Researchers have developed an innovative approach that combines computational fluid dynamics (CFD) and machine learning techniques to accurately predict the concentration of solutes in a membrane separation process. This breakthrough could significantly improve water treatment methods, making them more efficient and cost-effective. The study evaluated various regression models, including Multi-Layer Perceptron (MLP), Kernel Ridge Regression (KRR), and K-Nearest Neighbors (KNN), all optimized using the Harris Hawks Optimization (HHO) algorithm. The results show that the MLP model outperformed the others, achieving an impressive R2 score of 0.98637 on the test data, with low root-mean-square error (RMSE) and mean absolute error (MAE) values. This research paves the way for more accurate predictive modeling in membrane science, which is crucial for fields like environmental science, chemistry, and engineering.
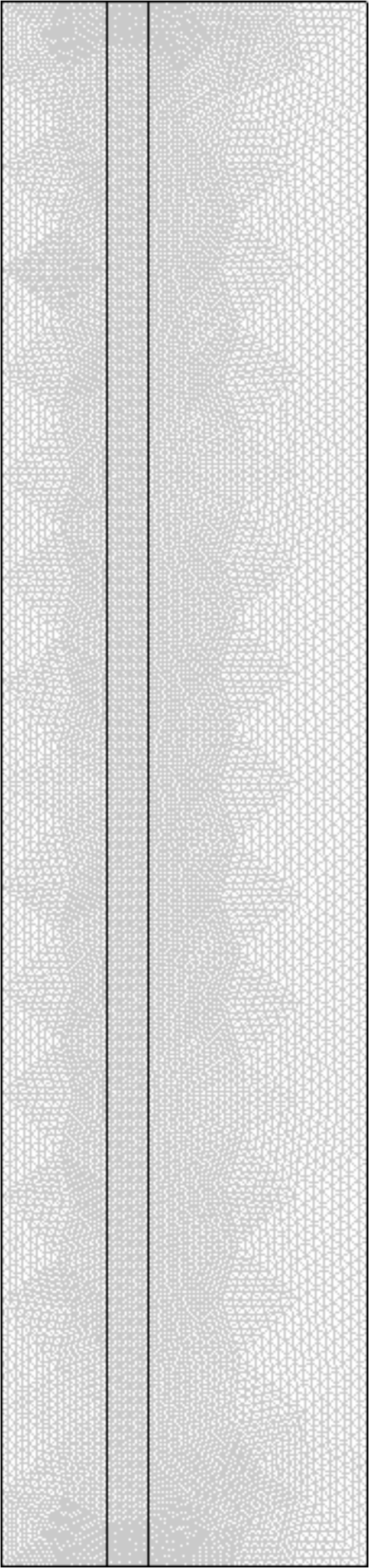
Unlocking the Power of Hybrid Modeling
Membrane separation processes are widely used in water treatment, but accurately predicting the concentration of solutes has been a challenging task. Traditionally, computational fluid dynamics (CFD) has been the go-to method, but it can be computationally expensive and complex. To overcome these limitations, the researchers in this study integrated CFD with machine learning (ML) techniques, leveraging the strengths of both approaches.
The team evaluated five regression models – MLP, KRR, KNN, Radius Nearest Neighbor (RNN), and LASSO – all optimized using the Harris Hawks Optimization (HHO) algorithm. HHO is a powerful optimization method inspired by the hunting behavior of Harris hawks, known for its superior exploration and exploitation capabilities.
MLP Emerges as the Standout Performer
Among the models tested, the MLP stood out as the clear winner, demonstrating exceptional predictive performance. It achieved an R2 score of 0.98637 on the test data, indicating that the model was able to capture a significant portion of the variance in the dataset. Additionally, the MLP model had the lowest RMSE and MAE values, showcasing its precision and generalization ability.
The researchers attribute the MLP’s success to its deep learning architecture, which allows it to capture complex nonlinear relationships in the data. The KRR model also performed well, with an R2 score of 0.95795 on the test data, closely following the MLP. The KNN and RNN models showed reasonable performance, while the LASSO model had the lowest scores among the evaluated models.
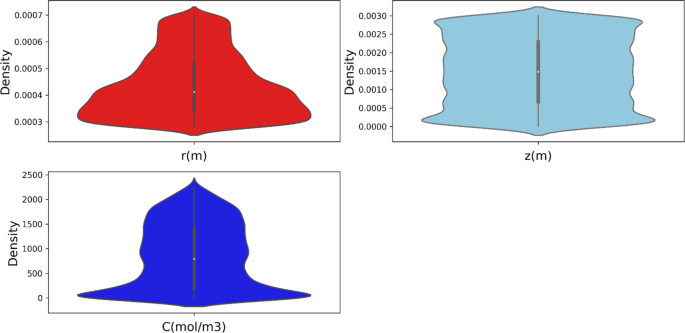
Fig. 2
Unlocking the Potential of Hybrid Modeling
The findings of this study highlight the power of integrating CFD and machine learning techniques for accurate predictive modeling in membrane separation processes. By leveraging the strengths of both approaches, the researchers were able to develop hybrid models that outperform traditional methods, paving the way for more efficient and cost-effective water treatment solutions.
This research has far-reaching implications for professionals and researchers in fields such as environmental engineering, chemical engineering, and water treatment. The ability to accurately predict solute concentrations can lead to significant improvements in membrane separation processes, ultimately benefiting both the environment and human health.
As the world faces growing challenges in water scarcity and water quality, innovative solutions like the one presented in this study become increasingly crucial. By harnessing the power of machine learning and CFD, researchers are unlocking new possibilities for transforming the way we approach water treatment and separation science.
Author credit: This article is based on research by Kamal Y. Thajudeen, Mohammed Muqtader Ahmed, Saad Ali Alshehri.
For More Related Articles Click Here