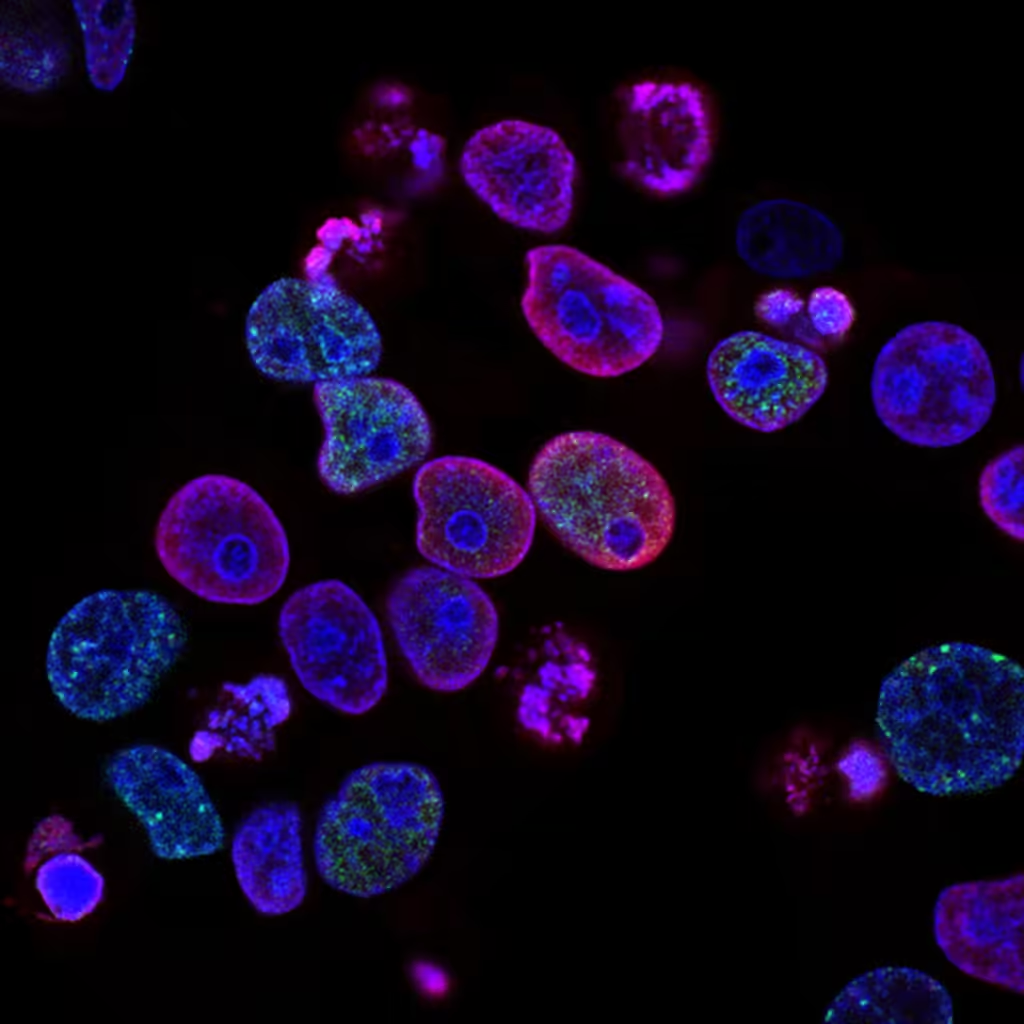
Cord blood is a valuable source of hematopoietic stem and progenitor cells (HSPCs) that can be used to treat a variety of blood, immune, and metabolic disorders. The number of CD34+ HSPCs in a cord blood unit is a critical factor in determining the success of a cord blood transplant. Researchers have developed a new machine learning-based approach to predict the proportion of CD34+ cells in cord blood units, which could help cord blood banks optimize the selection of units for clinical use. This work combines parametric and non-parametric statistical models to analyze maternal and neonatal factors that influence the CD34+ cell content, with the goal of improving transplantation outcomes for patients in need. Hematopoietic stem cells, Cord blood, Stem cell transplantation, Machine learning
Cord Blood: A Lifesaving Stem Cell Source
Cord blood, the blood that remains in the umbilical cord and placenta after childbirth, is a rich source of hematopoietic stem and progenitor cells (HSPCs). These cells have the ability to develop into various blood cell types, including red blood cells, white blood cells, and platelets. Cord blood transplantation has become a standard treatment for a wide range of hematological, oncological, metabolic, and immunodeficiency disorders, especially in pediatric patients.
