the Complexity of Malaria
Malaria, a deadly disease caused by Plasmodium parasites, remains a formidable global health challenge. Despite significant progress in treatment methods, the emergence of drug-resistant strains of Plasmodium falciparum, the most deadly malaria parasite, has posed serious challenges to effective treatment. Understanding the complex life cycle of the Plasmodium parasite, which involves both human and mosquito hosts, is crucial for developing efficient drugs.
Harnessing the Power of Single-Cell Transcriptomics
The study by Choudhuri and Ghosh leverages the power of single-cell transcriptomics, a cutting-edge technology that allows researchers to analyze the gene expression patterns of individual cells. By studying the transcriptomes of Plasmodium falciparum cells across different life cycle stages, the researchers were able to identify crucial proteins that are essential for the parasite’s survival.
Computational Approach to Drug Target Identification
The researchers implemented a mutual information-based feature reduction algorithm and classification models to select the most important proteins from the single-cell transcriptomic datasets. These key proteins were then used to construct protein-protein interaction (PPI) networks, revealing the critical hubs and bottlenecks that are essential for the parasite’s survival.
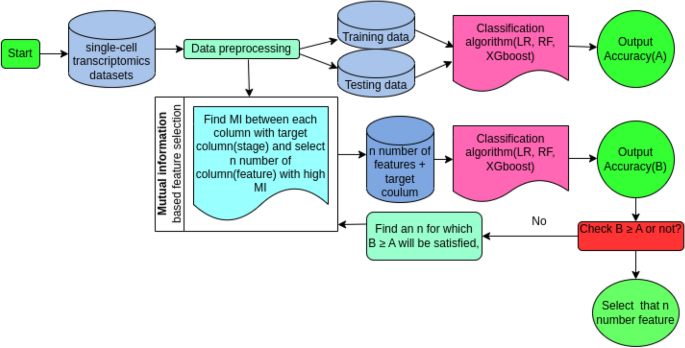
Generating Potential Drug Molecules
With the identification of crucial protein targets, the researchers turned their attention to drug discovery. They used a deep learning-based generative model called TargetDiff to computationally predict potential drug molecules that could bind to the identified protein targets. These drug candidates were then analyzed for their physicochemical, medicinal, and ADMET (absorption, distribution, metabolism, excretion, and toxicity) properties to identify the most promising lead compounds.
Paving the Way for Improved Malaria Treatment
The study by Choudhuri and Ghosh demonstrates the power of integrating single-cell transcriptomics, computational analysis, and machine learning techniques to accelerate the discovery of new antimalarial drugs. By targeting crucial proteins in both the asexual and sexual stages of the Plasmodium falciparum life cycle, this approach holds the potential to develop more effective and long-lasting treatment options, ultimately reducing the global burden of this devastating disease.
The researchers’ findings highlight the importance of interdisciplinary collaboration in the fight against malaria. By combining expertise in biology, bioinformatics, and machine learning, this study represents a significant step forward in the quest to conquer one of the world’s deadliest infectious diseases.
Author credit: This article is based on research by Soham Choudhuri, Bhaswar Ghosh.
For More Related Articles Click Here