Researchers have developed a powerful machine learning model that can accurately predict the refractive index (RI) of inorganic compounds. This breakthrough has significant implications for the design and development of optical devices, from lasers to fiber optics. By leveraging the relationship between RI and a material’s band gap energy, as well as the properties of its constituent elements, the researchers have created a model that outperforms traditional empirical formulas. This innovative approach paves the way for faster and more cost-effective material discovery, ultimately driving advancements in fields like photonics and optoelectronics.
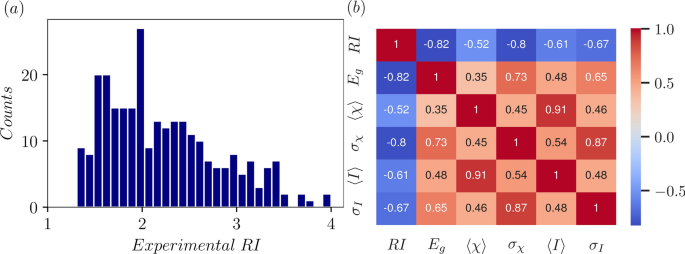
Predicting the Refractive Index of Inorganic Materials
The refractive index (RI) is a fundamental optical property that determines how light propagates through a material. Knowing the RI of a given inorganic compound is crucial for designing and manufacturing a wide range of optical devices, from optical fibers to optical switches. However, accurately predicting RI has been a long-standing challenge, as it depends on the complex electronic structure and atomic composition of the material.
Leveraging Machine Learning for Enhanced Predictive Power
In a groundbreaking study, researchers from K.N. Toosi University of Technology and the Institute for Research in Fundamental Sciences (IPM) in Iran have developed a machine learning (ML) model that can reliably predict the RI of a wide range of inorganic compounds. By analyzing a dataset of 272 inorganic materials, the researchers identified key predictors, such as the band gap energy and the atomic properties of the constituent elements, that strongly influence the RI.

Fig. 2
Outperforming Traditional Empirical Formulas
The researchers compared the performance of their ML model against well-known empirical formulas, such as the Moss and Ravindra equations, which have been commonly used to estimate RI. Their results showed that the ML model, particularly the Extremely Randomized Trees Regression (ERTR) method, outperformed these traditional approaches, providing more accurate predictions across a broader range of RI values.
Accelerating Material Discovery and Innovation
The ability to accurately predict the RI of inorganic materials using machine learning has significant implications for the scientific community. This approach can greatly accelerate the discovery and development of new functional materials, as it reduces the need for costly and time-consuming experimental measurements. By leveraging the power of ML, researchers can now explore a vast parameter space and identify promising candidate materials more efficiently, ultimately driving advancements in fields like optoelectronics and photonics.
Towards a Brighter Future with Inorganic Materials
The findings of this study highlight the immense potential of machine learning in materials science. By combining physicochemical insights with powerful computational techniques, researchers can unlock the secrets of inorganic materials and pave the way for a new era of innovation. As we continue to push the boundaries of what’s possible, the applications of this research could have far-reaching impacts on technologies that shape our daily lives, from high-speed communication to renewable energy solutions.
Author credit: This article is based on research by Elham Einabadi, Mahdi Mashkoori.
For More Related Articles Click Here