One of the critical factors determining the success of a cord blood transplant is the number of CD34+ cells present in the cord blood unit. CD34+ cells are a specific type of HSPC that are essential for the reconstitution of the recipient’s blood and immune system after transplantation. Higher doses of CD34+ cells have been associated with improved engraftment and survival rates for patients undergoing cord blood transplants.
Predicting CD34+ Cell Content with Machine Learning
Researchers from the Cordlife Group Limited, a leading cord blood bank, have developed a novel approach to predict the proportion of CD34+ cells in cord blood units using a combination of parametric and non-parametric machine learning models. The goal of this study was to create a reliable and accurate predictive algorithm that could assist cord blood banks in selecting the optimal units for transplantation.
The researchers analyzed data from 802 processed cord blood units collected between 2020 and 2022. They gathered information on 24 different maternal and neonatal parameters, including factors such as baby gender, delivery type, cord blood volume and weight, and various cell counts. These variables were then used to train three different predictive models:
1. Multivariate linear regression: A parametric approach that generates a formula to predict the proportion of CD34+ cells.
2. Random forest: A non-parametric ensemble method that uses multiple decision trees to improve prediction accuracy.
3. Backpropagation neural network (BPNN): A non-parametric deep learning algorithm that can capture complex patterns in the data.
The researchers found that the BPNN model demonstrated the highest predictive power, with a median absolute deviation of 0.0689 and a forecast accuracy of 56.99% in predicting the CD34+ cell proportion. In contrast, the multivariate linear regression model had the lowest root-mean-square deviation of 0.0982, while the random forest model had a forecast accuracy of 36.79%.
Identifying Key Predictive Factors
The study also revealed several maternal and neonatal parameters that were particularly influential in predicting the CD34+ cell content of cord blood units. These included:
– Pre-processing leukocyte and lymphocyte counts: Higher counts of these cell types were associated with increased CD34+ cell yields.
– Post-processing TNC count: The total nucleated cell count after processing was a crucial indicator of graft potency.
– Time interval between collection and storage: Shorter intervals between cord blood collection and cryopreservation were linked to better CD34+ cell preservation.
By incorporating these key factors into the predictive models, the researchers were able to develop a powerful tool that can assist cord blood banks in selecting the most suitable units for transplantation. This could ultimately lead to improved engraftment and survival rates for patients receiving cord blood transplants.
Implications and Future Directions
The development of this machine learning-based predictive algorithm represents a significant advancement in the field of cord blood banking and transplantation. By accurately estimating the CD34+ cell content of cord blood units, cord blood banks can optimize the selection process and ensure that patients receive the best possible graft for their transplant.
This work also highlights the potential of machine learning to enhance various aspects of stem cell research and clinical applications. The ability to uncover complex relationships between maternal, neonatal, and processing factors, and their influence on HSPC content, could lead to a deeper understanding of the biology underlying cord blood stem cell potency.
Looking ahead, the researchers plan to further refine and validate their predictive models by incorporating additional variables and expanding the dataset. They also aim to explore the use of these algorithms in estimating the absolute CD34+ cell count, which is a crucial parameter for determining the appropriate cell dose for transplantation.
Overall, this study demonstrates the power of data-driven approaches in the field of cord blood banking and transplantation. By leveraging the predictive capabilities of machine learning, researchers can develop tools that improve the selection and utilization of this valuable stem cell resource, ultimately leading to better outcomes for patients in need of life-saving transplants.
Author credit: This article is based on research by Chi-Kwan Leung, Pengcheng Zhu, Ian Loke, Kin Fai Tang, Ho-Chuen Leung, Chin-Fung Yeung.
For More Related Articles Click Here