Maintaining the health of pump station units (PSUs) is crucial for ensuring the reliable operation of water management systems. Researchers have developed a novel method that combines spatio-temporal data analysis and uncertainty quantification to provide real-time, comprehensive health assessments of PSUs. This approach not only helps identify potential issues early on but also supports the development of more effective maintenance strategies, ultimately extending the lifespan and efficiency of these critical infrastructure components. Water management and pump stations play a vital role in various industries, making this research highly relevant for practitioners and policymakers.
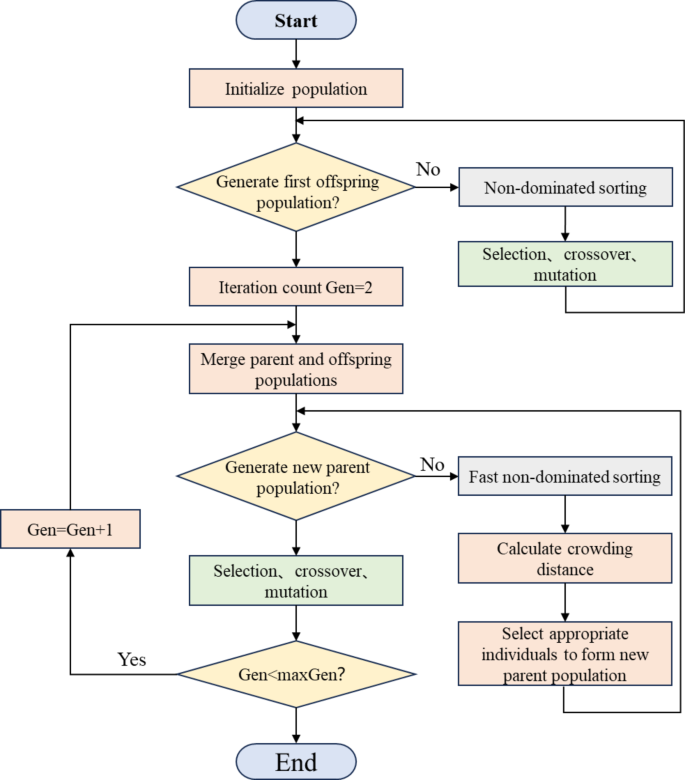
Tackling the Complexity of PSU Health Assessment
Pump station engineering is essential for applications like flood control and drainage, but it demands high operational safety standards. The machinery units, or PSUs, are the heart of these systems, and their health and efficiency are crucial for the stable functioning of water management infrastructure. However, traditional maintenance approaches, which rely on reactive repairs and scheduled preventive measures, can lead to both under-maintenance and over-maintenance, escalating safety risks and costs.
To address these challenges, researchers have been exploring data-driven health status assessment (HSA) methods, which leverage real-time monitoring data collected from sensors installed on the PSUs. These methods typically involve three steps: First, a health benchmark model (HBM) is constructed using the monitoring data collected during the unit’s healthy state. Next, the HBM is used to predict real-time monitoring signals. Finally, a health degradation index (HDI) is calculated based on the differences between the predicted and actual signals, providing insights into the equipment’s degradation process.
Enhancing HSA through Spatio-Temporal Fusion and Uncertainty Quantification
While existing data-driven HSA methods have shown promise, they often fall short in fully capturing the complex spatio-temporal dependencies and inherent uncertainties within the monitoring data. The research team, led by Panpan Qiu, Jianzhuo Yan, Hongxia Xu, and Yongchuan Yu, proposed an innovative approach to address these limitations.
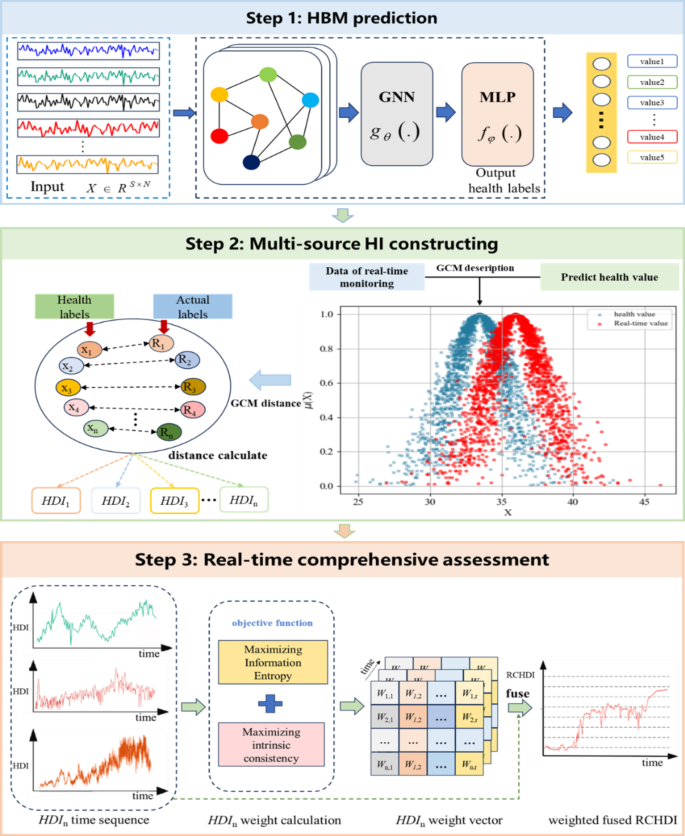
Their method starts by constructing an HBM using a Graph Neural Network (GNN) architecture that can effectively mine the spatio-temporal dependencies in the multi-dimensional monitoring data. The GNN-based HBM is capable of capturing cross-scale and cross-variable interactions, thereby improving its robustness to noise and better reflecting the complex relationships between different variables.
To further enhance the accuracy of the HSA, the researchers introduced a novel HDI construction method based on Mahalanobis distance (MD) and the Gaussian Cloud Model (GCM). This approach considers the uncertainty in signal changes, allowing for a more comprehensive and sensitive assessment of the unit’s health status.
Comprehensive Real-Time Health Evaluation
Finally, the researchers employed a dynamic multi-objective optimization algorithm, NSGA-II, to determine the optimal weights for integrating multiple HDIs into a Real-Time Comprehensive Health Degradation Index (RCHDI). This RCHDI provides a thorough and up-to-date assessment of the PSU’s overall health, enabling more informed maintenance decisions and better resource allocation.
The proposed method was validated through a case study using data from a pump station in China’s South-to-North Water Diversion Project. The results demonstrated that the method significantly improved the stability and smoothness of the health assessment curve, compared to other approaches. This enhanced stability and smoothness are crucial for early fault detection and timely maintenance, ultimately extending the operational efficiency and lifespan of the PSUs.
Implications and Future Directions
The research team’s innovative approach to PSU health assessment has important implications for water management and infrastructure maintenance. By integrating spatio-temporal data analysis and uncertainty quantification, this method offers a more reliable and comprehensive understanding of equipment health status, supporting the development of data-driven maintenance strategies and enhancing the overall resilience of critical water infrastructure.
As the researchers note, future work could explore the integration of high-fidelity data enhancement techniques to address the common challenge of poor data quality in field applications. Advancements in this area could further strengthen the accuracy and practical applicability of data-driven HSA methods, ultimately leading to more efficient and sustainable water management systems.
Author credit: This article is based on research by Panpan Qiu, Jianzhuo Yan, Hongxia Xu, Yongchuan Yu.
For More Related Articles Click Here