Climate change poses a pressing challenge, and understanding its impacts is crucial. In a comprehensive study, researchers evaluated the performance of 40 global climate models (GCMs) in simulating temperature and precipitation patterns across Iran, a country with diverse climatic conditions. By applying various evaluation criteria, the team identified top-performing models and investigated the effectiveness of bias correction methods. The findings offer valuable insights for improving climate projections and informing crucial decisions in areas like water resource management and sustainable agriculture. Climate change, Global climate models, Iran
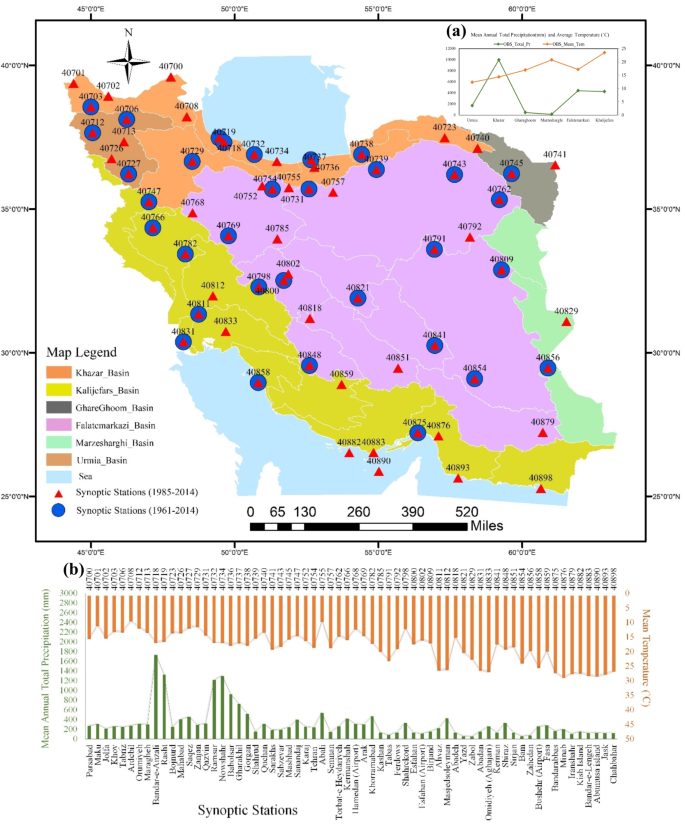
Tackling the Challenges of Climate Modeling
Climate change is a global phenomenon with far-reaching consequences, and governments worldwide are actively seeking ways to address its impacts. The Intergovernmental Panel on Climate Change (IPCC) plays a crucial role in compiling and publishing assessment reports based on extensive research, with the recent release of the Coupled Model Intercomparison Project Phase 6 (CMIP6) emphasizing the pivotal role of Global Climate Models (GCMs) in elucidating future climate patterns.
However, despite the significance of these models, considerable uncertainty remains in predicting and assessing the impacts of climate change on environmental and hydrological systems. Selecting suitable GCMs and addressing inherent biases in their outputs are essential steps in enhancing the reliability of climate projections.
Evaluating CMIP6 Models Across Iran
In this comprehensive study, researchers evaluated the performance of 40 CMIP6 GCMs in simulating temperature and precipitation patterns across Iran, a country with diverse climatic conditions. By applying a wide range of evaluation criteria, including deterministic, dichotomous, and probabilistic measures, as well as trend and nonstationarity analysis, the team identified the strengths and weaknesses of each model.
The results revealed that the performance of the models varied significantly across different regions and criteria. Some models, such as NorESM2-MM, AWI-ESM-1-1-LR, and MPI-ESM1-2-LR, consistently ranked among the top performers for precipitation, while others, like MPI-ESM1-2-LR, TaiESM1, INM-CM4-8, and IITM-ESM, excelled in simulating temperature.
Addressing Biases Through Bias Correction
To enhance the accuracy of the climate model outputs, the researchers applied two bias correction methods: linear scaling and quantile mapping. The quantile mapping approach demonstrated superior performance and reduced the disparity between the models, although it proved ineffective in addressing biases at stations with nonstationarity over time.
The study also explored the use of Bayesian Model Averaging (BMA) to aggregate the results of the climate models. The researchers found that the multi-model ensemble approach improved accuracy compared to individual models, resulting in a reduction in the differences between the minimum and maximum error values and narrowing the range of the correlation coefficient.
Informing Climate-Resilient Decisions
The findings of this study offer valuable insights for researchers, policymakers, and stakeholders interested in understanding and addressing the impacts of climate change. By identifying the top-performing climate models for Iran and evaluating the effectiveness of bias correction methods, the study provides a framework for improving the reliability of climate projections, particularly in regions with diverse climatic conditions.
This knowledge can inform critical decisions in areas such as agriculture’>sustainable agriculture, and the development of resilient infrastructure. By leveraging the insights from this research, stakeholders can better prepare for and mitigate the challenges posed by a changing climate, ensuring a more sustainable future for Iran and its people.
Author credit: This article is based on research by Narges Azad, Azadeh Ahmadi.
For More Related Articles Click Here