Researchers have developed a powerful new technique that combines deep learning and traditional image processing methods to accurately recognize scenes in open-pit coal mining areas using high-resolution satellite imagery. This breakthrough could lead to better monitoring and management of these critical mining operations, which can have significant impacts on the surrounding environment. The study combines features extracted using Gray-Level Co-occurrence Matrix (GLCM) and Gabor filters with deep features from Convolutional Neural Networks (CNNs) and Graph Convolutional Networks (GCNs) to create a comprehensive understanding of mining scenes. This fusion of shallow and deep features achieves over 92% accuracy in recognizing different mining features, outperforming other state-of-the-art methods.
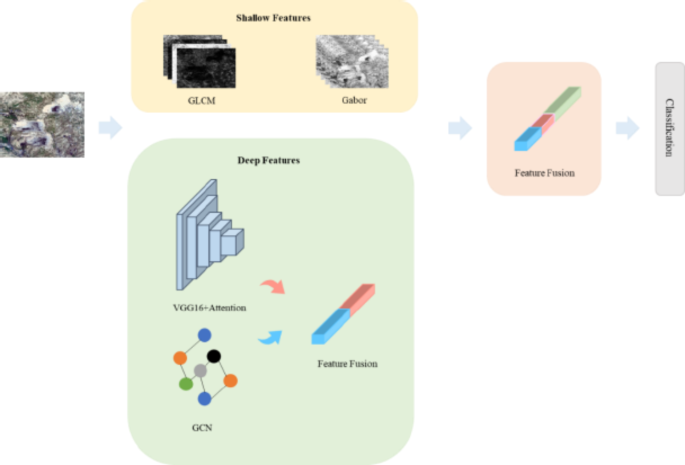
Mapping the Footprint of Open-Pit Mining
Open-pit coal mining is a major industrial activity that can have significant impacts on the surrounding landscape and environment. These large-scale, long-term mining operations can drastically alter the natural terrain, leading to substantial damage and disruption. Accurately assessing and monitoring these changes is crucial for effective management and reclamation efforts.
High-resolution satellite imagery provides a wealth of detailed information about the surface features in mining areas. However, extracting and interpreting this data can be challenging. Traditional image processing techniques rely on manually designed features, which have limited capabilities in capturing the complex, high-level information present in these images. On the other hand, deep learning models, while powerful, often require large amounts of training data to achieve good performance.
Fusing Shallow and Deep Features for Robust Scene Recognition
To address these limitations, the researchers developed a novel framework that combines the strengths of traditional and deep learning approaches. The key features of this framework include:
1. Shallow Feature Extraction: The team used GLCM and Gabor filters to extract local and global texture features, respectively, providing a comprehensive understanding of the scene’s visual characteristics.
2. Deep Feature Extraction: The deep learning component of the framework consists of two branches: a key feature extraction module that uses attention mechanisms to highlight important local information, and a contextual feature extraction module that employs Graph Convolutional Networks (GCNs) to capture the relationships between different scene elements.
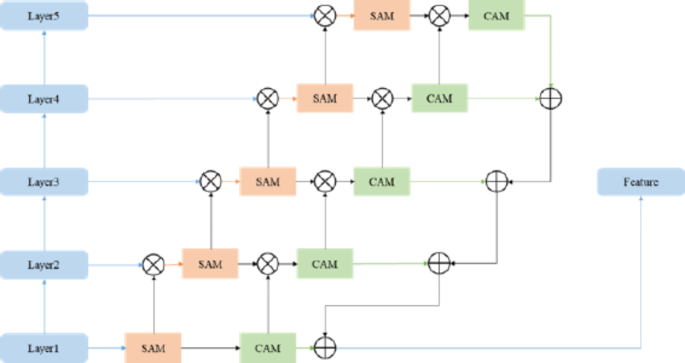
3. Feature Fusion and Classification: The shallow and deep features are then combined and fed into a Particle Swarm Optimization-based Support Vector Machine (PSO-SVM) classifier, which optimizes the parameters to achieve high classification accuracy.
Unlocking the Potential of Open-Pit Mining Monitoring
The researchers tested their framework on two publicly available remote sensing scene datasets, as well as a dataset of open-pit coal mining areas in China. The results were impressive, with the proposed method achieving classification accuracies of over 92% – a significant improvement over existing state-of-the-art techniques.
This breakthrough has important implications for the effective management and monitoring of open-pit coal mining operations. By accurately recognizing and mapping the various features of these complex mining landscapes, authorities and researchers can better understand the environmental impacts and plan for appropriate mitigation and reclamation strategies.
Moving forward, the researchers plan to further optimize their framework to enable end-to-end processing and to improve the model’s ability to distinguish between similar mining features, such as stripping areas and discharge sites. As the world continues to grapple with the challenges of sustainable resource extraction, innovations like this deep learning-based approach to remote sensing analysis will be crucial for driving informed decision-making and environmental stewardship.
Author credit: This article is based on research by Yang Liu, Jin Zhang.
For More Related Articles Click Here