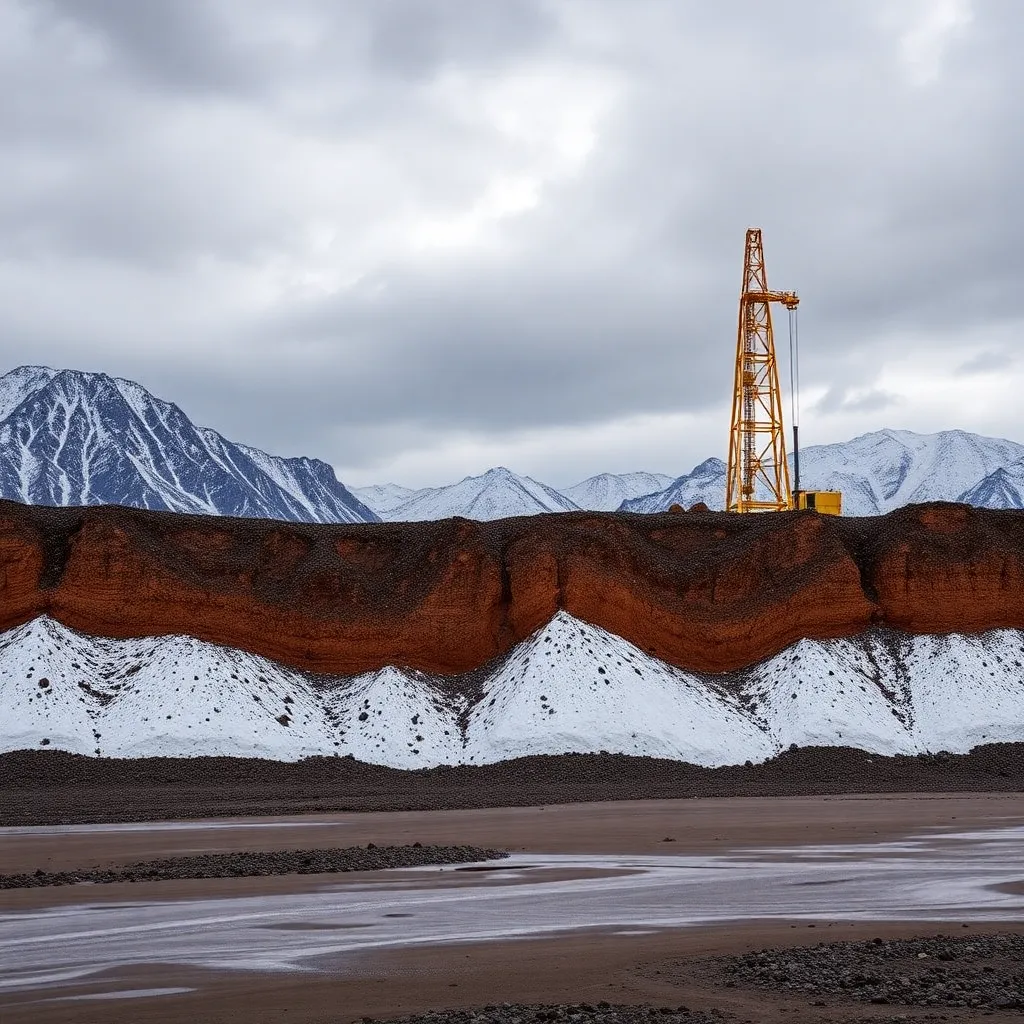
Researchers have developed a cutting-edge method to accurately predict surface subsidence in mining operations, leveraging the power of Unmanned Aerial Vehicle (UAV) oblique photogrammetry. This innovative approach combines the Probabilistic Integral Method (PIM) with a multi-layer recurrent neural network (RNN), effectively mitigating the impact of internal and external factors on surface subsidence. By analyzing data from the Pulang copper mine in Yunnan, China, the researchers achieved a remarkable prediction accuracy of 91.47% for maximum surface subsidence height and 87.52% for subsidence area. This research expands the applications of UAV photogrammetry in mining and establishes a cost-effective and efficient process for predicting mine surface subsidence, paving the way for safer and more sustainable mining practices. Unmanned aerial vehicle, Photogrammetry, Block caving, Surface subsidence, Recurrent neural network
Unraveling the Complexities of Mining-Induced Surface Subsidence
Mining operations, particularly the block caving method, can have a significant impact on the surrounding environment, often resulting in surface subsidence. This phenomenon occurs as the ore body collapses and the overlying rock formations gradually sink, leading to the formation of large-scale collapse areas resembling open-pit mines. The Pulang copper mine in Yunnan, China, the largest mine utilizing the block caving method in the country, has experienced severe surface subsidence issues, with the maximum block caving height reaching an impressive 370 meters.
