Learn more about this potentially path-breaking work that utilizes machine learning along with X-ray diffraction data to change the way we have been making and understanding new polymers, thus standing as a game-changer across multiple industries.
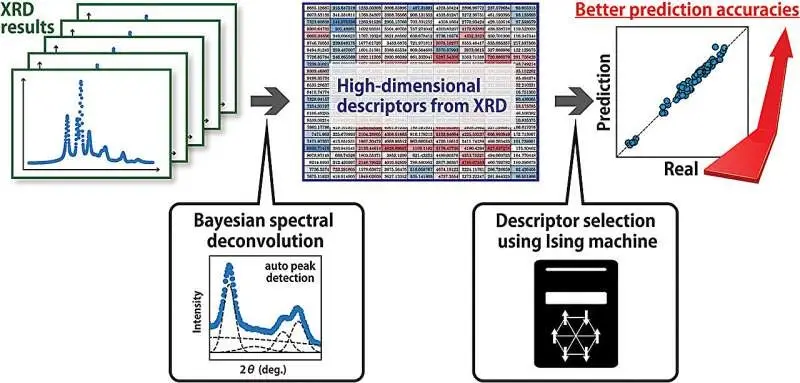
Revolutionizing Polymer Design
Polymers like polypropylene are the bedrock of modern technology, from computers to cars. Materials scientists need to know how these materials are going to perform under such and certain conditions.
It used to be, that you had to do physical tests which were expensive and destructive. However, a team of Japanese researchers has found a way to revolutionize the approach using machine learning to predict the mechanical properties of new polymers from X-ray diffraction data.
With the intricate polymer structure, researchers could instead identify certain characteristics in X-ray diffraction patterns and corollate those to material traits — such as rigidity, suppleness temperature. This non-invasive method is a clear improvement when compared to traditional testing techniques, as it opens doors for optimized and cost-saving polymer development.
Unlocking the Secrets of Polymer Structure
Thermoplastic crystalline polymers (such as polypropylene) have an especially complicated structure that is changed during production 1. For this reason, they turned to a technique called Bayesian spectral deconvolution — which is designed to help identify patterns in noisy data.
One of their datasets consisted of X-ray diffraction data from 15 different homo-polypropylenes at various temperatures, while the other was assembled to describe how injection moulding affected four different homo-polypropylene. To identify this relationship between the X-ray diffraction data and the mechanical properties of polymers, they closely examined the datasets to pinpoint the most critical descriptors.
Such an innovative methodology not only improves computational efficiency for the prediction of polymer behavior but also elucidates the structure-property relationship in these materials. In addition, the researchers think that their Bayesian spectral deconvolution method could be used to interpret data from other sources, such as X-ray photoelectron spectroscopy, to determine the properties of a wide variety of materials, including both organic and inorganic materials.
Conclusion
The discovery from the National Institute for Materials Science in Japan might change how we create and perceive new polymers. The research utilised machine learning to make predictions on the mechanical properties of polymers without causing them any damage, this allowed a better evaluation of the index of their polymeric nature using X-ray diffraction data while being customisable and cheaper than existing methods. It is a development that could have industry-wide implications, affecting everything from electronics to automotive, as materials scientists can now more efficiently and precisely design new polymer materials. The team’s Bayesian spectral deconvolution approach is a flexible tool to investigate the properties of an extensive variety of materials, so it is conceivable that this method could shine light into the future of materials science.