Researchers have developed a groundbreaking deep learning model that can accurately analyze the fluid spaces within the inner ear, providing a powerful new tool for diagnosing conditions like Meniere’s disease and fluctuating sensorineural hearing loss. By leveraging 3D magnetic resonance imaging (MRI) data and advanced neural networks, this automated system can segment the delicate endolymph and perilymph fluid spaces with unprecedented precision, paving the way for more reliable and efficient clinical assessments. This research represents a significant advancement in the field of inner ear imaging and holds the potential to transform the way healthcare providers approach the diagnosis and management of complex inner ear disorders.
Unraveling the Mysteries of the Inner Ear
The human inner ear is a remarkable and intricate structure, home to the essential components responsible for our sense of hearing and balance. At the core of this delicate system lies a critical pathological condition known as endolymphatic hydrops (EH), a hallmark of various inner ear disorders. EH is characterized by an imbalance in the fluid volumes within the inner ear, leading to the distension of the structures that enclose the endolymphatic space.
Historically, the only way to definitively confirm the presence of EH was through invasive post-mortem examination of the temporal bone. However, the development of advanced imaging technologies, particularly magnetic resonance imaging (MRI), has opened up new avenues for non-invasive detection and assessment of this condition.
A Breakthrough in Automated Fluid Analysis
In a groundbreaking study, a team of researchers from Jeonbuk National University in South Korea have developed a novel deep learning-based approach to analyze the fluid spaces within the inner ear using 3D MRI data. Their method, which combines state-of-the-art neural network architectures with a unique “data pair” strategy, has demonstrated remarkable accuracy in segmenting the endolymph and perilymph fluid spaces.
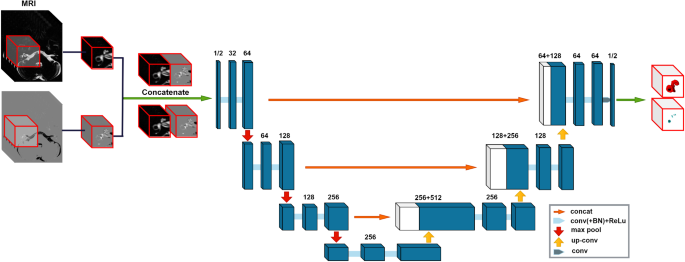
The researchers built a comprehensive dataset of MRI scans, including MR cisternography (MRC) and HYDROPS-Mi2 images, with detailed annotations of the fluid spaces. This allowed them to train a 3D U-Net, a powerful deep learning model, to simultaneously segment the perilymph and endolymph fluid spaces in a single, end-to-end process.
Unparalleled Accuracy and Efficiency
The results of the study are truly impressive. The researchers’ 3D segmentation approach achieved a Dice similarity coefficient (DSC) of 0.9574 for the total fluid space and 0.8400 for the endolymph fluid space, indicating an exceptionally high level of accuracy. Furthermore, the endolymphatic hydrops (EH) volume ratio calculated by the deep learning model showed strong agreement with the ground truth values, as demonstrated by the high intraclass correlation coefficient (ICC) of 0.951.
This automated system offers several key advantages over traditional manual segmentation methods. First, it eliminates the time-consuming and labor-intensive process of manual delineation, which can be particularly challenging for the small and irregularly shaped endolymphatic space. Second, the model’s ability to simultaneously segment both the perilymph and endolymph fluid spaces in a single pass ensures consistent and reliable EH volume ratio calculations, reducing the risk of inter-specialist variability.
Transforming Clinical Practice
The implications of this research extend far beyond the technical achievements. By automating the analysis of inner ear fluid spaces, the researchers have paved the way for more efficient and accurate diagnosis of conditions like Meniere’s disease and fluctuating sensorineural hearing loss. These disorders are often characterized by EH, and the ability to quantify the EH volume ratio through non-invasive MRI can significantly improve clinical decision-making and patient care.
Moreover, the researchers have made their work openly available, sharing the code and models on GitHub. This gesture of scientific collaboration and transparency will undoubtedly accelerate the adoption and further development of this technology, ultimately benefiting the broader medical community and the patients they serve.
Future Directions and Broader Implications
While the current study represents a remarkable achievement, the researchers acknowledge the need for further refinement and validation. Expanding the training dataset, exploring alternative deep learning architectures, and assessing the model’s generalizability across different imaging modalities and patient populations are all areas for future investigation.
Beyond the immediate clinical applications, this research also holds broader implications for the field of medical imaging and deep learning. The ability to accurately segment and quantify small, complex structures within the human body using 3D neural networks paves the way for advancements in the diagnosis and monitoring of a wide range of medical conditions. As the field of computational medicine continues to evolve, innovations like this automated inner ear fluid analysis system will undoubtedly play a pivotal role in transforming the way healthcare providers approach complex diagnostic challenges.
Author credit: This article is based on research by Tae-Woong Yoo, Cha Dong Yeo, Minwoo Kim, Il-Seok Oh, Eun Jung Lee.
For More Related Articles Click Here