Researchers have developed a new method to effectively detect and clean up abnormal data in big energy datasets. By using sparse self-coding techniques, the method can accurately identify and remove anomalies in energy data, ensuring higher data quality and reliability for decision-making. This is particularly important for industries like steel production, where abnormal data can negatively impact operations and lead to safety risks. The new approach outperforms existing methods in terms of error detection rate, missing detection rate, and overall cleaning efficiency. This breakthrough could help enterprises in the energy sector improve their data management and optimize their decision-making processes.
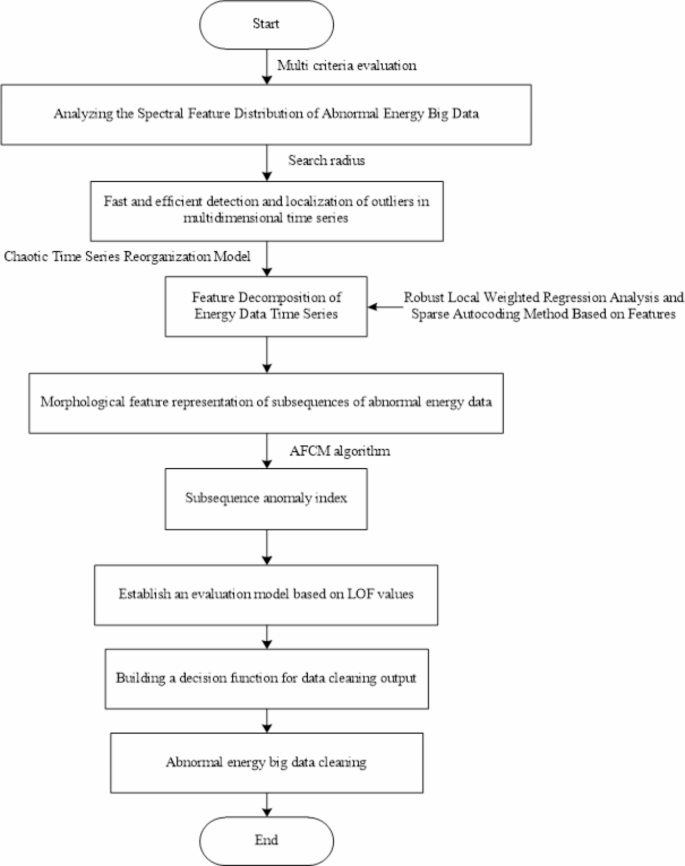
Tackling the Challenge of Abnormal Energy Data
In the age of big data, the steel industry and other energy-intensive sectors are generating vast amounts of information from their operations. This data, collected from sensors and monitoring equipment, can provide valuable insights to help optimize production, reduce costs, and enhance safety. However, the complex environment in energy production facilities often leads to sensor malfunctions, data transmission errors, and other issues, resulting in the presence of abnormal data points within these large datasets.
Abnormal energy data can have serious consequences, such as skewing the analysis of production trends, compromising the accuracy of predictive models, and even contributing to safety incidents. Traditional methods of identifying and removing these anomalies often rely on the experience of human dispatchers, which can be time-consuming and inefficient.
A Sparse Self-Coding Approach to Cleaning Energy Data
To address this challenge, researchers from the Electric Power Research Institute of State Grid Ningxia Electric Power Co., Ltd. have developed a new method for cleaning abnormal energy big data using sparse self-coding techniques. The key steps of their approach include:
1. Analyzing the spectral features of abnormal energy data to detect and localize anomalies in the multi-dimensional time series data.
2. Reconstructing the chaotic time series and decomposing the energy data features using robust local weighted regression and sparse self-coding.
3. Adaptively segmenting the original data sequence based on its periodicity and evaluating the morphological characteristics of the resulting subsequences.
4. Establishing an anomaly evaluation model using the Local Outlier Factor (LOF) to effectively identify and remove abnormal data points.
Outperforming Existing Methods
The researchers tested their method using real-world data from a steel production facility, specifically the flow rate of blast furnace gas. Compared to other existing approaches, the sparse self-coding method demonstrated superior performance:
– Error detection rate: 0.24% (vs. 5.48-11.65% for other methods)
– Missing detection rate: 0.27% (vs. 7.43-14.37% for other methods)
– Cleaning rate: 99.49% (vs. 70.15-72.97% for other methods)
– Detection and cleaning time: less than 2 seconds (significantly faster than other methods)
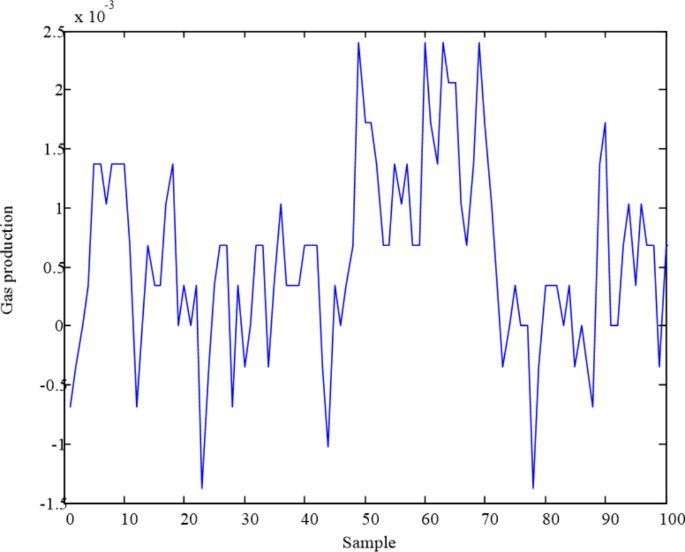
Improving Data Quality and Decision-Making
The ability to quickly and accurately clean up abnormal energy data is a significant breakthrough for industries like steel production. By providing reliable, high-quality data, the sparse self-coding method can help enterprises make more informed decisions, optimize their operations, and enhance safety. This research represents an important step forward in the field of energy data management and could have far-reaching implications for the energy sector as a whole.
Author credit: This article is based on research by Dongge Zhu, Shuang Zhang, Rui Ma, Wenni Kang, Jiangbo Sha.
For More Related Articles Click Here