Researchers in the ENIGMA consortium have developed a powerful tool to compare scores across different tests of verbal memory and learning. By applying advanced statistical techniques to a massive dataset spanning over 10,000 individuals, they were able to create “crosswalks” that can translate scores between common assessment tools like the California Verbal Learning Test, Rey Auditory Verbal Learning Test, and Hopkins Verbal Learning Test. This breakthrough could help clinicians and scientists better understand subtle cognitive changes associated with conditions like traumatic brain injury, dementia, and mental health disorders. The findings demonstrate how big data and innovative data harmonization methods can address long-standing challenges in the behavioral sciences and pave the way for more robust, reproducible research. Cognitive assessment and big data are revolutionizing our understanding of the brain.
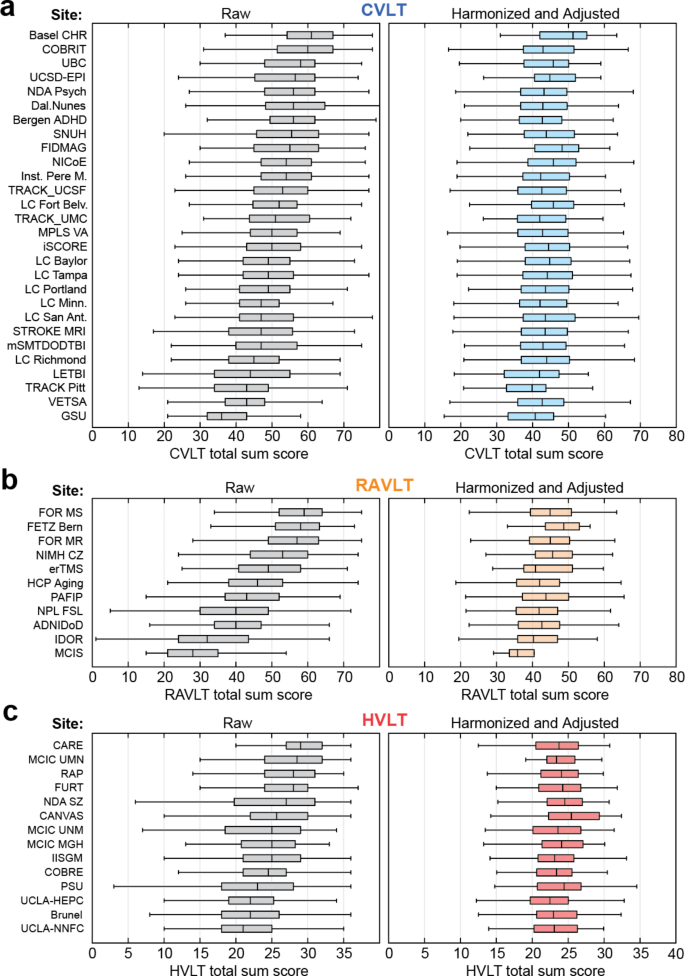
Overcoming Inconsistencies in Cognitive Measurement
Assessing cognitive functions like memory, attention, and language is crucial for tracking the impact of neurological and psychiatric conditions. However, clinicians and researchers have long grappled with the fact that there are numerous, non-equivalent tests available for measuring these abilities. For example, several distinct “auditory verbal learning tests” (AVLTs) exist to evaluate verbal memory and recall, each with their own unique features and scoring systems. These differences can make it difficult to compare results across studies or track changes in an individual over time.
The goal of the current study was to develop a way to accurately translate scores between common AVLT instruments. By assembling a massive dataset from 53 international research sites, the team was able to apply advanced statistical techniques to remove unwanted sources of variation while preserving meaningful effects related to age, education, and traumatic brain injury status.
Harmonizing Big Data to Unlock New Insights
Bringing together data from over 10,500 participants, the researchers first used a technique called ComBat-GAM to isolate and remove site-specific differences in test administration and scoring. This allowed them to directly compare performance across the CVLT, RAVLT, and HVLT – three of the most widely used AVLTs.
Next, the team employed item response theory to place all individuals on a common scale of “verbal learning ability.” This approach accounted for differences in item difficulty across the various tests, enabling the derivation of conversion tables that can translate raw scores between instruments. Validation on a small subset of participants who had taken multiple tests confirmed the accuracy of these crosswalks.
Implications for Clinical Practice and Research
The availability of these conversion tools has important implications. Clinicians can now more easily track changes in a patient’s memory and learning abilities over time, even if different assessment instruments are used. Researchers, in turn, can combine data from diverse studies to gain the statistical power needed to uncover subtle cognitive effects related to brain injury, dementia, and mental health conditions.
Beyond enabling direct comparisons, the findings also shed light on the relative difficulty of the AVLT instruments. The CVLT emerged as the most challenging test overall, while the HVLT was the easiest. This information can guide clinicians and researchers in selecting the appropriate tool for their needs.
Ultimately, this work demonstrates the power of big data and innovative data harmonization techniques to address longstanding challenges in the behavioral sciences. By bringing together a wealth of international data and applying cutting-edge analytical methods, the ENIGMA consortium has created a valuable resource that can transform how we understand and assess cognitive function.
Author credit: This article is based on research by Eamonn Kennedy, Shashank Vadlamani, Hannah M. Lindsey, Pui-Wa Lei, Mary Jo-Pugh, Paul M. Thompson, David F. Tate, Frank G. Hillary, Emily L. Dennis, Elisabeth A. Wilde, for the ENIGMA Clinical Endpoints Working Group.
For More Related Articles Click Here