Predicting and mitigating the impact of surface subsidence is crucial for safeguarding the environment, infrastructure, and the well-being of nearby communities. Conventional methods for predicting surface subsidence, such as empirical, experimental, and computational approaches, often fall short in accurately capturing the complex interplay between internal and external factors that influence this process.
Harnessing the Power of UAV Oblique Photogrammetry
To address this challenge, researchers have developed a novel prediction method that combines the strengths of UAV oblique photogrammetry and advanced data analysis techniques. By leveraging the high-resolution imaging capabilities of UAVs, the researchers were able to construct detailed 3D models of the mining site, even in areas that were previously inaccessible due to the risks posed by the collapsing surface.
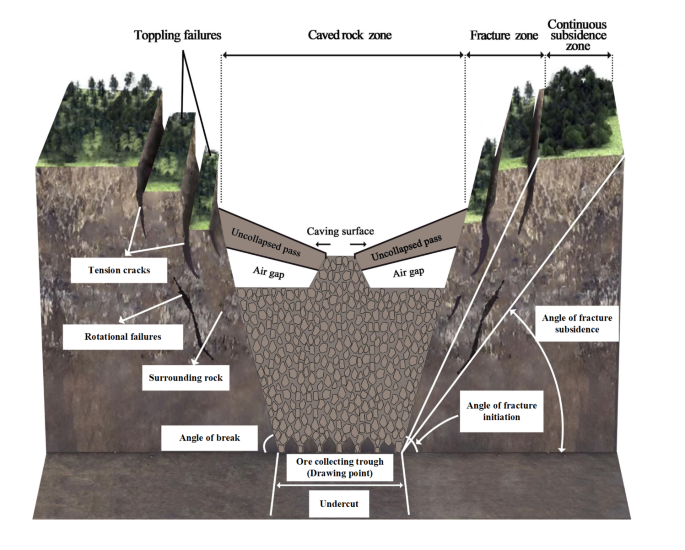
The researchers employed a sophisticated positioning method, integrating Network Real-Time Kinematic (NRTK) and Post-Processed Kinematic (PPK) techniques, to ensure the accuracy of the aerial surveys. This approach allowed them to overcome the challenges of traditional UAV aerial surveying, such as the need for ground control points, which can be difficult to establish in complex mining environments.
Integrating PIM and RNN for Accurate Subsidence Prediction
The researchers developed a two-stage prediction model that combines the Probabilistic Integral Method (PIM) with a recurrent neural network (RNN). The PIM is a numerical model based on stochastic medium theory, which simulates the distribution pattern of rock and surface movement influenced by mining activities over time and space.
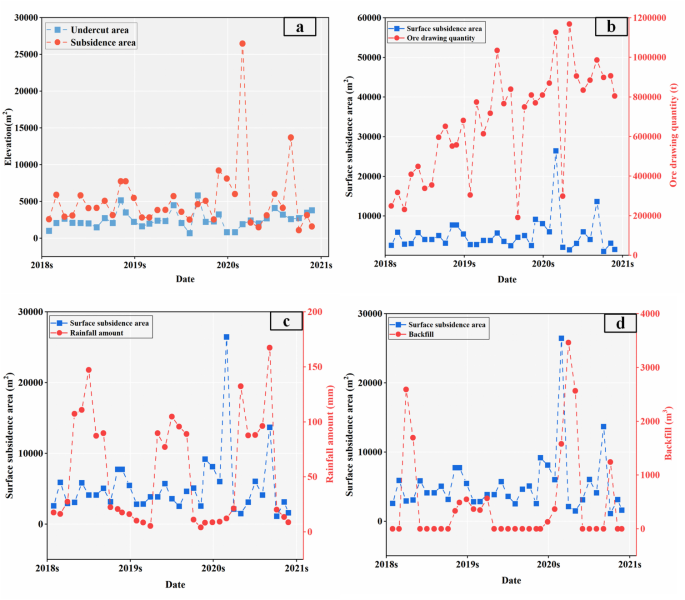
Fig. 2
The RNN component of the model, known as the FIMF-RNN, effectively addresses the limitations of traditional time-series prediction methods. By integrating the PIM’s physical understanding of the subsidence process with the RNN’s ability to learn complex patterns, the researchers were able to accurately predict both the maximum subsidence height and the affected subsidence area.
Validating the Approach with Pulang Copper Mine Data
To evaluate the accuracy of their prediction model, the researchers analyzed data from the Pulang copper mine spanning 2018 to 2020. The results were impressive, with the FIMF-RNN model achieving a prediction accuracy of 91.47% for maximum surface subsidence height and 87.52% for subsidence area.
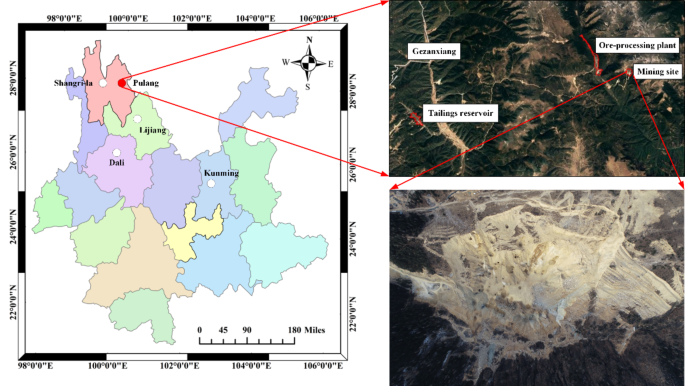
Fig. 3
The researchers also explored the influence of various external factors, such as undercut area, ore drawing quantity, rainfall, and surface backfilling, on the surface subsidence process. By incorporating these factors into their model, they were able to better capture the complex dynamics of the mining environment and improve the overall predictive accuracy.
Transforming Mining Operations with Innovative Subsidence Prediction
This groundbreaking research expands the potential applications of UAV oblique photogrammetry within the mining industry. By establishing a cost-effective and efficient operational procedure for predicting mine surface subsidence, the researchers have provided crucial data support for mitigating the hazards associated with block caving mining techniques.
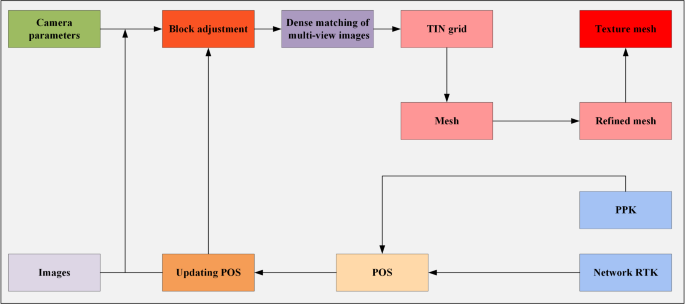
Fig. 4
The ability to accurately forecast surface subsidence can help mining companies plan their operations more effectively, minimize environmental impacts, and ensure the safety of nearby infrastructure and communities. Moreover, this research lays the foundation for further advancements in the field, potentially leading to the development of more sophisticated prediction models and the integration of additional data sources, such as satellite imagery and sensor networks.
As the global demand for minerals and resources continues to grow, the need for innovative and sustainable mining practices becomes increasingly crucial. The research presented in this study represents a significant step forward in addressing the challenges of mining-induced surface subsidence, paving the way for a more responsible and environmentally-conscious extraction of valuable resources.
Author Credit: This article is based on research by Weijia Ling, Xinglong Feng, Liguan Wang, Zhonghua Zhu, Shiwen Wang, Haiying Fu, Shuwen Zhang, Ying Zhao.
For More Related Articles Click Here